Multi-View Bipartite Graph Clustering With Coupled Noisy Feature Filter
IEEE TRANSACTIONS ON KNOWLEDGE AND DATA ENGINEERING(2023)
摘要
Unsupervised bipartite graph learning has been a hot topic in multi-view clustering, to tackle the restricted scalability issue of traditional full graph clustering in large-scale applications. However, the existing bipartite graph clustering paradigm pays little attention to the adverse impact of noisy features on learning process. To further facilitate this part of research, apart from simply reweighting features to depress the noisy ones, we take the first step towards analyzing the induced adverse impact via theoretical and experimental investigations. One crucial finding in this article is that the existence of noisy features will incur "anchor shift" phenomenon, which deviates from the potential representations of anchors and then degrades performance. To this end, we propose a coupled noisy feature filter mechanism with automatically finding feature importance to remedy the anchor shift issue in this article. Apart from leveraging features, we theoretically analyze the bounds of proposed feature-adaptive bipartite graph's fuzzy membership. Specifically, distinguishing features' discrimination will increase the fuzzy membership to achieve soft partitions against the potential inaccurate absolute relationships. With the afore-mentioned merits, our proposed multi-view bipartite graph clustering with coupled noisy feature filter model (MVBGC-NFF) provides novel and interesting insights on the feature level of anchor shift. The effectiveness and efficiency of MVBGC-NFF are demonstrated on synthetic and real-world datasets with improved clustering performance, increasing fuzzy membership, and filtering noisy features. The code is available on https://github.com/liliangnudt/MVBGC-NFF.
更多查看译文
关键词
Anchor shift,bipartite graph learning,multi-view clustering,noisy features
AI 理解论文
溯源树
样例
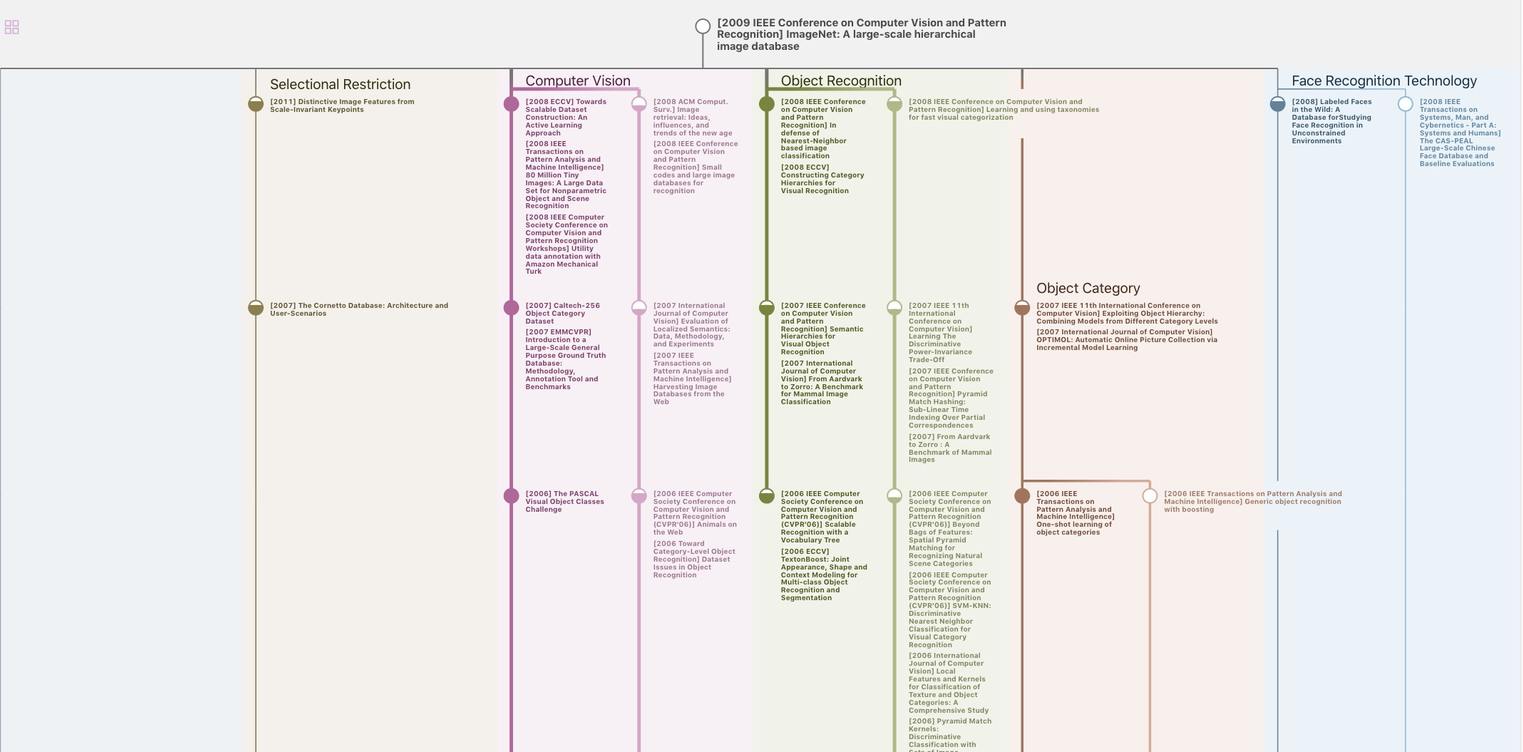
生成溯源树,研究论文发展脉络
Chat Paper
正在生成论文摘要