Class-Oriented Poisoning Attack
arXiv (Cornell University)(2021)
摘要
Poisoning attacks on machine learning systems compromise the model performance by deliberately injecting malicious samples in the training dataset to influence the training process. Prior works focus on either availability attacks (i.e., lowering the overall model accuracy) or integrity attacks (i.e., enabling specific instance based backdoor). In this paper, we advance the adversarial objectives of the availability attacks to a per-class basis, which we refer to as class-oriented poisoning attacks. We demonstrate that the proposed attack is capable of forcing the corrupted model to predict in two specific ways: (i) classify unseen new images to a targeted "supplanter" class, and (ii) misclassify images from a "victim" class while maintaining the classification accuracy on other non-victim classes. To maximize the adversarial effect, we propose a gradient-based framework that manipulates the logits to retain/eliminate the desired/undesired feature information in the generated poisoning images. Using newly defined metrics at the class level, we illustrate the effectiveness of the proposed class-oriented poisoning attacks on various models (e.g., LeNet-5, Vgg-9, and ResNet-50) over a wide range of datasets (e.g., MNIST, CIFAR-10, and ImageNet-ILSVRC2012).
更多查看译文
关键词
poisoning attacks,class-oriented
AI 理解论文
溯源树
样例
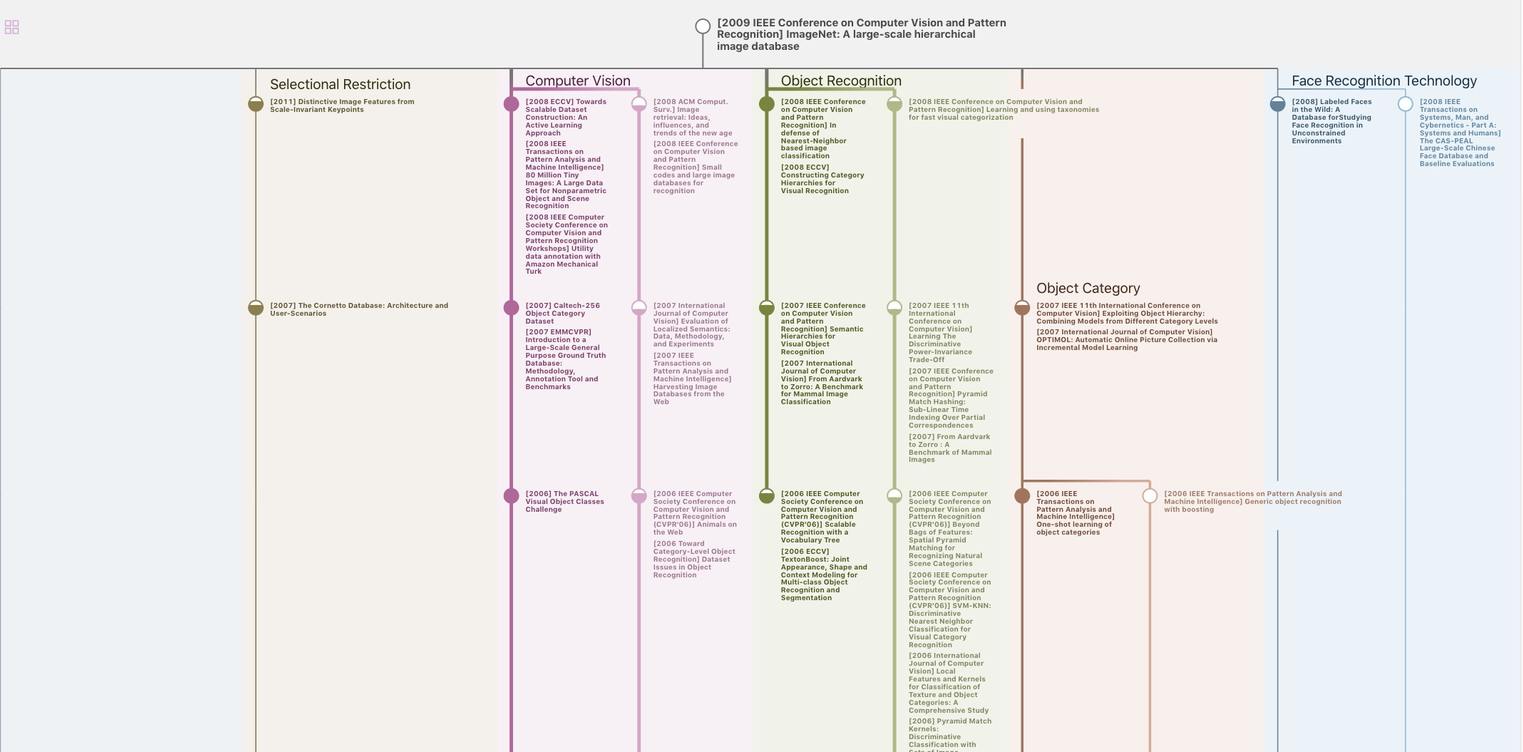
生成溯源树,研究论文发展脉络
Chat Paper
正在生成论文摘要