Regression modelling with I-priors
arxiv(2020)
摘要
We introduce a methodology with the aim of providing a unifying framework for estimating a variety of regression methods and models, including multilevel, varying coefficient, longitudinal models, and models with functional covariates and responses. As we show, a natural space for the regression functions pertaining to such models is the reproducing kernel Krein space (RKKS). We introduce the I-prior over the RKKS of the model, defined as the maximizer of entropy subject to a suitable constraint involving the Fisher information on the regression function. The I-prior is Gaussian with covariance kernel proportional to the Fisher information on the regression function, and the regression function is estimated by its posterior distribution under the I-prior. The I-prior has the intuitively appealing property that the more information is available on a linear functional of the regression function, the larger the prior variance, and the smaller the influence of the prior mean on the posterior distribution. The methodology we introduce has some advantages compared to commonly used methods in terms of ease of estimation and model comparison. Firstly, a single methodology can be used for a variety of models, for which previously different methods were used. Secondly, an EM algorithm with a simple E and M step for estimating the scale parameter of each covariate is available, facilitating estimation for complex models. Thirdly, we propose a novel parsimonious model formulation, requiring a single scale parameter for each covariate and no further parameters for interaction effects, allowing a semi-Bayes approach to the selection of interaction effects. An R-package implementing our methodology is available (Jamil, 2019).
更多查看译文
AI 理解论文
溯源树
样例
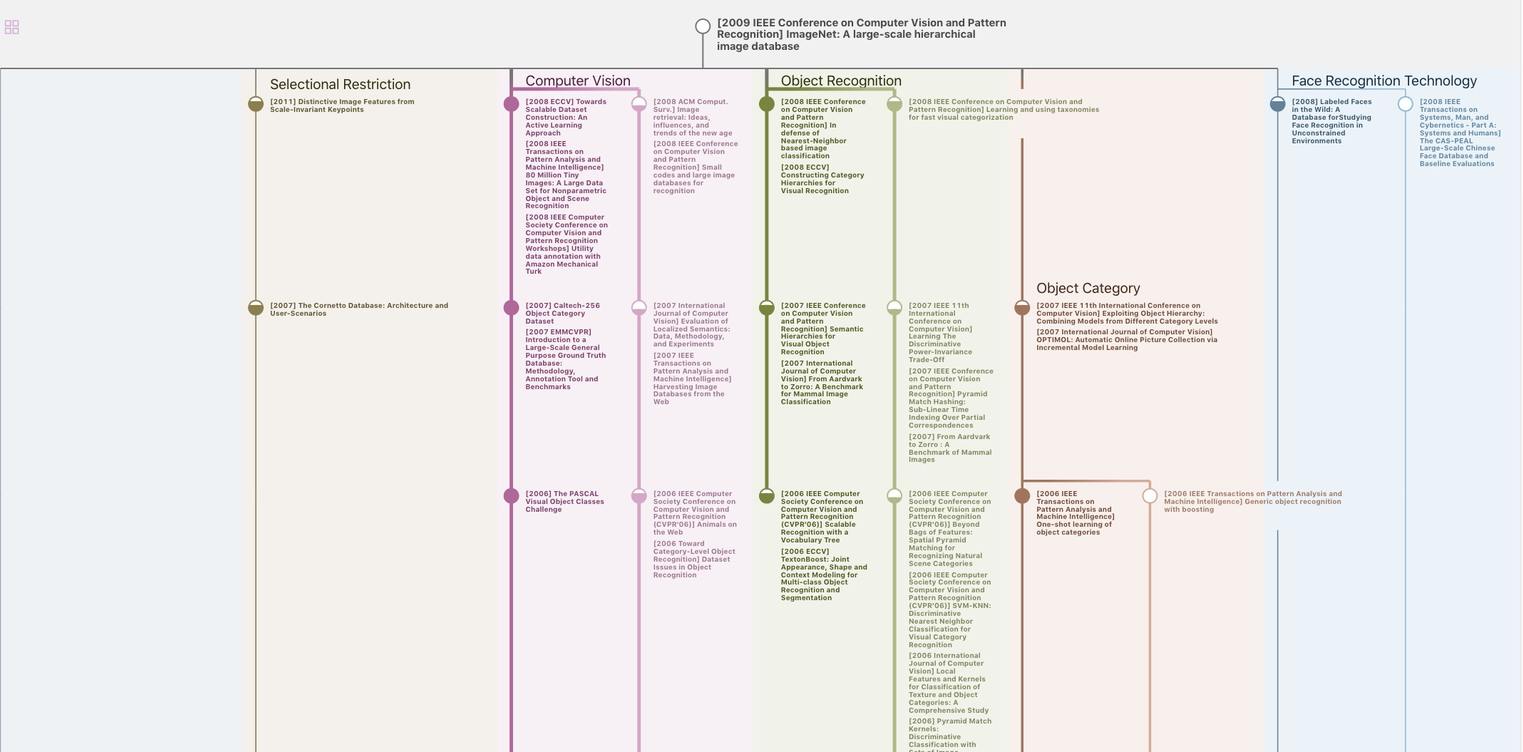
生成溯源树,研究论文发展脉络
Chat Paper
正在生成论文摘要