Articles with impact: insights into 10 years of research with machine learning.
JOURNAL OF APPLIED PHYSIOLOGY(2020)
摘要
Worldwide scientific output is growing faster and faster. Academics should not only publish much and fast, but also publish research with impact. The aim of this study is to use machine learning to investigate characteristics of articles that were published in the Journal of Applied Physiology between 2009 and 2018, and characterize high-impact articles. Article impact was assessed for 4,531 publications by three common impact metrics: the Altmetric Attention Scores, downloads, and citations. Additionally, a broad collection of (more than 200) characteristics was collected from the article's title, abstract, authors, keywords, publication, and article engagement. We constructed random forest (RF) regression models to predict article impact and articles with the highest impact (top-25% and top-10% for each impact metric), which were compared with a naive baseline method. RF models outperformed the baseline models when predicting the impact of unseen articles (P < 0.001 for each impact metric). Also, RF models predicted top-25% and top-10% high-impact articles with a high accuracy. Moreover, RF models revealed important article characteristics. Higher impact was observed for articles about exercise, training, performance and V̇o2max, reviews, human studies, articles from large collaborations, longer articles with many references and high engagement by scientists, practitioners and public or via news outlets and videos. Lower impact was shown for articles about respiratory physiology or sleep apnea, editorials, animal studies, and titles with a question mark or a reference to places or individuals. In summary, research impact can be predicted and better understood using a combination of article characteristics and machine learning.NEW & NOTEWORTHY Common measures of article impact are the Altmetric Attention Scores, number of downloads, and number of citations. To our knowledge, this is the first study that applies machine learning on a comprehensive collection of article characteristics to predict article attention scores, downloads, and citations. Using 10 years of research articles, we obtained accurate predictions of high-impact articles and discovered important article characteristics related to article impact.
更多查看译文
关键词
altmetrics, bibliometrics, machine learning, natural language processing, scientometrics
AI 理解论文
溯源树
样例
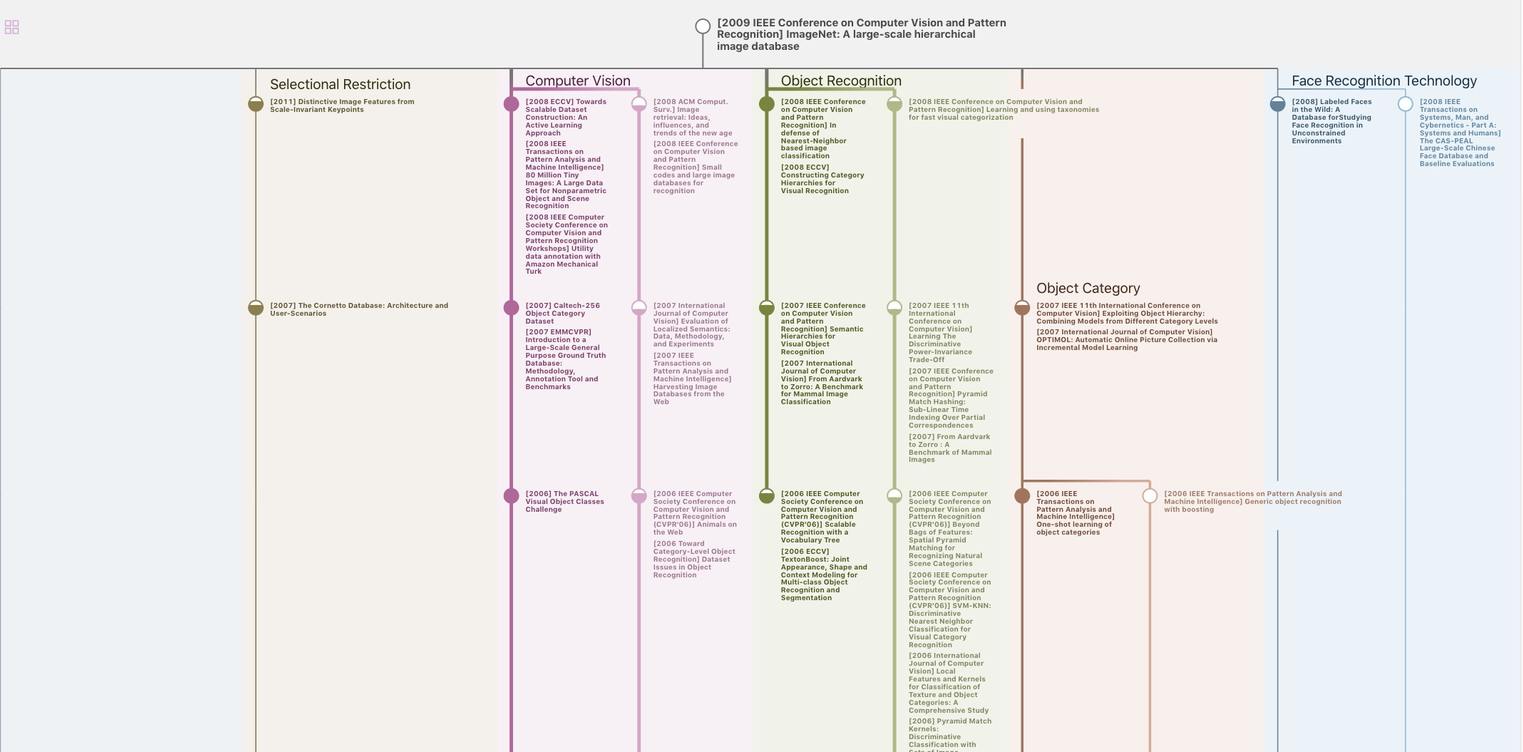
生成溯源树,研究论文发展脉络
Chat Paper
正在生成论文摘要