Low-rate Image Compression with Super-resolution Learning
2020 IEEE/CVF Conference on Computer Vision and Pattern Recognition Workshops (CVPRW)(2020)
摘要
In this paper, we propose an end-to-end learned image compression framework for low-rate scenarios. Based on variational autoencoder, our method features a pair of compact-resolution and super-resolution networks, a set of hyper and main codec networks, and a conditional context model. The learning process of this framework is facilitated with integrated non-local attention modules and phase congruency priors. Multiple models are obtained from training with different hyper-parameters, and are jointly used in the image-level model selection process for rate control, which ensures that the bit rate constraint of the CLIC challenge is satisfied. Experimental results demonstrate that the proposed method can achieve an averaged multi-scale structural similarity (MS-SSIM) score of 0.9648 with bit rate consumption of 0.1499 bits per pixel, which outperforms the BPG image coding method significantly.
更多查看译文
关键词
BPG image coding method,bit rate consumption,bit rate constraint,rate control,image-level model selection process,different hyper-parameters,phase congruency priors,integrated nonlocal attention modules,learning process,conditional context model,main codec networks,super-resolution networks,compact-resolution,variational autoencoder,low-rate scenarios,end-to-end learned image compression framework,super-resolution learning,low-rate image compression
AI 理解论文
溯源树
样例
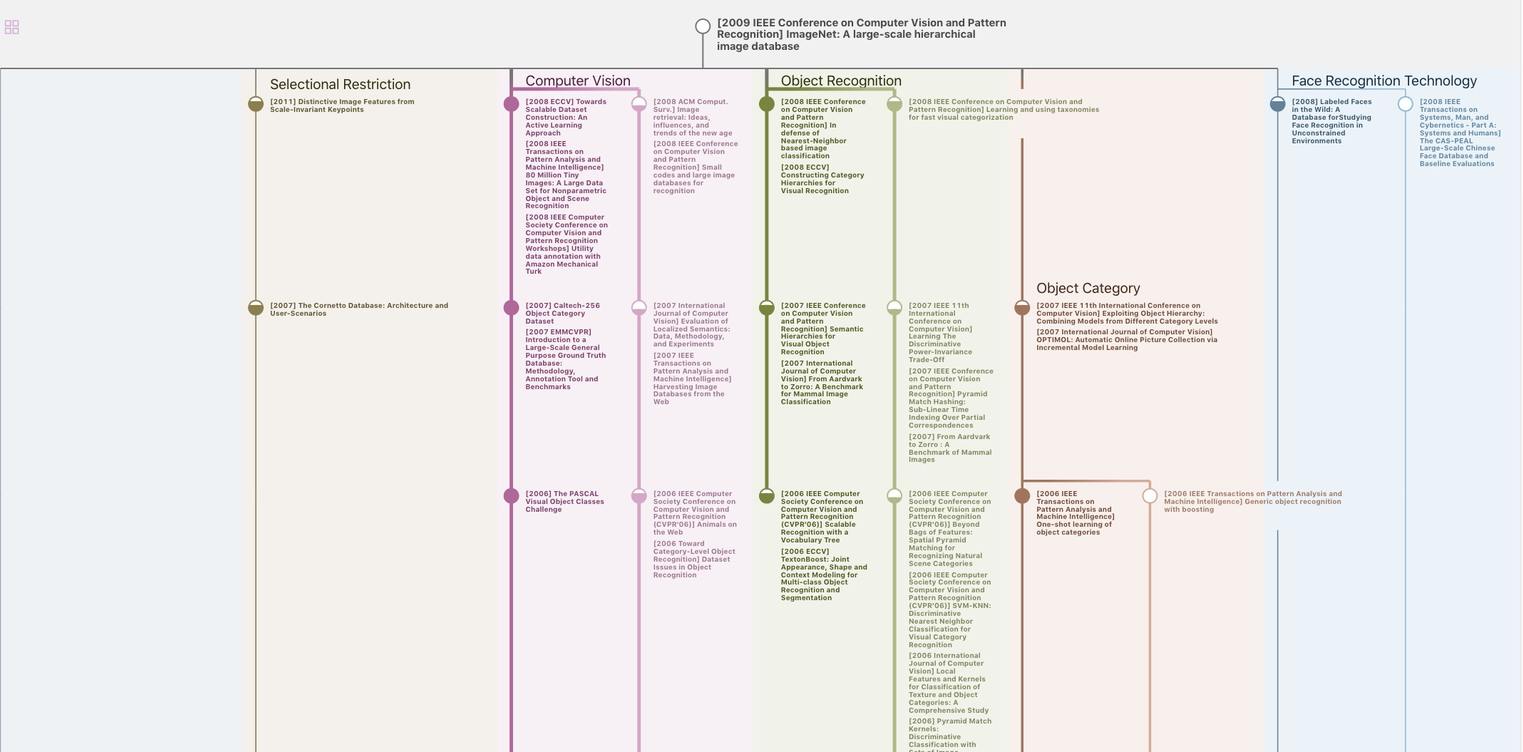
生成溯源树,研究论文发展脉络
Chat Paper
正在生成论文摘要