P4ML: A Phased Performance-Based Pipeline Planner for Automated Machine Learning
Proceedings of Machine Learning Research, ICML 2018 AutoML Workshop(2018)
摘要
While many problems could benefit from recent advances in machine learning, significant time and expertise are required to design customized solutions to each problem. Prior attempts to automate machine learning have focused on generating multi-step solutions composed of primitive steps for feature engineering and modeling, but using already clean and featurized data and carefully curated primitives. However, cleaning and featurization are often the most time-consuming steps in a data science pipeline. We present a novel approach that works with naturally occurring data of any size and type, and with diverse third-party data processing and modeling primitives that can lead to better quality solutions. The key idea is to generate multi-step pipelines (or workflows) by factoring the search for solutions into phases that apply a different expert-like strategy designed to improve performance. This approach is implemented in the P4ML system, and demonstrates superior performance over other systems on a variety of raw datasets.
更多查看译文
AI 理解论文
溯源树
样例
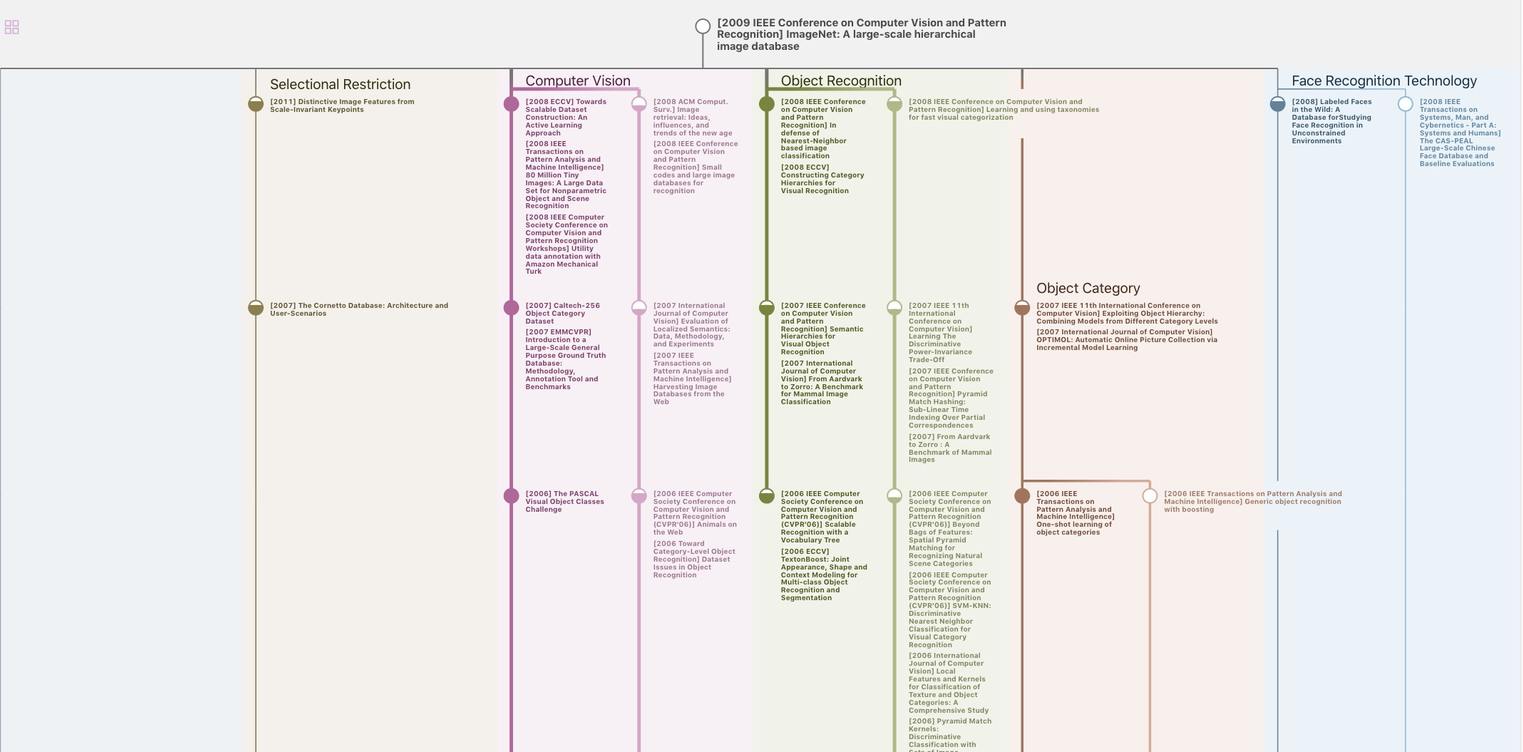
生成溯源树,研究论文发展脉络
Chat Paper
正在生成论文摘要