$K$ K -Shot Contrastive Learning of Visual Features With Multiple Instance Augmentations
IEEE Transactions on Pattern Analysis and Machine Intelligence(2022)
摘要
In this paper, we propose the
$K$
-Shot Contrastive Learning (KSCL) of visual features by applying multiple augmentations to investigate the sample variations within individual instances. It aims to combine the advantages of
inter-instance discrimination
by learning discriminative features to distinguish between different instances, as well as
intra-instance variations
by matching queries against the variants of augmented samples over instances. Particularly, for each instance, it constructs an instance subspace to model the configuration of how the significant factors of variations in
$K$
-shot augmentations can be combined to form the variants of augmentations. Given a query, the most relevant variant of instances is then retrieved by projecting the query onto their subspaces to predict the positive instance class. This generalizes the existing contrastive learning that can be viewed as a special one-shot case. An eigenvalue decomposition is performed to configure instance subspaces, and the embedding network can be trained end-to-end through the differentiable subspace configuration. Experiment results demonstrate the proposed
$K$
-shot contrastive learning achieves superior performances to the state-of-the-art unsupervised methods.
更多查看译文
关键词
Unsupervised learning,self-supervised learning,contrastive learning
AI 理解论文
溯源树
样例
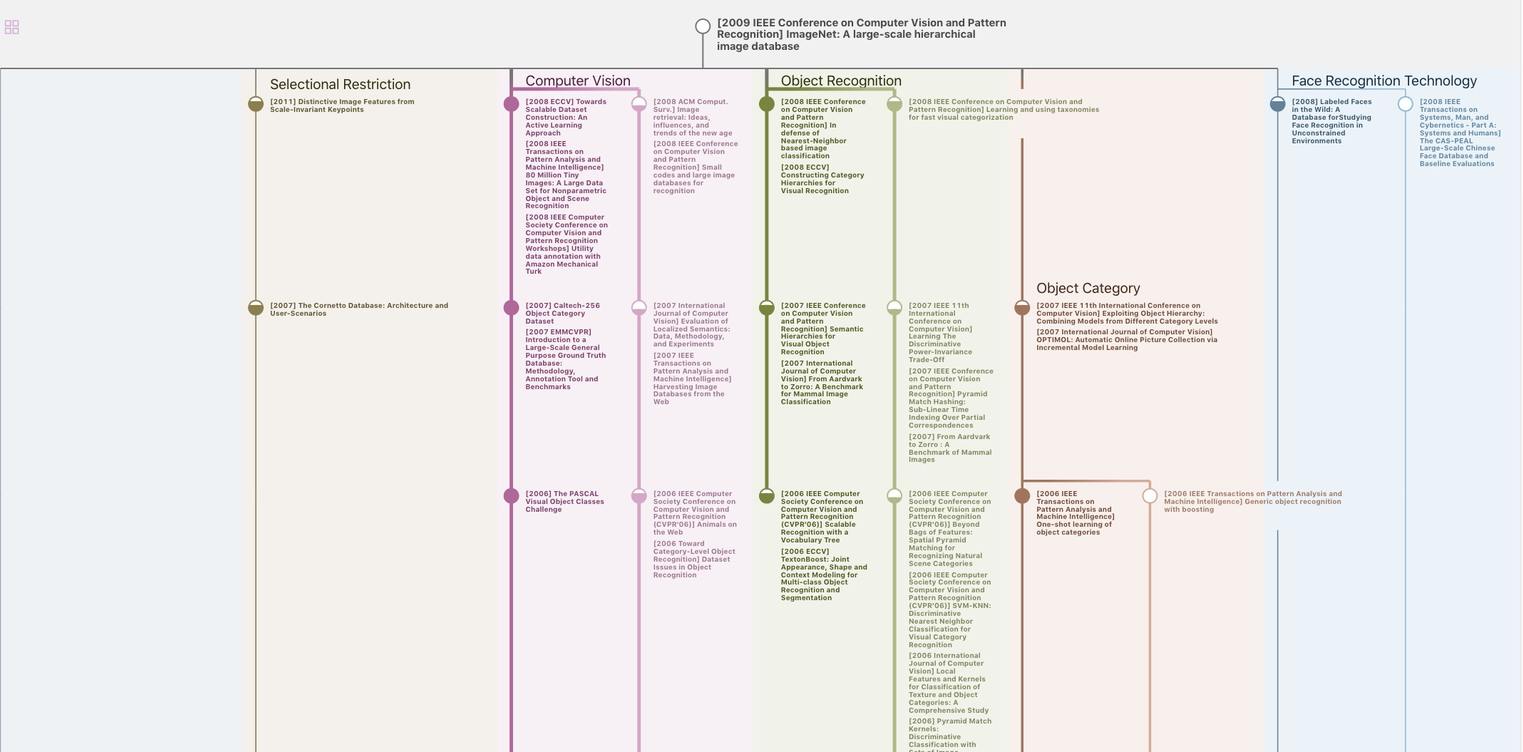
生成溯源树,研究论文发展脉络
Chat Paper
正在生成论文摘要