Learning Network Legos by Infinite Non-Negative Matrix Factorization
semanticscholar(2010)
摘要
Active molecular interactions change over time or under different conditions, leading to various phenotypes. Modeling the dynamics of such interaction networks is a critical problem in systems biology and can help us discover unknown relationships between molecular interactions to phenotypes of interest. In this paper, we propose a new Bayesian nonparametric matrix factorization model to address this problem. In this model, the molecular interaction networks perturbed at different times or under different conditions are represented by a matrix. We factorize this matrix into two latent matrices, one representing building blocks (which we call network legos) of these timeor conditionspecific response networks and the other (the loading matrix) telling us how to combine the legos to generate the response networks. The loading matrix is given a Bayesian nonparametric prior based on the Indian Buffet Process. While this prior allows the loading matrix to have infinite number of columns theoretically, in practice, this prior enables us to learn the number of latent building blocks automatically from the data. For the lego matrix, we use a truncated Gaussian prior, regularizing the model to prevent overfitting. This prior also effectively imposes nonnegativity constraints on the legos, such that the estimated legos can be easily interpreted as building blocks whose additive combinations compose the given response networks. Due to the nonparametric prior and the non-negativity constraints, we call our model Infinite Non-negative Matrix Factorization (INMF). We apply INMF to both synthetic network data and human functional genomic data and compare with alternative approaches. On synthetic response network data with different levels of noise, size, and density, INMF consistently outperforms classical nonnegative matrix factorization and the nonparametric Bayesian linear-Gaussian approach in terms of accuracy of estimating true network legos. When applied to data for environmental stresses on human cells, INMF outperforms our earlier Boolean approach in terms of robustly handling noise in the data and avoiding overfitting. On data for 18 human cancers, INMF discovers network legos that serve as “information backbones” and represent processes commonly perturbed in many cancers as well as network legos that capture aspects of specific subsets of cancers.
更多查看译文
AI 理解论文
溯源树
样例
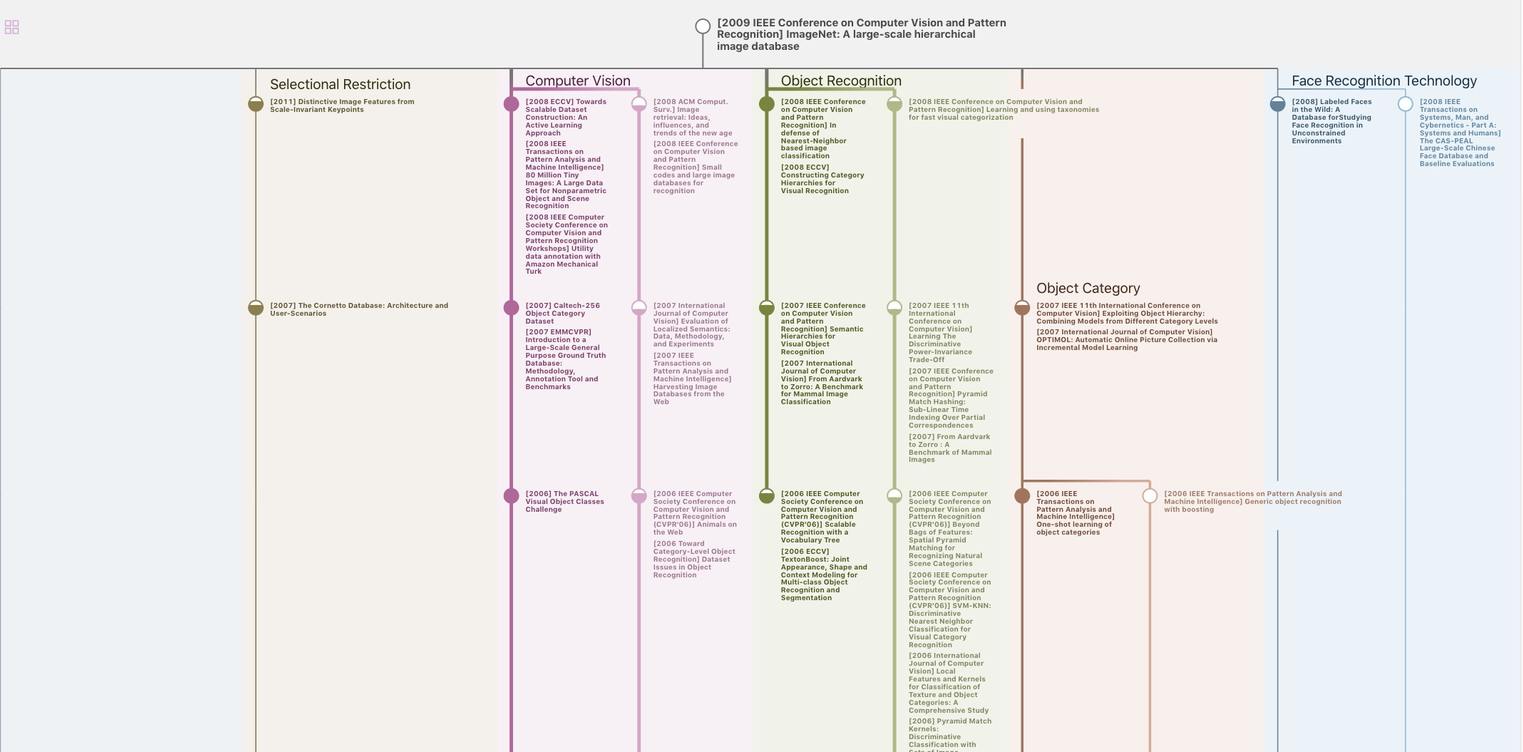
生成溯源树,研究论文发展脉络
Chat Paper
正在生成论文摘要