Scaling of Union of Intersections for Inference of Granger Causal Networks from Observational Data
2020 IEEE International Parallel and Distributed Processing Symposium (IPDPS)(2020)
摘要
The development of advanced recording and measurement devices in scientific fields is producing high-dimensional time series data. Vector autoregressive (VAR) models are well suited for inferring Granger-causal networks from high dimensional time series data sets, but accurate inference at scale remains a central challenge. We have recently introduced a flexible and scalable statistical machine learning framework, Union of Intersections (UoI), which enables low false-positive and low false-negative feature selection along with low bias and low variance estimation, enhancing interpretation and predictive accuracy. In this paper, we scale the UoI framework for VAR models (algorithm UoI
V AR
) to infer network connectivity from large time series data sets (TBs). To achieve this, we optimize distributed convex optimization and introduce novel strategies for improved data read and data distribution times. We study the strong and weak scaling of the algorithm on a Xeon-phi based supercomputer (100,000 cores). These advances enable us to estimate the largest VAR model as known (1000 nodes, corresponding to 1M parameters) and apply it to large time series data from neurophysiology (192 neurons) and finance (470 companies).
更多查看译文
AI 理解论文
溯源树
样例
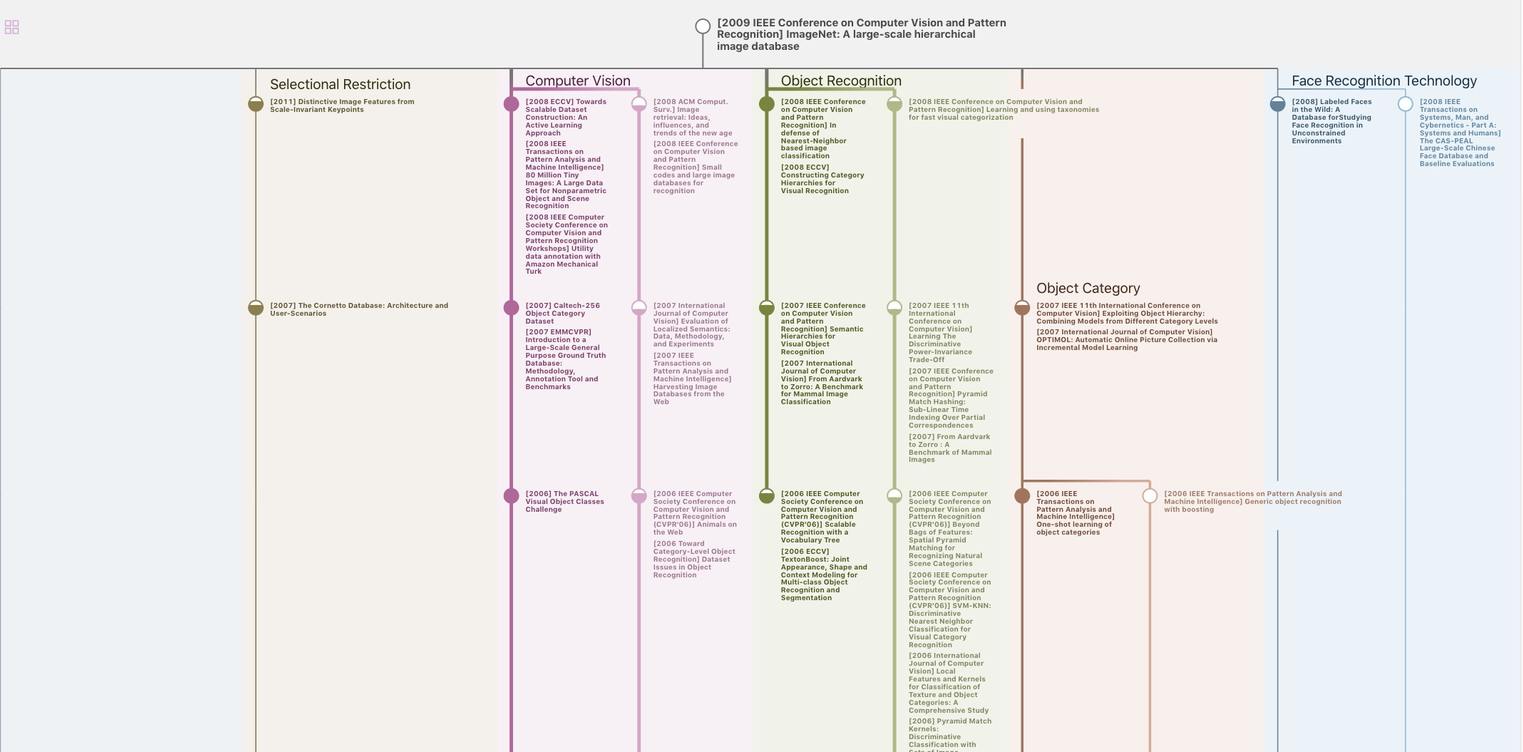
生成溯源树,研究论文发展脉络
Chat Paper
正在生成论文摘要