Privacy-Utility Tradeoff and Privacy Funnel
semanticscholar(2020)
摘要
We consider a privacy-utility trade-off encountered by users who wish to disclose some information to an analyst, that is correlated with their private data, in the hope of receiving some utility. We propose a general framework under which data is transformed according to a probabilistic privacy-preserving mapping before it is disclosed. We show that applying this general framework to the setting where the adversary uses the log-loss cost function naturally leads to a non-asymptotic information-theoretic formulation for characterizing the best achievable privacy subject to utility constraints. This formulation can be cast as a modified rate-distortion problem. We justify the relevance and generality of the privacy metric under the log-loss by proving that the inference threat under any bounded cost function can be upper bounded by an explicit function of the mutual information between private data and disclosed data. We then study connections between our framework and differential privacy. In addition, we show that when the log-loss is used in this framework in both the privacy metric and the utility metric, the average information leakage and the utility constraint can be reduced to the mutual information between private data and disclosed data, and between non-private data and disclosed data, respectively. We then show that the privacy-utility tradeoff under the log-loss can be cast as a non-convex optimization termed Privacy Funnel. Finally, we study the problem of finding optimal privacy-preserving mapping.
更多查看译文
AI 理解论文
溯源树
样例
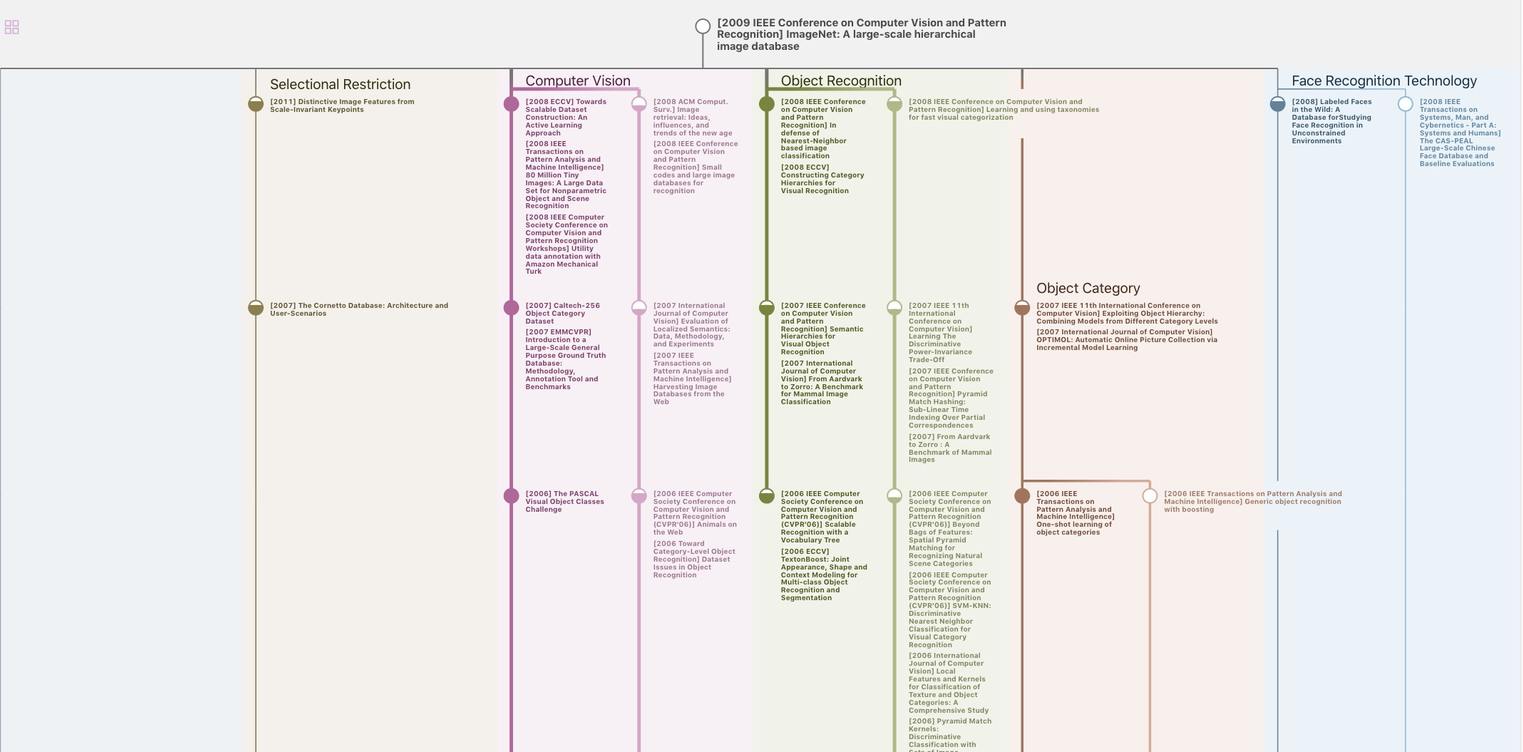
生成溯源树,研究论文发展脉络
Chat Paper
正在生成论文摘要