Assessing brain effective connectivity from EEG – a simulation study
semanticscholar(2012)
摘要
Information flow between brain areas from EEG measurements is hard to estimate reliably due to the presence of noise, as well as due to volume conduction. We present results of a simulation study, in which we test the ability of popular measures of effective connectivity to detect neuronal interactions, as well as machine learning approaches to inverse source reconstruction and blind source separation to improve the estimation. We find that volume conduction limits the neurophysiological interpretability of sensor-space connectivity analyses and may even lead to conflicting Preprint submitted to NeuroImage 15 May 2012 results. On the other hand, the estimation of connectivity on the basis of source activity, which has been estimated with some of the tested machine learning methods, yields correct and interpretable results. The success here crucially depends on the correctness of the source reconstruction, which in turn depends on the capability of the method to model multiple spatially-distinct interacting sources. As a novel methodological contribution, we propose to use time-reversed data as surrogates in order to cancel out artifactual confounders that are not related to true interaction, e. g., due to the volume conduction. We show that this novel approach improves the performance of Granger-causal connectivity measures. Finally, integrating the insights of our study, we provide a guidance for measuring information flow in EEG. Software and benchmark data is made available.
更多查看译文
AI 理解论文
溯源树
样例
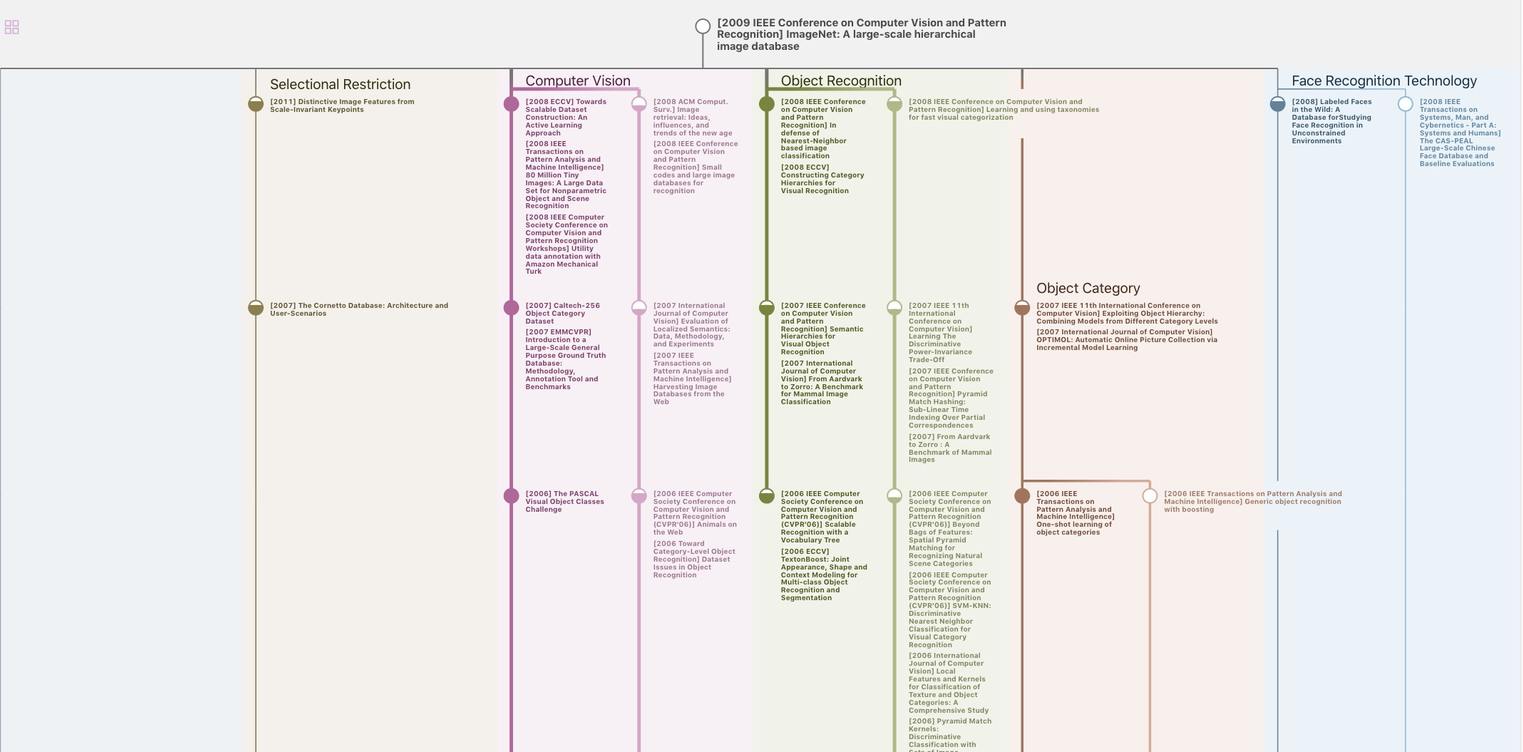
生成溯源树,研究论文发展脉络
Chat Paper
正在生成论文摘要