Heterogeneous Distributed SRAM Configuration for Energy-Efficient Deep CNN Accelerators
2020 18th IEEE International New Circuits and Systems Conference (NEWCAS)(2020)
摘要
Convolutional Neural Networks (CNNs) are often the first choice for visual recognition systems due to their high, even superhuman, recognition accuracy. The memory configuration of CNN accelerators highly impacts their area and energy efficiency, and employing on-chip memories such as SRAMs is unavoidable. SRAMs can reduce the number of energy-hungry DRAM accesses by storing a large amount of data locally. In this paper, we propose a new on-chip memory configuration, for a certain class of CNN accelerators that divides the memories into two groups. The first group consists of shallow but wide SRAMs into which parallel computational units accumulate intermediate results. The second group includes narrow but deep SRAMs shared between adjacent computational units to store then transfer final results to the external DRAM without interrupting the computation process. Implementation results show that the proposed configuration reduces the area by 21 % and improves the energy efficiency by 18% compared to designs which use an ordinary ping-pong structure for SRAM-DRAM data transfer.
更多查看译文
关键词
computational dataflow,convolutional neural networks (CNNs),hardware implementation,application-specific integrated circuit (ASIC)
AI 理解论文
溯源树
样例
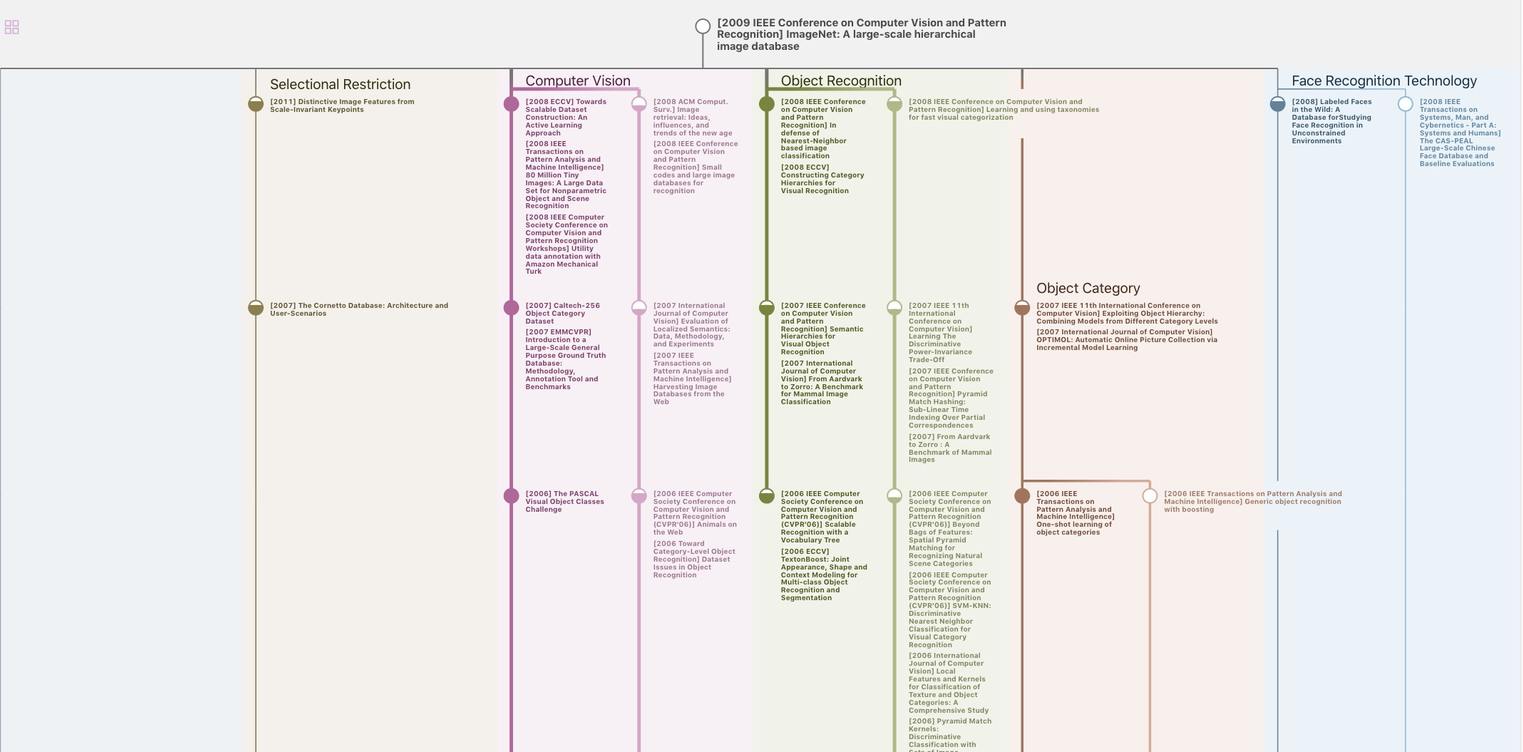
生成溯源树,研究论文发展脉络
Chat Paper
正在生成论文摘要