Knowledge Graphs Effectiveness In Neural Machine Translation Improvement
COMPUTER SCIENCE-AGH(2020)
摘要
Maintaining semantic relations between words during the translation process yields more accurate target-language output from Neural Machine Translation (NMT). Although difficult to achieve from training data alone, it is possible to leverage Knowledge Graphs (KGs) to retain source-language semantic relations in the corresponding target-language translation. The core idea is to use KG entity relations as embedding constraints to improve the mapping from source to target. This paper describes two embedding constraints, both of which employ Entity Linking (EL)-assigning a unique identity to entities-to associate words in training sentences with those in the KG: (1) a monolingual embedding constraint that supports an enhanced semantic representation of the source words through access to relations between entities in a KG; and (2) a bilingual embedding - constraint that forces entity relations in the source-language to be carried over to the corresponding entities in the target-language translation. The method is evaluated for English-Spanish translation exploiting Freebase as a source of knowledge. Our experimental results demonstrate that exploiting KG information not only decreases the number of unknown words in the translation but also improves translation quality.
更多查看译文
关键词
natural language processing, neural machine translation, knowledge graph representation
AI 理解论文
溯源树
样例
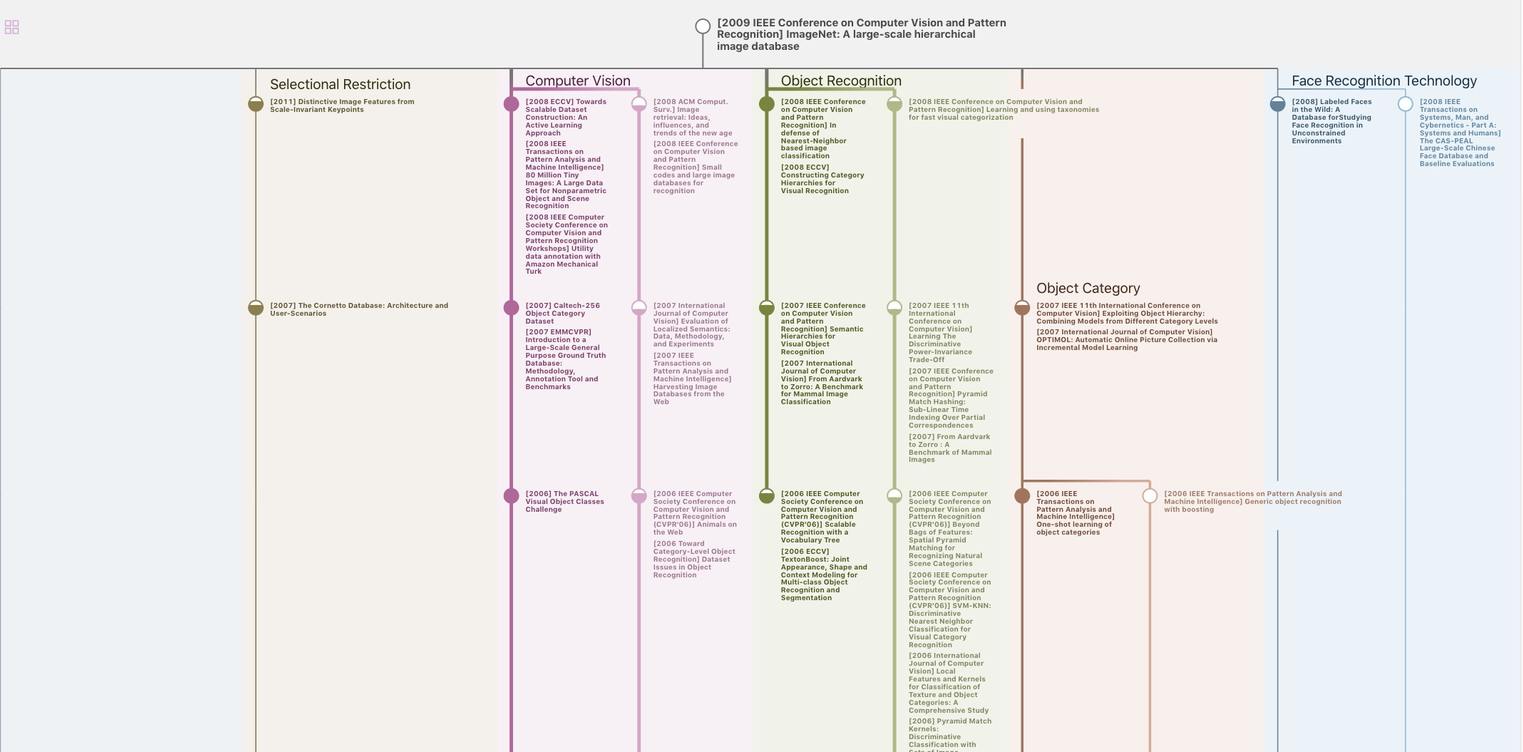
生成溯源树,研究论文发展脉络
Chat Paper
正在生成论文摘要