Use Transfer Learning for Efficient Deep Learning Training on Intel ® Xeon ® Processors
semanticscholar(2018)
摘要
This is an educational white paper on transfer learning, showcasing how existing deep learning models can be easily and flexibly customized to solve new problems. One of the biggest challenges with deep learning is the large number of labeled data points that are required to train the deep learning models them to sufficient accuracy. For example, the ImageNet* [20] database for image recognition consists of over 14 million hand-labeled images. While the number of possible applications of deep learning systems in vision tasks, text processing, speech-to-text translation and many other domains is enormous, very few potential users of deep learning systems have sufficient training data to create models from scratch. A common concern among teams considering the use of deep learning to solve business problems is the need for training data: “Doesn’t deep learning need millions of samples and months of training to get good results?” One powerful solution is transfer learning, in which part of an existing deep learning model is re-optimized on a small data set to solve a related, but new, problem. In fact, one of the great attractions of transfer learning is that, unlike most traditional approaches to machine learning, we can take models trained on one (perhaps very large) dataset and modify them quickly and easily to work well on a new problem (where perhaps we have only a very small dataset). Transfer learning methods are not only parsimonious in their training data requirements, but they run efficiently on the same Intel® Xeon® CPUbased systems that are widely used for other analytics workloads, including machine learning and deep learning inference. The abundance of readily available CPU capacity in current datacenters, in conjunction with transfer learning, makes CPU-based systems the preferred choice for deep learning training and inference.
更多查看译文
AI 理解论文
溯源树
样例
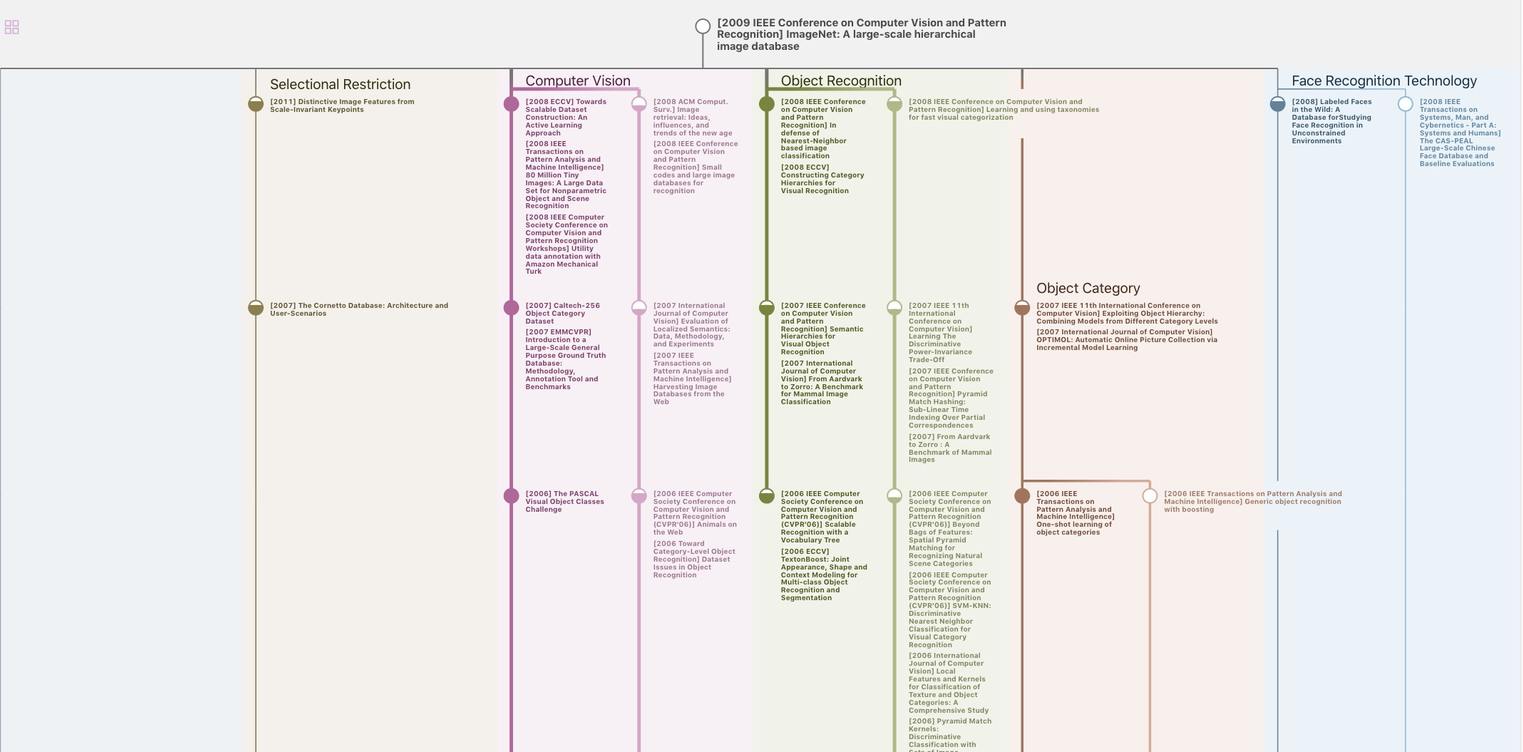
生成溯源树,研究论文发展脉络
Chat Paper
正在生成论文摘要