Benchmarking the Performance and Energy Efficiency of AI Accelerators for AI Training
2020 20th IEEE/ACM International Symposium on Cluster, Cloud and Internet Computing (CCGRID)(2020)
摘要
Deep learning has become widely used in complex AI applications. Yet, training a deep neural network (DNNs) model requires a considerable amount of calculations, long running time, and much energy. Nowadays, many-core AI accelerators (e.g., GPUs and TPUs) are designed to improve the performance of AI training. However, processors from different vendors perform dissimilarly in terms of performance and energy consumption. To investigate the differences among several popular off-the-shelf processors (i.e., Intel CPU, NVIDIA GPU, AMD GPU, and Google TPU) in training DNNs, we carry out a comprehensive empirical study on the performance and energy efficiency of these processors
1
by benchmarking a representative set of deep learning workloads, including computation-intensive operations, classical convolutional neural networks (CNNs), recurrent neural networks (LSTM), Deep Speech 2, and Transformer. Different from the existing end-to-end benchmarks which only present the training time, We try to investigate the impact of hardware, vendor's software library, and deep learning framework on the performance and energy consumption of AI training. Our evaluation methods and results not only provide an informative guide for end users to select proper AI accelerators, but also expose some opportunities for the hardware vendors to improve their software library.
更多查看译文
关键词
AI Accelerator,Deep Learning,CPU,GPU,TPU,Computation-intensive Operations,Convolution Neural Networks,Recurrent Neural Networks,Transformer,Deep Speech 2
AI 理解论文
溯源树
样例
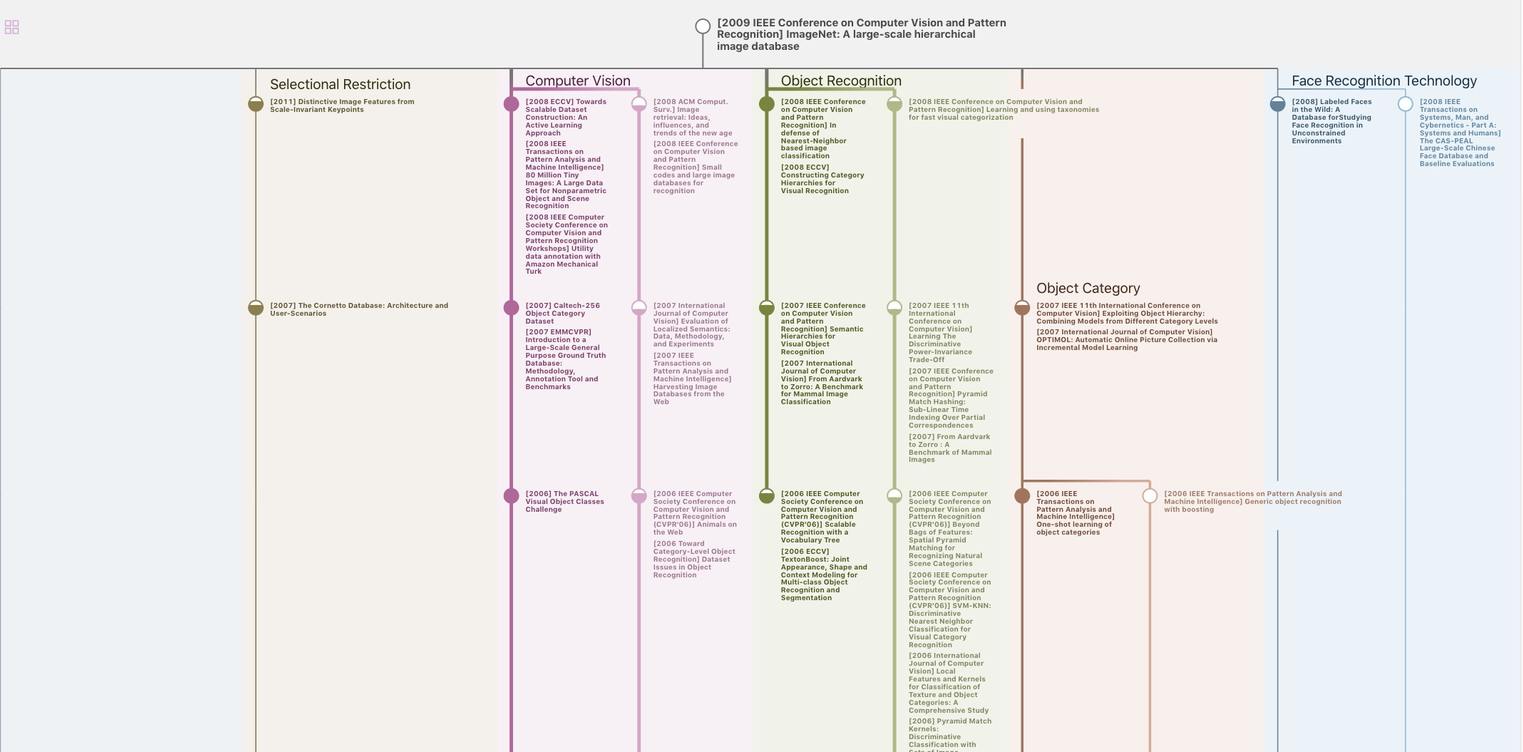
生成溯源树,研究论文发展脉络
Chat Paper
正在生成论文摘要