O Lp- A S Csp
semanticscholar(2010)
摘要
In a beautiful result, Raghavendra established optimal Unique Games Conjecture (UGC)-based inapproximability of a large class of constraint satisfaction problems (CSPs). In the class of CSPs he considers, of which M C is a prominent example, the goal is to find an assignment which maximizes a weighted fraction of constraints satisfied. He gave a generic semi-definite program (SDP) for this class of problems and showed how the approximability of each problem is determined by the corresponding SDP (upto an arbitrarily small additive error) assuming the UGC. He noted that his techniques do no apply to CSPs with strict constraints (which must be satisfied) such as V C and G-3-C. In this paper we address the approximability of these strict-CSPs. In the class of CSPs we consider, one is given a set of constraints over a set of variables, and a cost function over the assignments, the goal is to find an assignment to the variables of minimum cost which satisfies all the constraints. We present a natural linear program (LP) for a large class of strict-CSPs and give a UGC-based reduction to show how this LP captures their approximability up-to an arbitrarily small additive error. Some important problems our framework captures are V C, H V C, k-partite-H V C, I S and other covering and packing problems over q-ary alphabets, and a scheduling problem. For the covering and packing problems, we also provide a matching rounding algorithm. One goal of this paper is to provide a conceptual framework in which one can derive LP-based inapproximability results. Though, we make no attempt to list all the applications, the ones we do mention should provide evidence to more applications in the future. Like Raghavendra’s result in the SDP world, we derive probability distributions from the LP of a finite instance of the problem whose approximability we are trying to establish and then construct a dictatorship test based on these probabilities. The reduction and its analysis di ffers from his as we have to distinguish between variables and our constraints are strict. The soundness analysis of our reduction relies on the Invariance Principle of Mossel [Mos08] along-with some gaussian estimates. ∗Dept. of Computer Science and Engineering, IIT Delhi, New Delhi, email: am tk@cse.iitd.ac.in †Department of Computer Science, Princeton University, email: rajsekar@cs.princeton.edu. Supported by NSF Grants CCF0832797, 0830673, and 0528414 ‡Institute for Advanced Study, Princeton, email: madhurt@ias.edu. Supported by NSF grant 0832797 and IAS sub-contract no. 00001583. §Microsoft Research India, Bangalore, email: n sheeth.vishnoi@gmail.com
更多查看译文
AI 理解论文
溯源树
样例
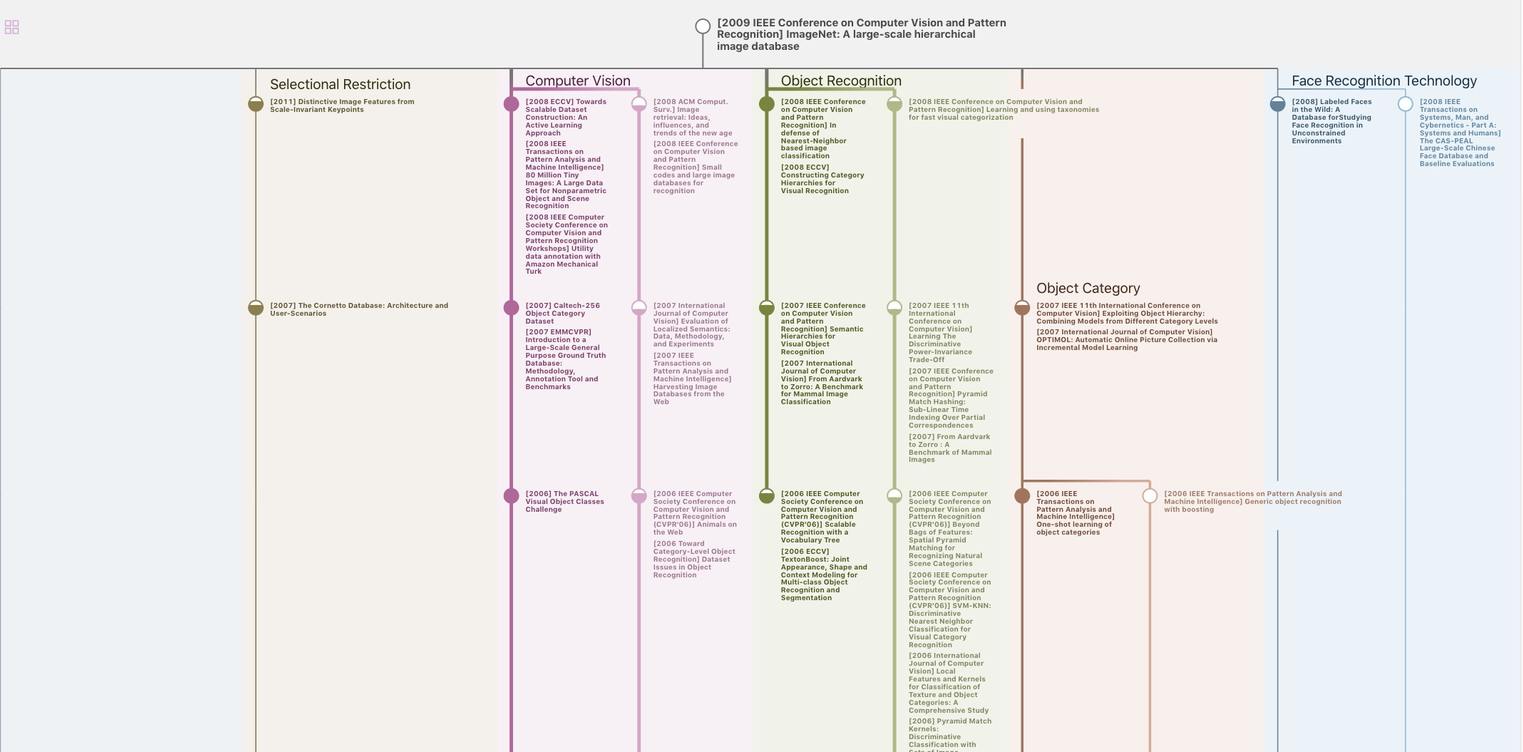
生成溯源树,研究论文发展脉络
Chat Paper
正在生成论文摘要