How high can we go? Evaluating massively high-dimensional propensity score models in large-scale observational studies
semanticscholar(2015)
摘要
Large-scale observational studies that fully utilize the information available in healthcare databases can include millions of patients and unique measurements of their health. These massively highdimensional scenarios pose challenges in developing propensity score and outcome models for conducting cohort studies to examine drug safety or comparative effectiveness. We have developed novel OHDSI tools that implement the high-dimensional propensity score (hdPS) algorithm and massive sample-size, regularized regression (MSSRR) methods in constructing comparable patient cohorts. We plan to evaluate the performance of both propensity score approaches through measures of cohort balance and through estimation of treatment effect when coupled with an outcome model. Comparison studies are conducted through data simulation and through analyzing several real-world drug safety issues at scale. We wish to characterize the capabilities of different propensity score and outcome models on the largest scales necessitated by observational healthcare data analysis. Introduction The specification of propensity score models to identify comparable patients is a crucial decision in conducting observational studies. In dealing with healthcare claims databases where the number of patients and variables alike can range in the millions or more, an investigator cannot know based on expert knowledge alone the exact covariates to include in a propensity score or outcome model. Variable selection techniques are needed to facilitate this process. The high-dimensional propensity score (hdPS) algorithm is one method for selecting potential confounders for inclusion in a propensity score [1]. Covariates are ranked by their prevalence and by their univariate association with the outcome and/or the treatment; a certain number are then used in the propensity score model. While hdPS has been used for large-scale observational studies, its actual performance compared to standard multivariate methods, such as regularized regression and its more recent OHDSI extensions for massive sample-size, regularized regression (MSSRR) [2], has only been investigated on much smaller scales [3]. MSSRR methods stand as useful alternatives to hdPS for propensity score models in massive observational healthcare settings. In regularized regression, all potential covariates are included in a multivariate regression; a penalty term shrinks coefficients with extreme values towards 0, leaving a subset of the original covariates for inclusion in the final model. The performance of MSSRR in generating propensity scores has not been thoroughly evaluated for large-scale observational studies.
更多查看译文
AI 理解论文
溯源树
样例
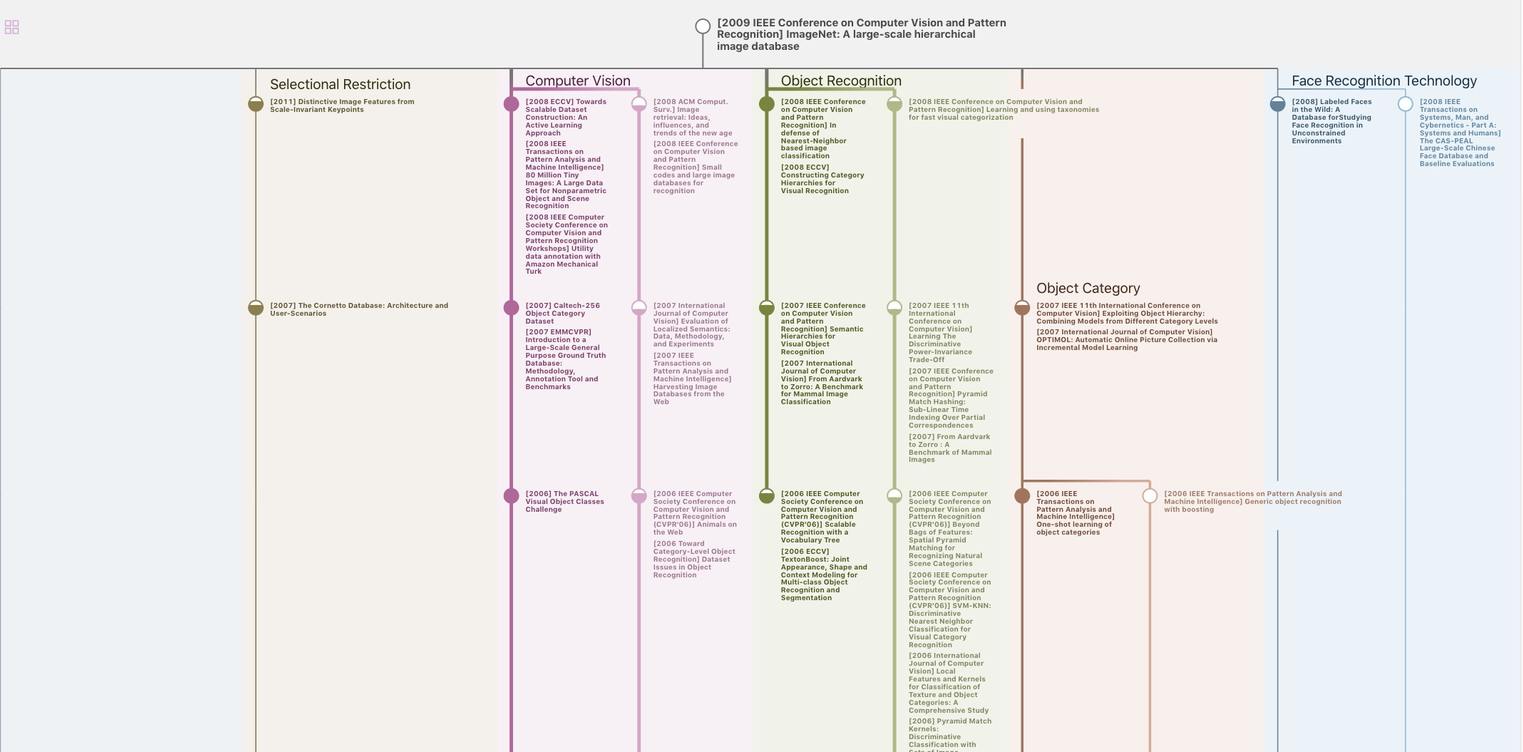
生成溯源树,研究论文发展脉络
Chat Paper
正在生成论文摘要