Multivariate Spatiotemporal Hawkes Processes and Network Reconstruction | SIAM Journal on Mathematics of Data Science | Vol. 1, No. 2 | Society for Industrial and Applied Mathematics
semanticscholar(2019)
摘要
There is often latent network structure in spatial and temporal data, and the tools of network analysis can yield fascinating insights into such data. In this paper, we develop a nonparametric method for network reconstruction from spatiotemporal data sets using multivariate Hawkes processes. In contrast to prior work on network reconstruction with point-process models, which has often focused on exclusively temporal information, our approach uses both temporal and spatial information and does not assume a specific parametric form of network dynamics. This leads to an effective way of recovering an underlying network. We illustrate our approach using both synthetic networks and networks that we construct from real-world data sets (a location-based social-media network, a narrative of crime events, and violent gang crimes). Our results demonstrate that, in comparison to using only temporal data, our spatiotemporal approach yields improved network reconstruction, providing a basis for meaningful subsequent analysis---such as examinations of community structure and motifs---of the reconstructed networks.
更多查看译文
AI 理解论文
溯源树
样例
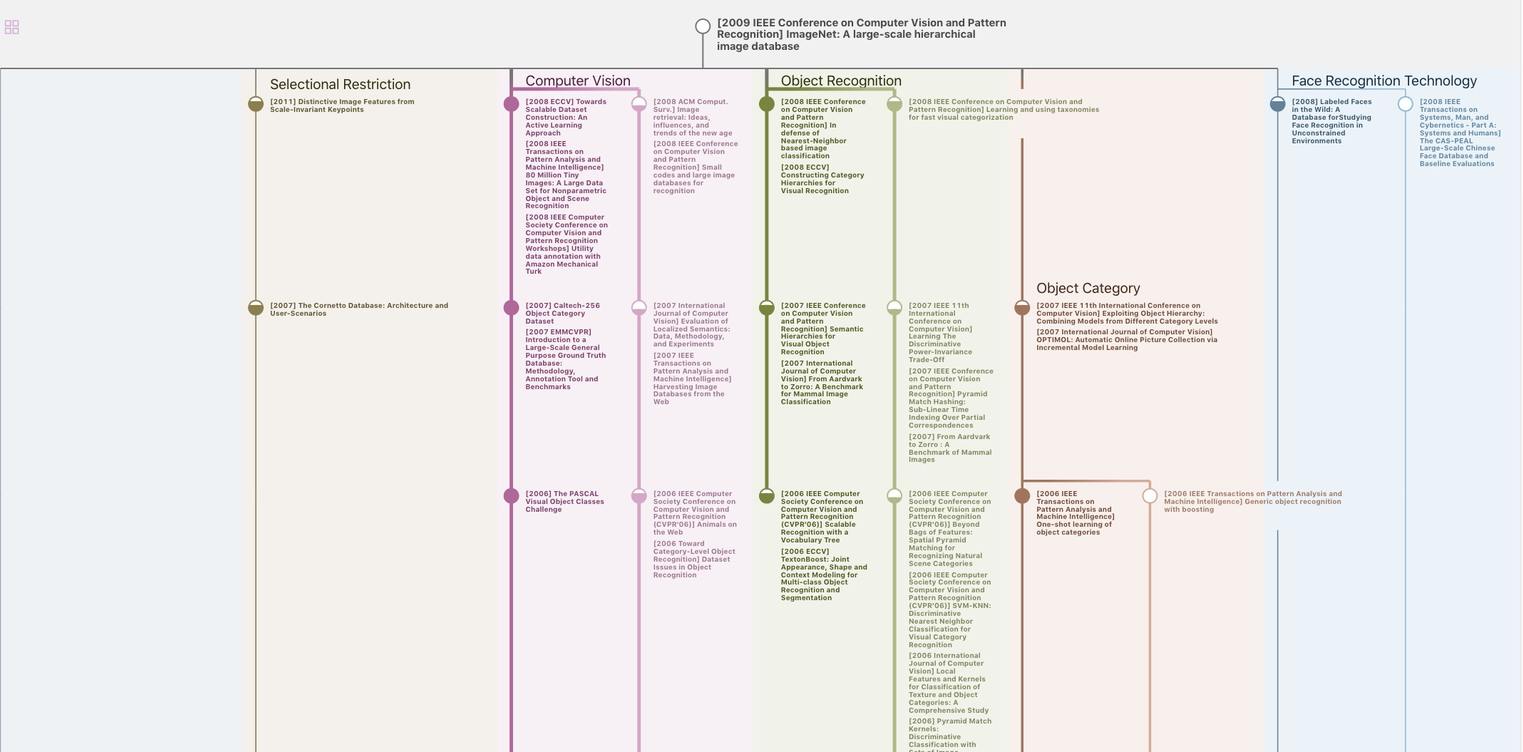
生成溯源树,研究论文发展脉络
Chat Paper
正在生成论文摘要