A Probabilistic Graphical Model for Identifying Text Patterns
semanticscholar(2011)
摘要
We describe a generic probabilistic graphical model that works for recognizing three types of text patterns in a sentence: noun phrases; the meaning of an ambiguous word; and semantic arguments of a verb. We model dependency in the input symbol sequence rather than the output class identification sequence as in CRF’s, HMM’s, and MEMMs. This enables the determination of the optimal class for each input symbol to be obtained independent of the optimal class for every other input symbol. The algorithm needs no dynamic programming. Experiments conducted on standard data sets show results just as good or better that the competing techniques. For instance, our method achieves an average precision of 97.7% and an average recall of 98.8% for recognizing noun phrases on WSJ data from Penn Treebank; an average accuracy of 81.12% for recognizing the six senses of the word ′line′; an average precision of 92.96% and an average of recall of 94.94% for classifying semantic argument boundaries of a verb of a sentence on WSJ data from Penn Treebank and PropBank.
更多查看译文
AI 理解论文
溯源树
样例
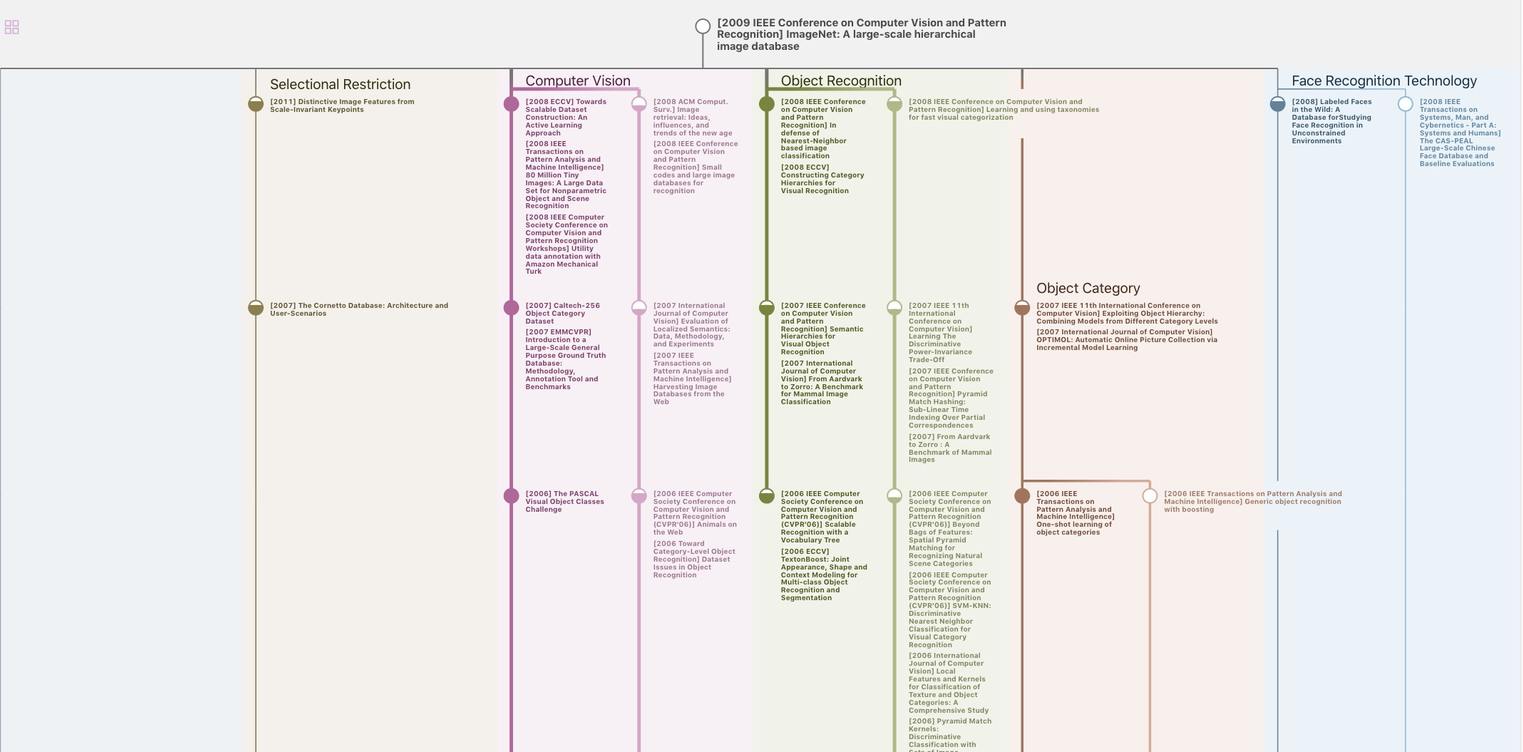
生成溯源树,研究论文发展脉络
Chat Paper
正在生成论文摘要