Breast lesion characterization using quantitative ultrasound mean-values , texture , and texture-derivates
semanticscholar(2019)
摘要
1.1 Breast lesion characterization using quantitative ultrasound mean-values, texture, and texture-derivates, Laurentius Osapoetra, Lakshamanan Sannachi, Hadi Moghadas, Daniel DiCenzo, Karina Quiaoit, Kashuf Fatima, Gregory Czarnota, Department of Radiation Oncology, and Physical Sciences, Sunnybrook Health Sciences Centre, Sunnybrook Research Institute, Toronto ON, Canada, gregory.czarnota@gmail.com Objectives: Previous studies have demonstrated the efficacy of QUS parametric maps in conjunction with textural features for non-invasive characterization of breast lesions. In this study, we expand the QUS feature space through the addition of texture-derivates and perform breast lesions characterization in a larger cohort of patients. Methods: Radiofrequency (RF) breast ultrasound data were acquired from 193 patients (101 benign and 92 malignant) with suspicious breast lesions using clinical ultrasound imaging system, operating at a 6 MHz transmit frequency. QUS Spectral parameters including mid-band fit (MBF), spectral slope (SS), and spectral intercept (SI) and backscatter coefficient parameters including average scattering diameter (ASD) and average acoustic concentration (AAC) were calculated from the tumor. Subsequently, texture analysis using gray-level co-occurrence matrices (GLCM) was performed on QUS parametric maps, resulting in quantitative measures of contrast (CON), correlation (COR), energy (ENE), and homogeneity (HOM) that reflects intra-tumor heterogeneity. Additionally, texture-derivate analysis was performed for each QUS parameter using a sliding window analysis to create textural images, from which second-pass "texture of texture" textural features were extracted from. Nine features were selected to train a machine learning algorithm identifying breast lesions as either “benign” or “malignant”. Three different classifiers including linear discriminant, k-nearest neighbors (KNN), and support vector machines (SVM) were implemented and evaluated. Results: Among the biomarkers investigated, one mean-value, 16 textural, and 40 texture-derivate features showed statistically-significant differences (p<0.05) between the two lesion types. Among the different classifiers, KNN demonstrated the highest prediction results with a sensitivity=77%, specificity=72%, and accuracy=75%. Conclusion: Mean-values of QUS parametric maps, along with texture and texture-derivates demonstrate a high potential for robust, noninvasive and accurate characterization of breast lesions. This work lays the foundations toward adapting novel QUS-based frameworks for breast cancer screening and rapid diagnosis in clinic.
更多查看译文
AI 理解论文
溯源树
样例
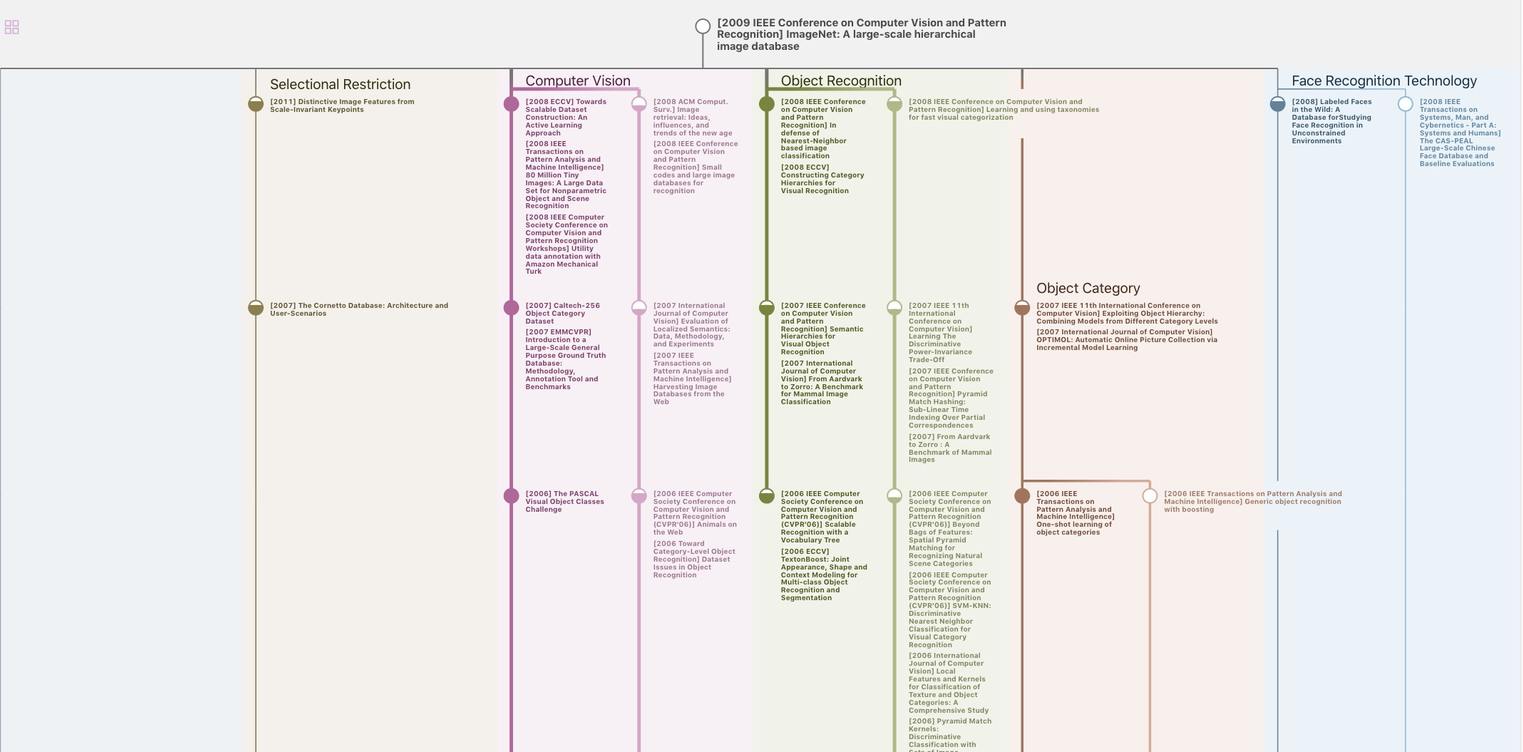
生成溯源树,研究论文发展脉络
Chat Paper
正在生成论文摘要