Ensemble-Learning for Sustainable NLP
semanticscholar(2020)
摘要
Large NLP models result in higher accuracy scores but are more resource intensive on tasks such as QA, incurring a significant environmental cost [1].The question we are addressing is the "Why a task such as QA requires such resource intensive deployment". In order to minimize inference costs while maximizing accuracy at inference time, we develop classifier models that can inform whether we could invoke a small model instead of a big one to answer questions. We found that a small QA model has the potential to answer questions with accuracy comparable to a BERT-based model in approximately 4 in every 5 questions. We find that a rule based ensemble can improve the F1 by over 25% and save 58% of the resources used. We also find that a neural ensemble that is used to predict whether a small model can answer a question correctly covers 50% of the gap between a small model and a big model. Our research recommends the development of NLP classifiers to find more energy efficient deep learning implementations. 1 Key Information to include • Mentor: Matthew Lamm • We have no external collaborators and are not sharing this project. • We choose grading Option 2.
更多查看译文
AI 理解论文
溯源树
样例
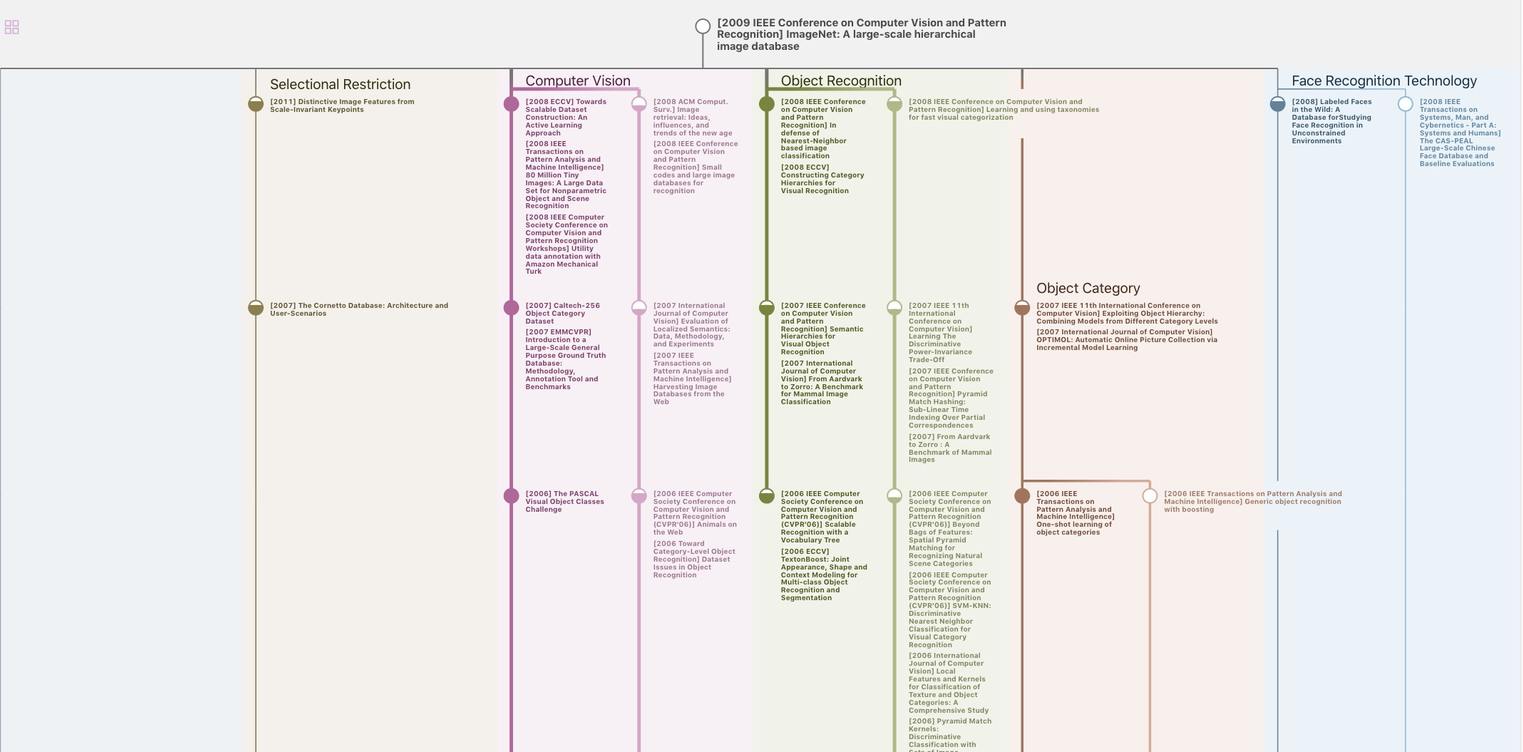
生成溯源树,研究论文发展脉络
Chat Paper
正在生成论文摘要