Batch Learning from Bandit Feedback through Bias Corrected Reward Imputation
semanticscholar(2019)
摘要
The problem of batch learning from logged contextual bandit feedback (BLBF) is ubiquitous in recommender systems, search, and online retail. Most previous methods for BLBF have followed a “Model the Bias” approach, estimating the expected reward of a policy using inverse propensity score (IPS) weighting. While unbiased, controlling the variance can be challenging. In contrast, we take a “Model the World” approach using the Direct Method (DM), where we learn a rewardregression model and derive a policy from the estimated rewards. While this approach has not been competitive with IPS weighting for mismatched models due to its bias, we show how directly minimizing the bias of the reward-regression model can lead to highly effective policy learning. In particular, we propose Bias Corrected Reward Imputation (BCRI) and formulate the policy learning problem as bi-level optimization, where the upper level maximizes the DM estimate and the lower level fits a weighted reward-regression. We empirically characterize the effectiveness of BCRI compared to conventional reward-regression baselines and an IPS-based method.
更多查看译文
AI 理解论文
溯源树
样例
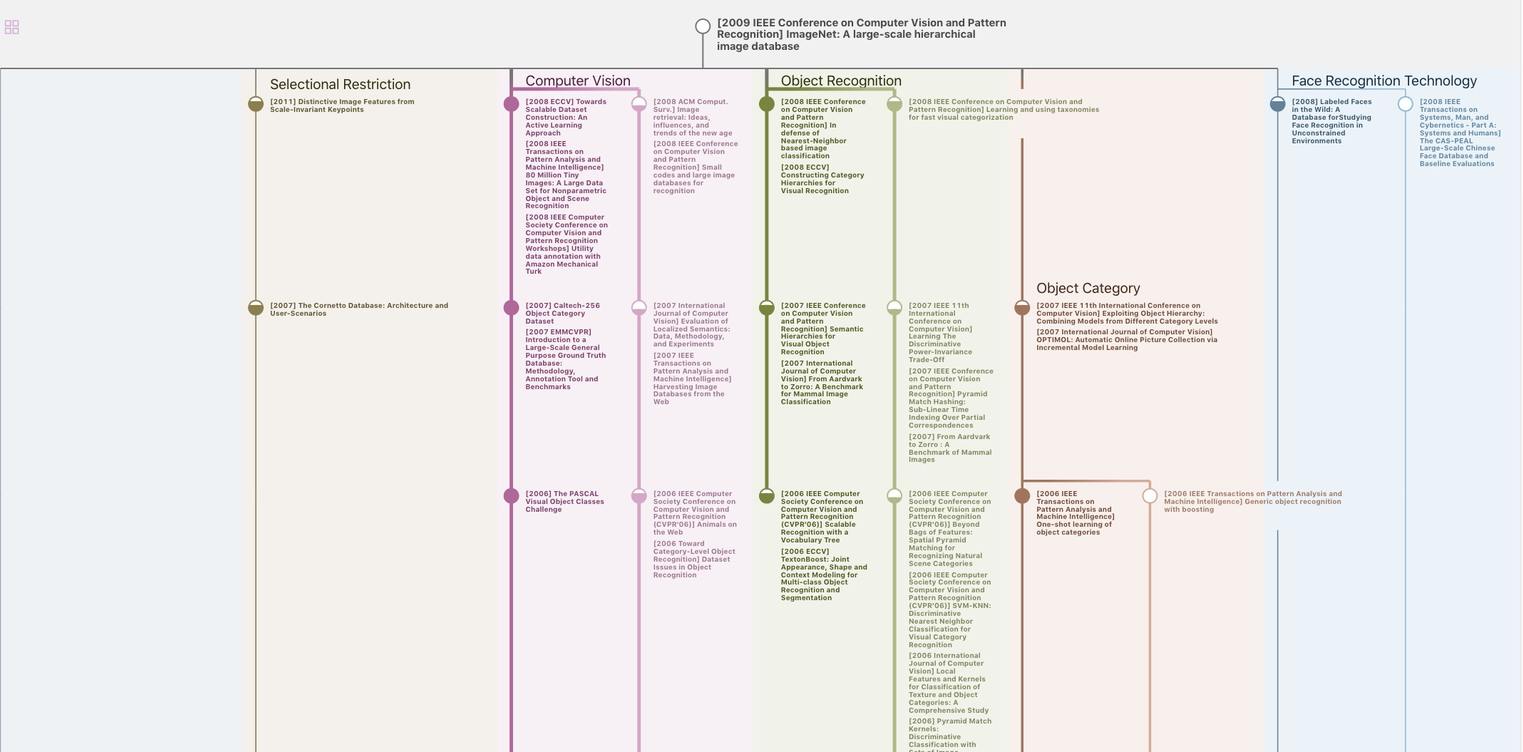
生成溯源树,研究论文发展脉络
Chat Paper
正在生成论文摘要