1 Related Work 1 . 1 Current Transferability Metrics
semanticscholar(2018)
摘要
For successful transfer and meta-learning, it’s critical to have a method for choosing relevant source and target tasks. Existing metrics require empirically evaluating transferability by quantifying time required to achieve benchmark performance on the target task. We would like a metric that can quantify potential for success after transfer, without empirically performing said transfer. In this work, we (1) describe relevant related work, highlighting lack of effective metrics for quantifying transfer in reinforcement learning, (2) introduce AEDist, a modification on the RBMDist transferability metric, (3) introduce a way to train and evaluate the metric, (4) introduce ways to incorporate the metric with ModelAgnostic Meta-Learning (MAML) by: guiding the learning of the metric using existing empirical metrics in MAML, guiding the learning of the MAML metalearner using AEDist, and mutually training the AEDist metric and the MAML meta-model, (5) evaluate all above models, (6) pose relevant research questions for directions of future work in expanding upon the AEDist transferability metric, and (7) create the first MuJoCo tensegrity environment, capable of locomotion with model-free learning, and (8) motivate research into transferability of tensegrity locomotion policies across environments with varying degrees of terrain incline. Our main takeaways are that (1) low AEDist is in fact predictive of tasks to which we can successfully transfer, in terms of immediate performance, asymptotic performance, and in terms of total return, and (2) incorporation of AEDist into the MAML meta-objective is not conducive to better MAML few-shot learning (neither MAML-return guided AEDist, nor AEDist-guided training of the MAML meta-learner, nor mutual guidance by both AEDist and empirical metrics). Our main contributions are as follows: • We introduce a “task-transferability” metric and evaluate its effectiveness as a stand-alone, and in combination with Model-Agnostic Meta-Learning. • We provide insight into transferability of Tensegrity locomotion policies across terrains of varying inclines, and motivate further work both in the “tasktransferability” metric, and its use in the domain of Tensegrity robotics.
更多查看译文
AI 理解论文
溯源树
样例
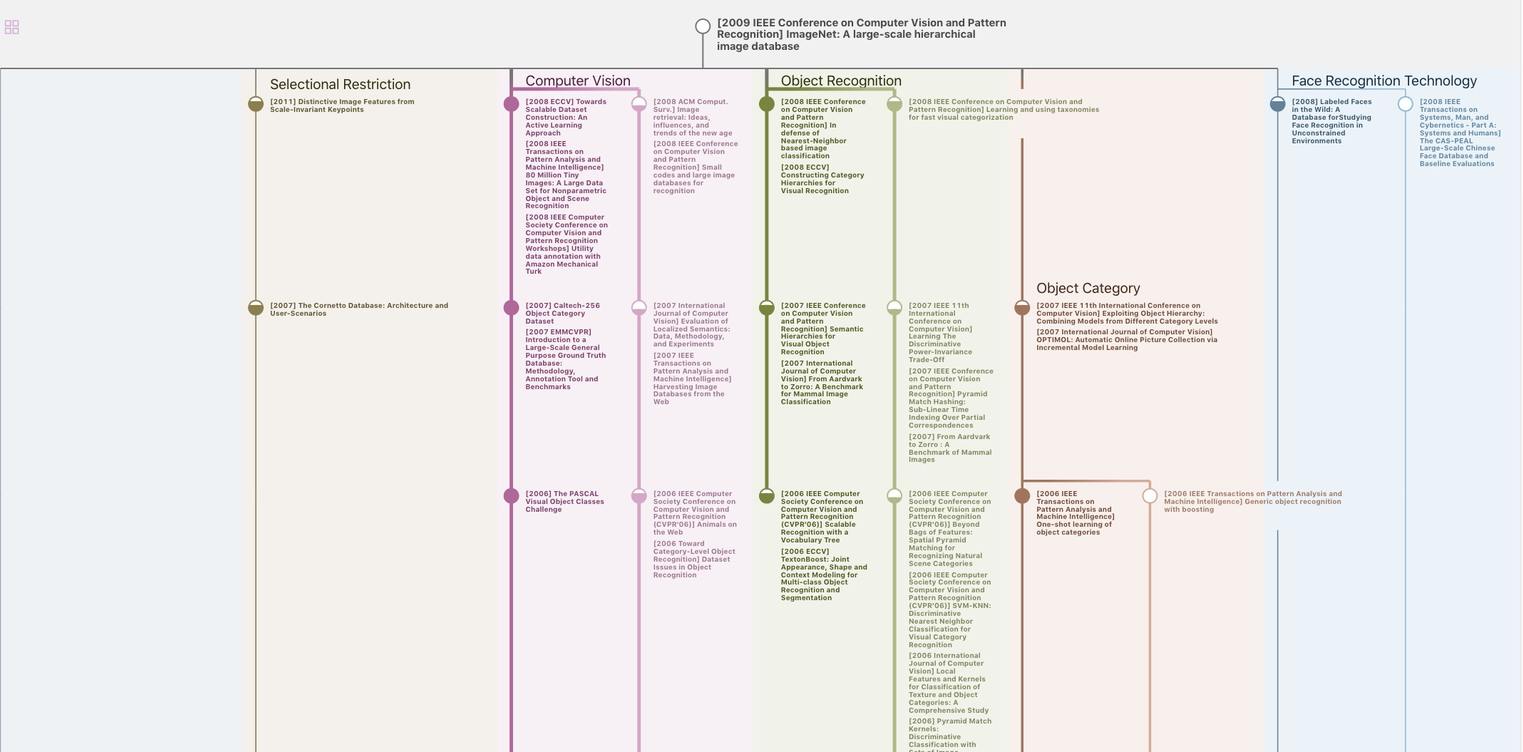
生成溯源树,研究论文发展脉络
Chat Paper
正在生成论文摘要