Decomposing Image Generation into Layout Prediction and Conditional Synthesis.
CVPR Workshops(2020)
摘要
Learning the distribution of multi-object scenes with Generative Adversarial Networks (GAN) is challenging. Guiding the learning using semantic intermediate representations, which are less complex than images, can be a solution. In this article, we investigate splitting the optimisation of generative adversarial networks into two parts, by first generating a semantic segmentation mask from noise and then translating that segmentation mask into an image. We performed experiments using images from the CityScapes dataset and compared our approach to Progressive Growing of GANs (PGGAN), which uses multiscale growing of networks to guide the learning. Using the lens of a segmentation algorithm to examine the structure of generated images, we find that our method achieves higher structural consistency in latent space interpolations and yields generations with better differentiation between distinct objects, while achieving the same image quality as PGGAN as judged by a user study and a standard GAN evaluation metric.
更多查看译文
关键词
image generation,layout prediction,conditional synthesis,multiobject scenes,generative adversarial networks,semantic intermediate representations,semantic segmentation mask,CityScapes dataset,PGGAN,segmentation algorithm,higher structural consistency,latent space interpolations,image quality,GAN evaluation metric,progressive growing of GANs
AI 理解论文
溯源树
样例
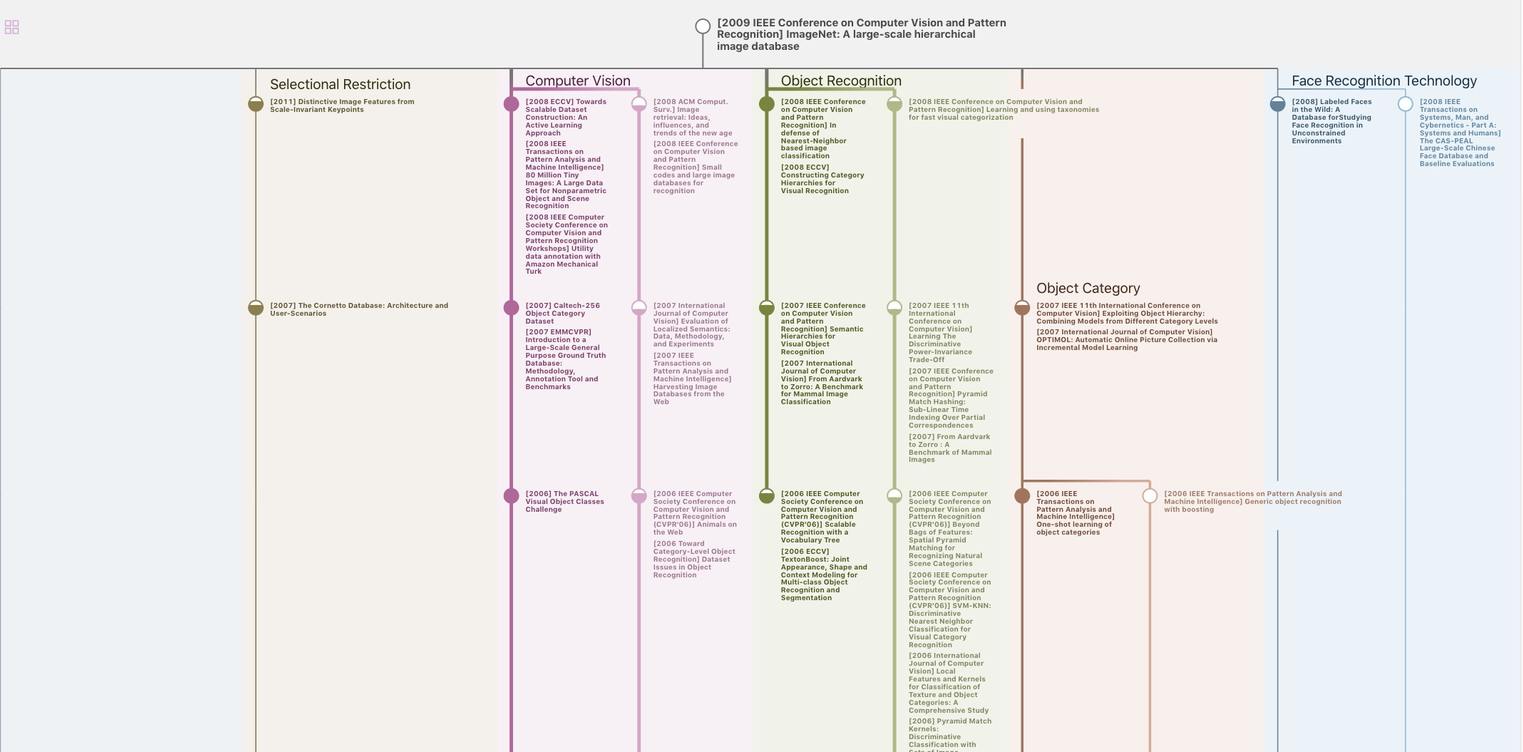
生成溯源树,研究论文发展脉络
Chat Paper
正在生成论文摘要