Modeling and Text Analysis to Empower FAERS Adverse Event Assessment
semanticscholar(2018)
摘要
Timely adverse event pattern detection and resolution, related to drugs, vaccines, and medical devices, can save lives and further quality of life. However, determining conclusions from the data can prove too time consuming for manual analysis, and can produce qualitative results at best. This paper applies a combination of exploratory and predictive text analytic techniques against FAERS data to signal primary textual cues from narratives associated with serious adverse drug events. Specifically, we will run models to generate Boolean combinations of terms and phrases for serious versus non-serious events, including the absence of terms. Additionally, we will assess two characteristic scores tied to drug associations with adverse events via association and market basket analysis. This project provides global observations about the relationship between drugs and adverse events, depicts trends and characterizes the details of serious events, and highlight events misclassified as non-serious for re-investigation. This project serves as proof of concept that modeling and text analysis is useful in adverse event review.
更多查看译文
AI 理解论文
溯源树
样例
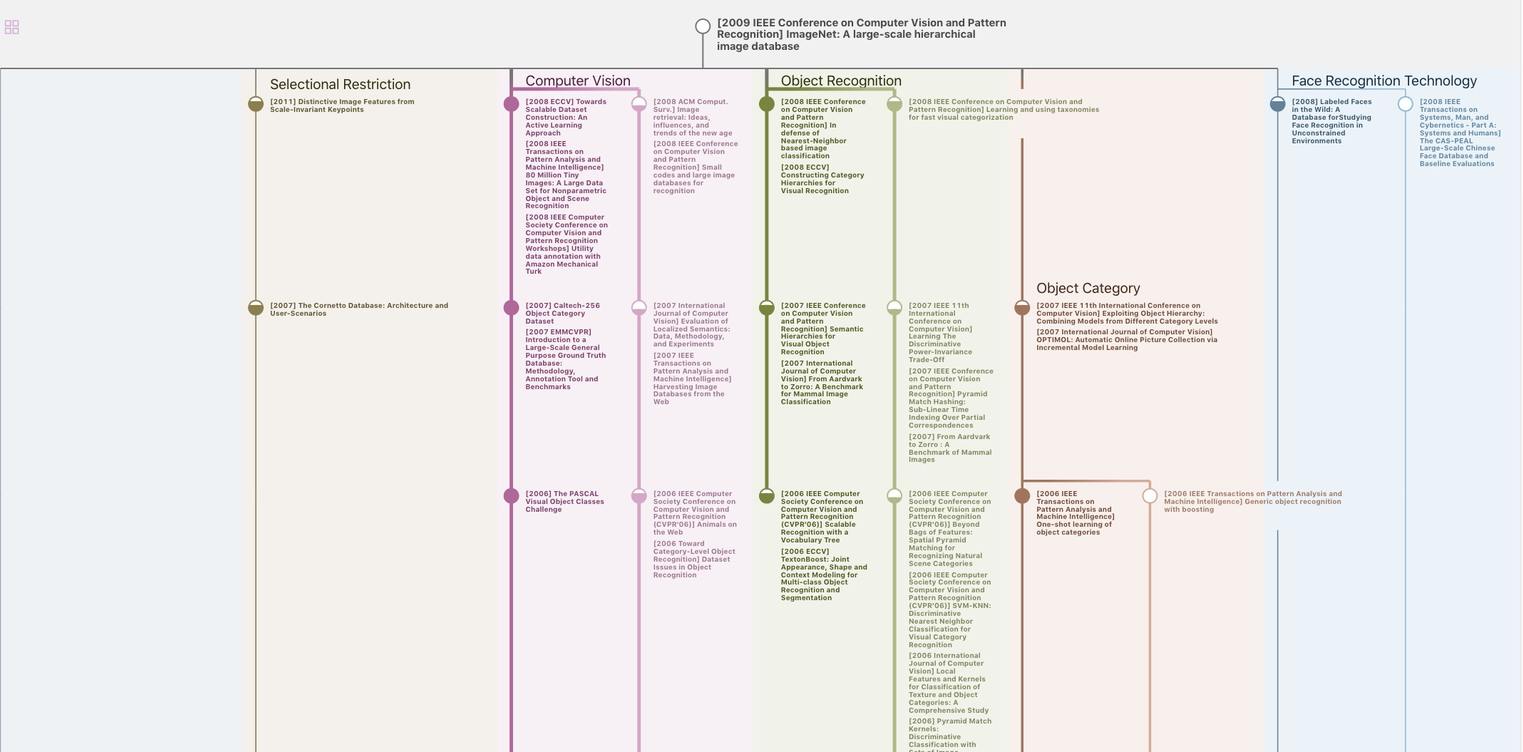
生成溯源树,研究论文发展脉络
Chat Paper
正在生成论文摘要