Multi-Granularity Tracking with Modularlized Components for Unsupervised Vehicles Anomaly Detection.
CVPR Workshops(2020)
摘要
Anomaly detection on road traffic is a fundamental computer vision task and plays a critical role in video structure analysis and urban traffic analysis. Although it has attracted intense attention in recent years, it remains a very challenging problem due to the complexity of the traffic scene, the dense chaos of traffic flow and the lack of fine-grained abnormal labeled data. In this paper, we propose a multi-granularity tracking approach with modularized components to analyze traffic anomaly detection. The modularized framework consists of a detection module, a background modeling module, a mask extraction module, and a multi-granularity tracking algorithm. Concretely, a box-level tracking branch and a pixel-level tracking branch is employed respectively to make abnormal predictions. Each tracking branch helps to capture abnormal abstractions at different granularity levels and provide rich and complementary information for the concept learning of abnormal behaviors. Finally, a novel fusion and backtracking optimization is further performed to refine the abnormal predictions. The experimental results reveal that our framework is superior in the Track4 test set of the NVIDIA AI CITY 2020 CHALLENGE, which ranked first in this competition, with a 98.5% F1-score and 4.8737 root mean square error.
更多查看译文
关键词
abnormal abstractions,granularity levels,abnormal behaviors,abnormal predictions,Track4 test set,NVIDIA AI CITY 2020 CHALLENGE,modularlized components,unsupervised vehicles anomaly detection,road traffic,fundamental computer vision task,video structure analysis,urban traffic analysis,intense attention,traffic scene,dense chaos,traffic flow,fine-grained abnormal labeled data,multigranularity tracking approach,modularized components,traffic anomaly detection,modularized framework,detection module,background modeling module,mask extraction module,multigranularity tracking algorithm,box-level tracking branch,pixel-level tracking branch
AI 理解论文
溯源树
样例
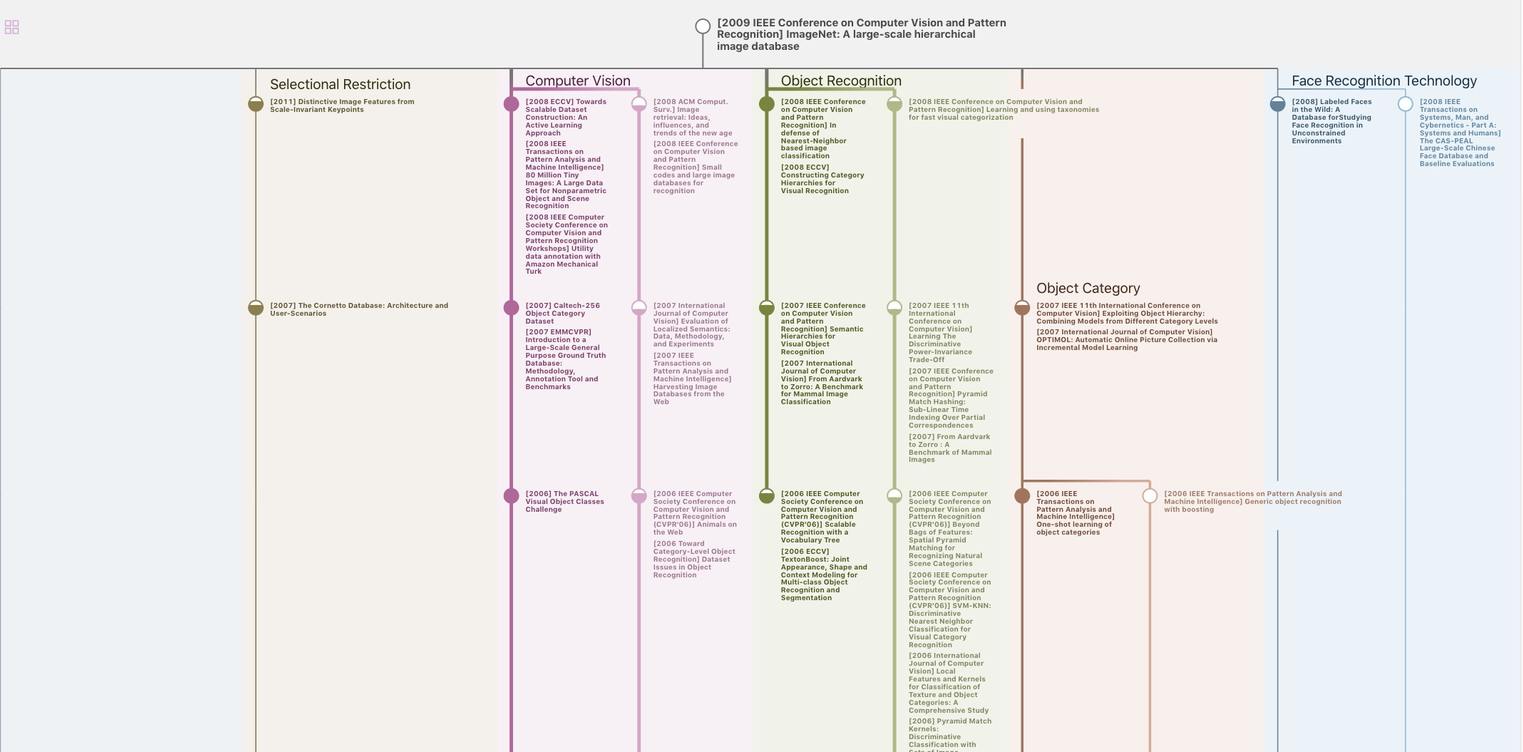
生成溯源树,研究论文发展脉络
Chat Paper
正在生成论文摘要