Visual saliency-based babbling of unknown dynamic environments
semanticscholar(2015)
摘要
Our everyday environment contains many different objects and we are frequently confronted to new objects, may it be known objects with a new shape or color, or completely new objects (smartphones or tablet computers, for instance, did not exist at all in our environment a few years ago). A robot working in our environment should then be able to deal with such modifications. It should in particular be able to identify these objects and what to do with them, i.e. their affordances. Human infants learn these affordances through an interaction with the environment called body babbling [1]. Developmental robotics [2] encourages applying the same exploration step in robots. But, how to define an environment exploration strategy that would work on any kind of environment and object that the robot may encounter before knowing them and their features? A widely used hypothesis consists in restricting the scenario composition, providing this a priori information to help segmenting the visual scene and then to help the babbling to focus on the objects thus identified. Typical hypotheses are that objects lay on a flat surface [3], or can be discriminated by an easy to detect color [4]. Although these approaches can perform properly in isolated controlled environments they would fail in open-ended scenarios, where it is not possible to envision all possible situations [5]. Other hypotheses are based on how humans attention is attracted by specific regions of the scene based on their visual saliency [6]. It is based on the variation of some properties in a visual scene (as color, intensity, shape, or orientation). Previous works ([7], [8], [9]) create a saliency map based on one or more properties of the scene. Interaction with the scene might yield new details to improve the saliency map [10]. However, this interaction should be guided without a priori information of the environment. In this work we propose an autonomous babbling of unknown environments, named Visual Saliency Babbling, driven by the salient regions of raw images of the scene obtained from a fixed RGB-D camera. First, Visual Saliency Babbling identifies the salient regions of the environment, without any previous scene assumption, and then randomly interacts with one of them using an available inverse kinematics model. Once the region has been reached, the robot’s arm comes back to an initial position, and the modifications that eventually resulted from this interaction are recorded for a future object identifiFig. 1. Steps of the generation of the set of SOI associated to the initial set-up used during the experiment. At the top left, raw image captured by the camera. At the top right, SIFT keypoints computed using the raw image. At the bottom left, supervoxels identified using the point cloud of the initial set-up. At the bottom right, blue regions represent the set of SOI computed using the SIFT keypoints and the supervoxels, projected over the previous point cloud.
更多查看译文
AI 理解论文
溯源树
样例
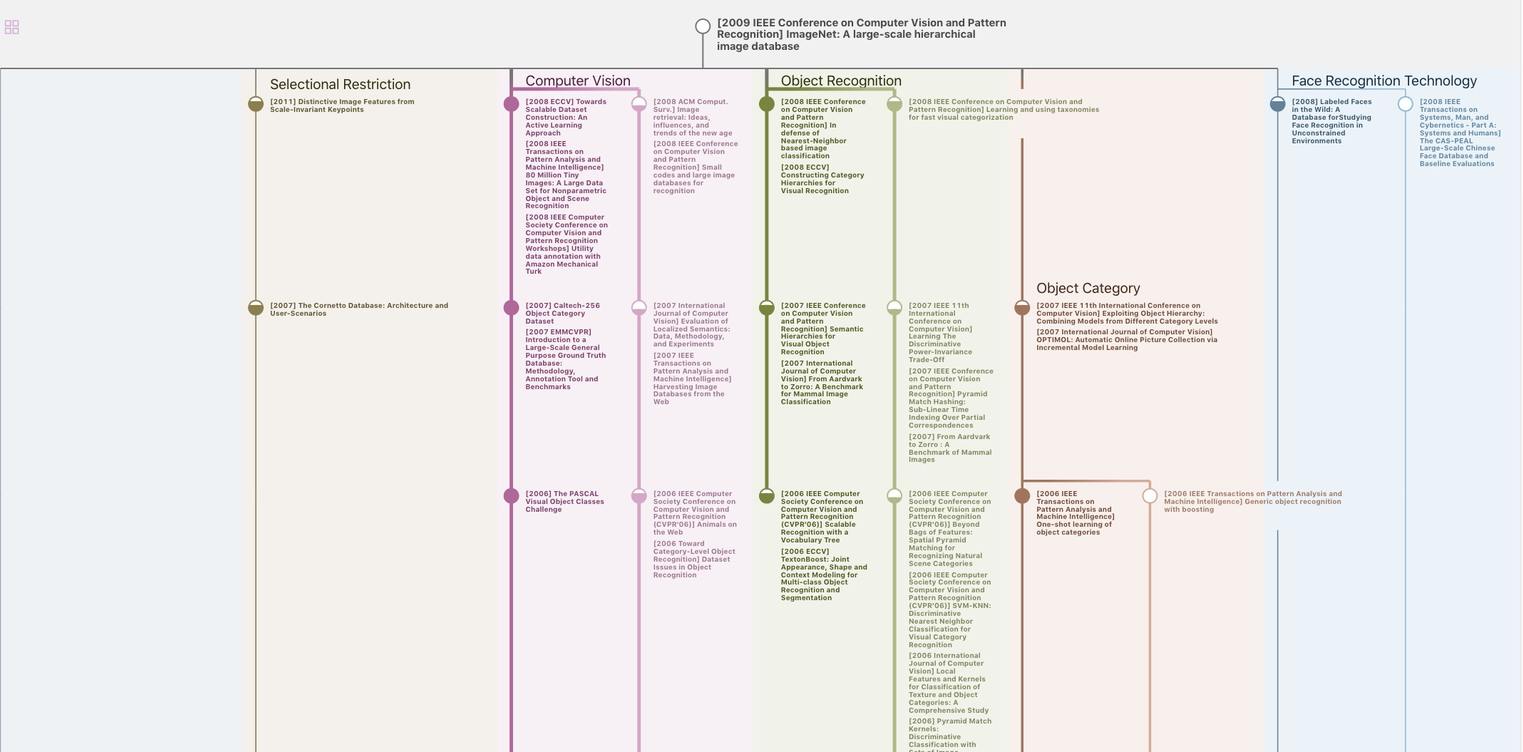
生成溯源树,研究论文发展脉络
Chat Paper
正在生成论文摘要