Explanation for defeasible entailment
semanticscholar(2019)
摘要
Description Logics (DLs) are well-known formalisms for reasoning about information in a given domain. DLs have many advantages such as being decidable fragments of First-Order Logic, and having a clear semantics and well-defined reasoning procedures which can be automated [7, 2]. Take the classic penguin example, and consider a knowledge base containing the statements: “penguins are birds”, “robins are birds”, “penguins do not fly”, “birds fly” and “birds have wings”.We can use the well-defined syntax and semantics of DLs to define entailment which allows us to derive implicit knowledge that can be made explicit through inferences [2]. For example using the information above we can query the knowledge base, ask “do robins have wings”, and the answer would be YES. DLs employ various reasoning services such as concept satisfiability, subsumption, consistency checking and instance checking which can be used to derive useful implicit information from knowledge bases. Reductions between reasoning services also enable only one reasoning procedure to be implemented which alleviates the need of creating tools to perform each and every reasoning service [9, 10]. Various reasoning techniques/algorithms have been developed to solve some of the reasoning problems highlighted above. The most widely used technique, the tableau-based approach, have been shown to be efficient in practice for real knowledge bases [2]. The DLs services mentioned above can be more useful by adding explanations to the conclusions that DLs systems can draw. Using the example above, the answer to our query “do robins have wings” was YES. However, it is more beneficial to users if the DL system can also provide an explanation of how it came to the conclusion. In this example an explanation to the query is that “we know that robins are birds, and birds have wings, therefore we can conclude that robins have wings”. Explanation facilities are useful in understanding entailments, debugging and repairing information declared in knowledge bases and also knowledge base comprehension. In our example above our knowledge base is very small with only five statements. In reality knowledge bases can contain ten of thousand of statements and without automated support for explanation, it can be difficult to identify the statements that give rise to entailments [3, 6]. There are various algorithms to compute justifications, and implementations of these algorithms for the DL case are available through the ontology editor Protégé [6]. Classical DLs cannot deal with exceptional cases. For this reason, there have been numerous proposals to define non-monotonic reasoning systems. One such approach is the KLM approach to defeasible reasoning, which was originally
更多查看译文
AI 理解论文
溯源树
样例
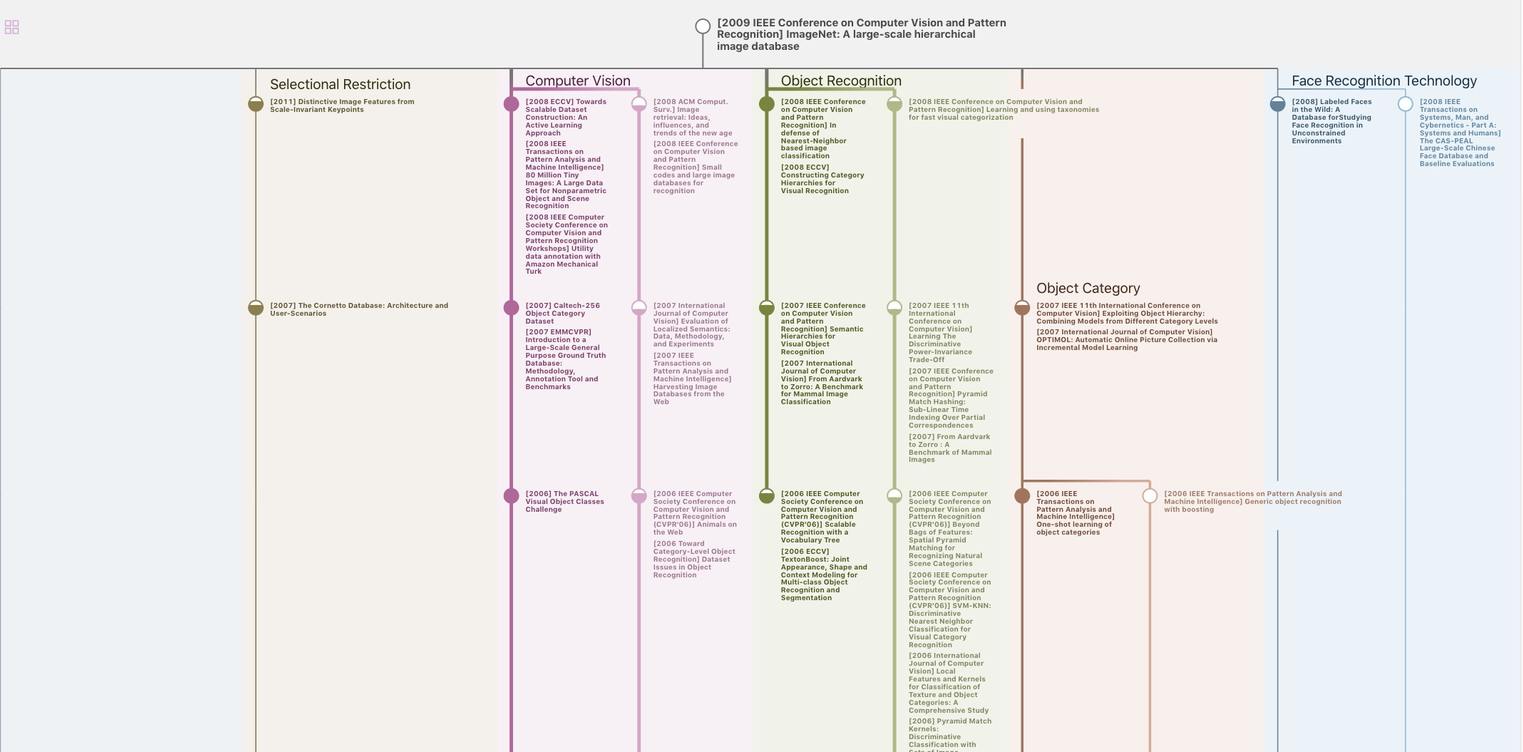
生成溯源树,研究论文发展脉络
Chat Paper
正在生成论文摘要