Understanding Performance Gains of Accelerator-Rich Architectures ( Invited Paper )
semanticscholar(2019)
摘要
The power and utilization walls in today’s processors have led to a recent focus on accelerator-rich architectures (ARAs), which include a sea of customized accelerators with orders-of-magnitude performance and energy gains. Meanwhile, some researchers wonder how the reported large gains are achieved, considering that ARAs use a similar memory hierarchy to conventional processors. In this paper we conduct an in-depth analysis of ARAs with a key focus on the memory access component not studied in prior work. Based on our experimental results, we observe that ARAs achieve performance gains from both computation and memory access customization. For computation customization, ARAs not only exploit the coarse-grained parallelism as conventional processors do, but also uniquely customize a deep processing pipeline without instruction overhead. For memory access customization, ARAs exploit a tile-based read-compute-write execution model that both reduces the number of memory accesses and improves the memory-level parallelism (MLP). We quantitatively evaluate the performance impact of such factors and surprisingly find that 1) memory access customization plays a bigger role in the performance improvement than computation customization, and 2) the dominating contributor to the ARA memory access performance improvement is the improved MLP rather than the widely-expected memory access reduction. Indeed, we find that existing GPU accelerators also benefit from the improved MLP through different techniques. The unique customized deep processing pipeline of ARAs further provide an average of 1.4x speedup over GPUs. Moreover, on average, ARAs are 18x more energy efficient over GPUs. We hope this understanding can help future ARA design and adoption.
更多查看译文
AI 理解论文
溯源树
样例
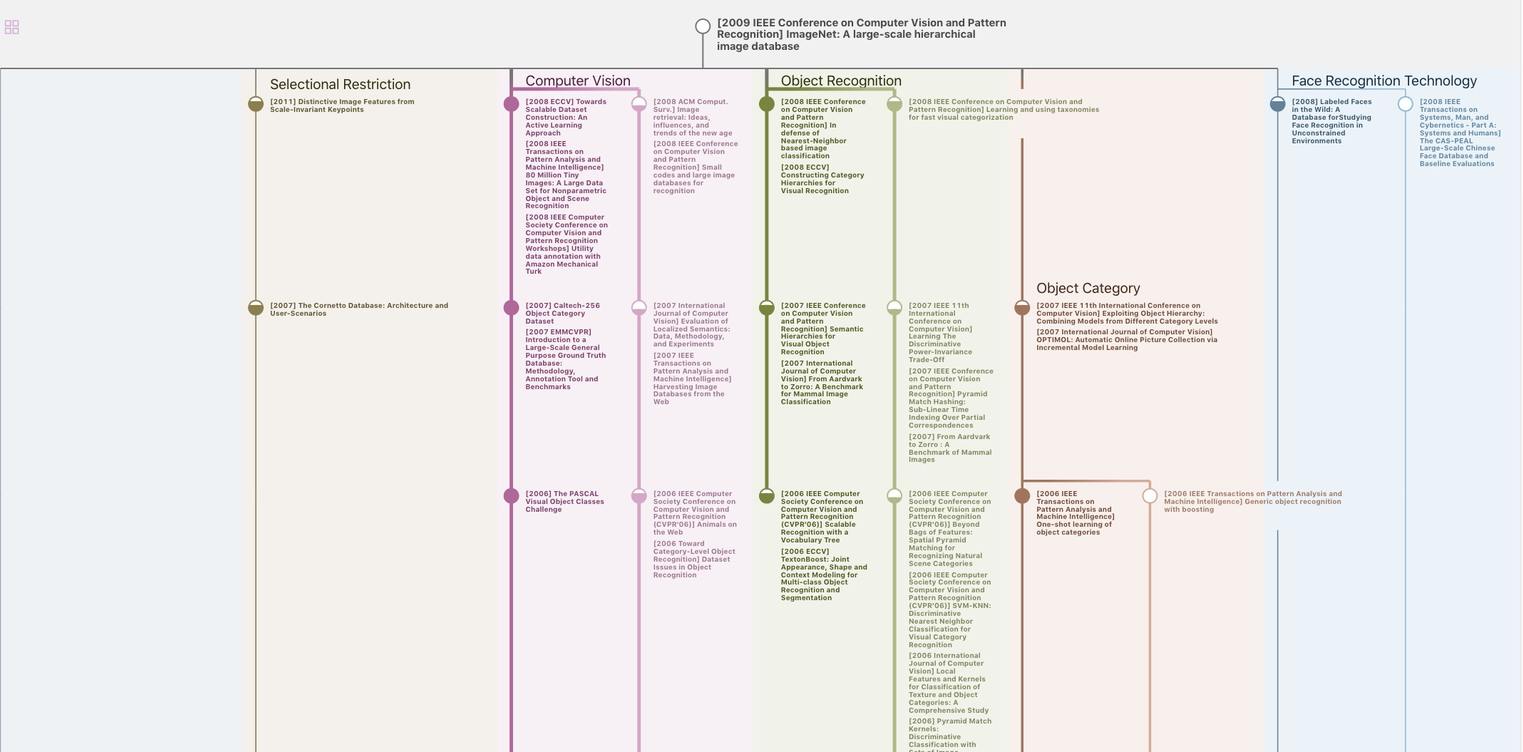
生成溯源树,研究论文发展脉络
Chat Paper
正在生成论文摘要