Frequency Estimation in Data Streams: Learning the Optimal Hashing Scheme
IEEE Transactions on Knowledge and Data Engineering(2023)
摘要
We present a novel approach for the problem of frequency estimation in data streams that is based on optimization and machine learning. Contrary to state-of-the-art streaming frequency estimation algorithms, which heavily rely on random hashing to maintain the frequency distribution of the data steam using limited storage, the proposed approach exploits an observed stream prefix to near-optimally hash elements and compress the target frequency distribution. We develop an exact mixed-integer linear optimization formulation, which enables us to compute optimal or near-optimal hashing schemes for elements seen in the observed stream prefix; then, we use machine learning to hash unseen elements. Further, we develop an efficient block coordinate descent algorithm, which, as we empirically show, produces high quality solutions, and, in a special case, we are able to solve the proposed formulation exactly in linear time using dynamic programming. We empirically evaluate the proposed approach both on synthetic datasets and on real-world search query data. We show that the proposed approach outperforms existing approaches by one to two orders of magnitude in terms of its average (per element) estimation error and by 45-90 percent in terms of its expected magnitude of estimation error.
更多查看译文
关键词
Data streams,streaming frequency estimation,learning to hash,optimal hashing scheme
AI 理解论文
溯源树
样例
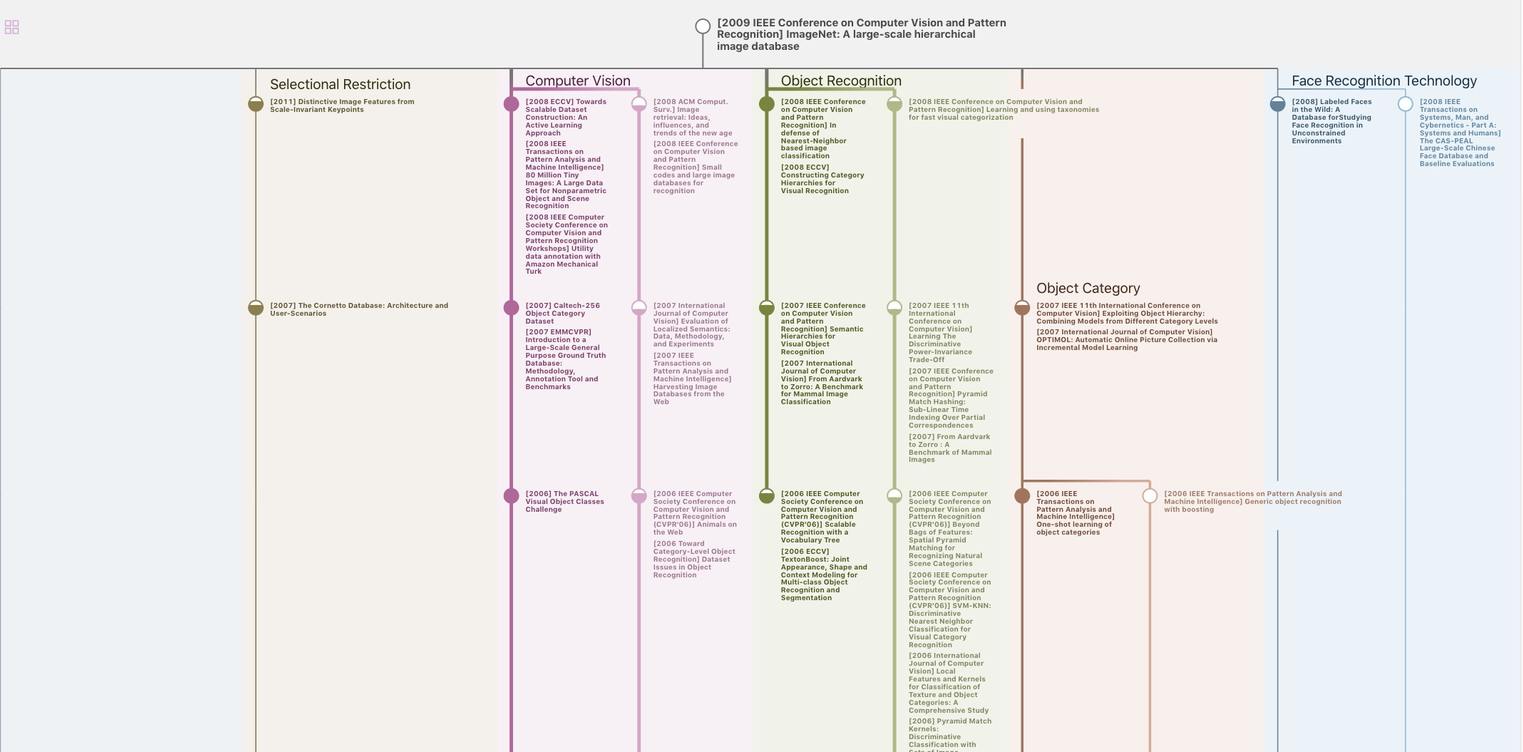
生成溯源树,研究论文发展脉络
Chat Paper
正在生成论文摘要