Forecasting Human Fine-grained Behaviours
semanticscholar(2018)
摘要
For self-driving cars and autonomous delivery platforms, one of the crucial steps to safe and seamless integration of these platforms is a human trajectory prediction module. While selfdriving cars reach good performances in urban environments, crowded scenarios require a more accurate prediction of human-human and human-space interactions. Recent approaches perform the motion forecasting by using only coordinates and velocities of the pedestrians. Inherently, some things are impossible to predict with this representation, e.g., when a pedestrian starts walking or if people recognize and consequently walk towards each other. This work adds human pose information and human activity labels as features to allow a new way of forecasting pedestrian movements. For every human, a time series of bounding boxes, poses, and activities are used to train a Long-Short Term Memory (LSTM) network to predict a future time series of bounding box coordinates. Further experiments will be performed to analyze if predictions for activities and poses are feasible. The LSTM is trained and validated with annotated volleyball and basketball images. In the future, this work should be validated for a broad and general use in human trajectory forecasting.
更多查看译文
AI 理解论文
溯源树
样例
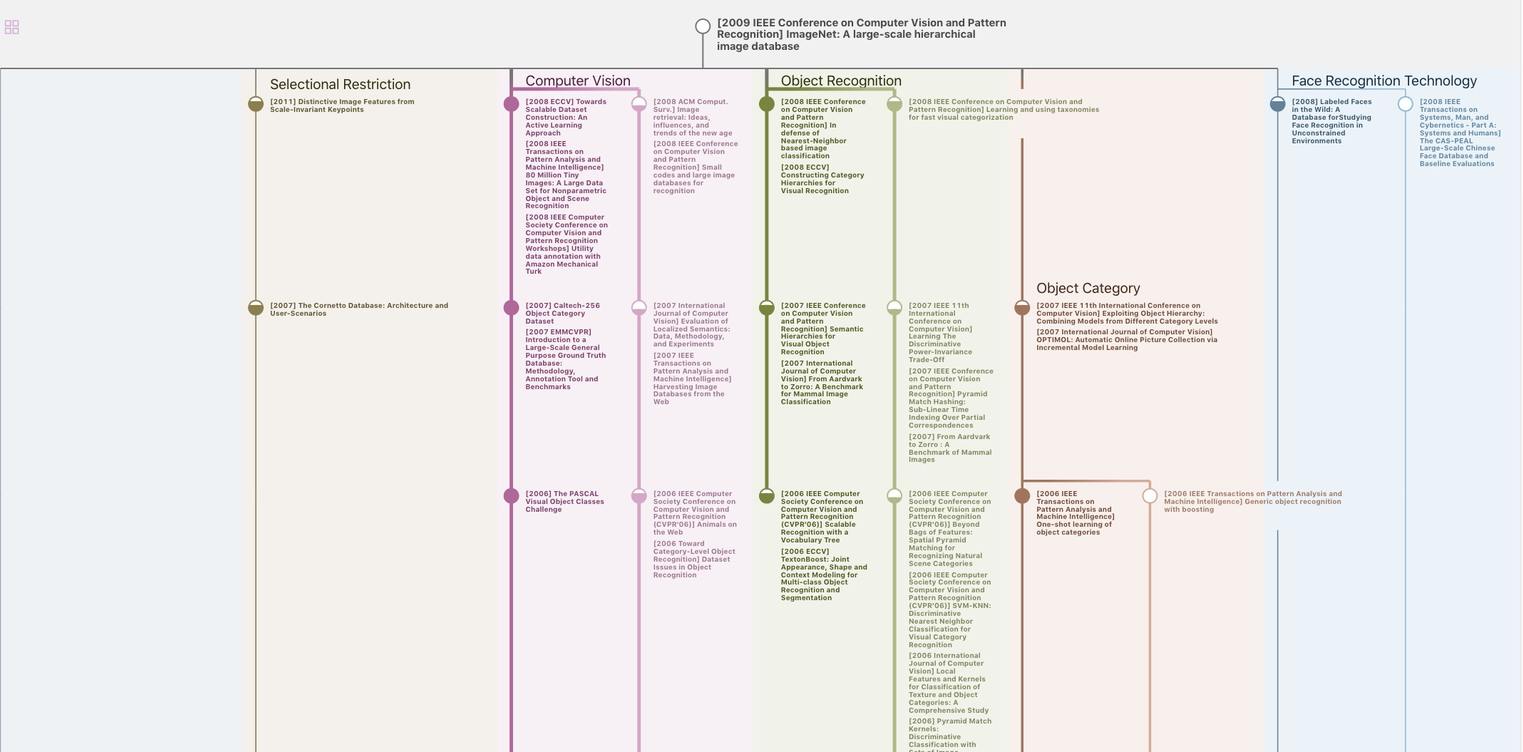
生成溯源树,研究论文发展脉络
Chat Paper
正在生成论文摘要