Learning on the Job : Improving Robot Perception Through Experience
Neural Information Processing Systems (NIPS) Workshop on “Autonomously Learning Robots”, Montreal, Quebec, Canada(2014)
摘要
This paper is about robots that autonomously learn how to interpret their environment through use. Specifically, robot perception is improved with every outing through effortless human assistance. This is made possible by the fact that robots operate repeatedly in specific application domains. Our approach, which we call Experience-Based Classification (EBC), is similar in spirit to the concept of hard negative mining (HNM), but it is entirely self-supervised. In the context of autonomous driving we use 17km of data to show that EBC is a practical alternative to HNM, and demonstrate the advantages of experience-specific classifiers. We believe that our approach presents a fundamental shift in how robot perception is viewed, and advocate for lifelong learning systems that excel in a specific application domain instead of providing mediocre performance everywhere.
更多查看译文
AI 理解论文
溯源树
样例
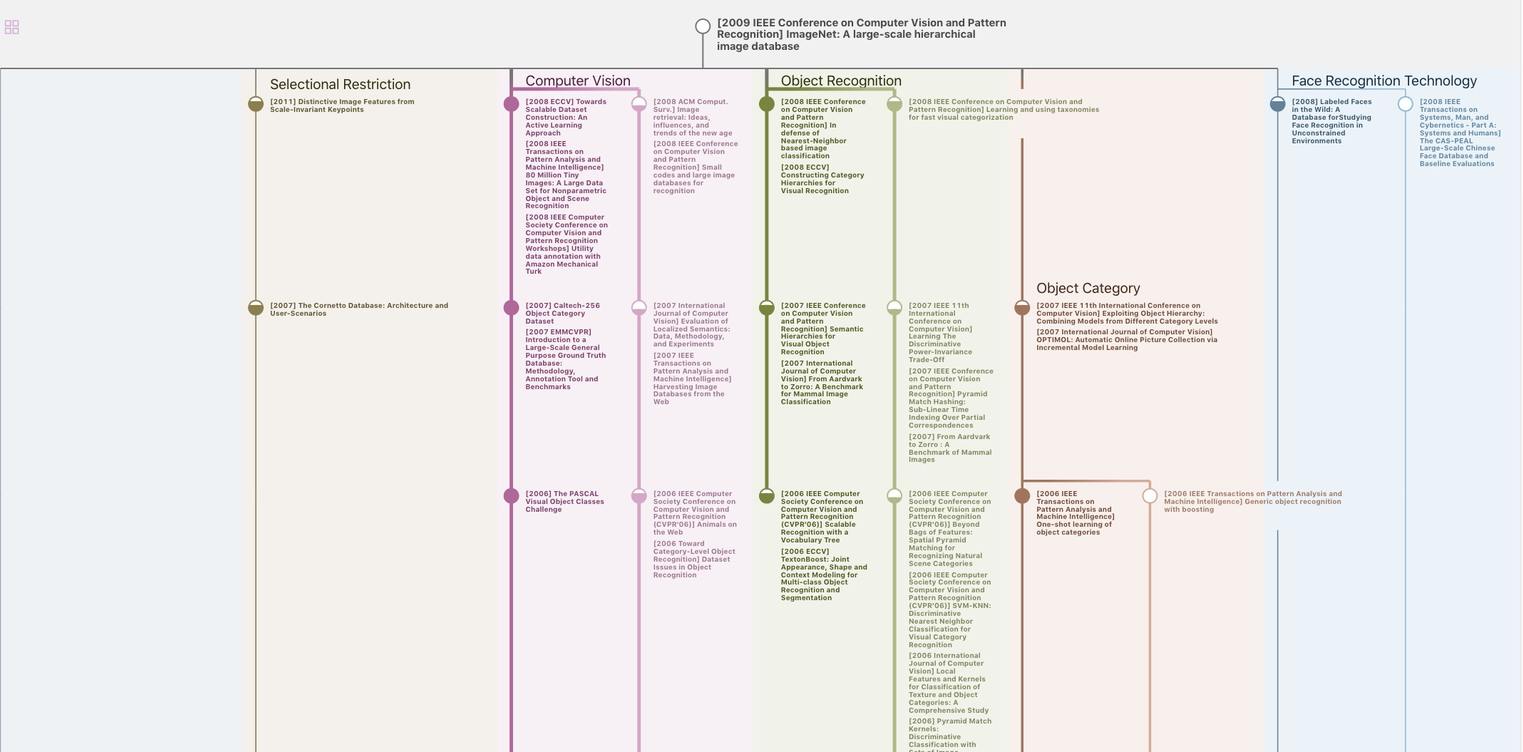
生成溯源树,研究论文发展脉络
Chat Paper
正在生成论文摘要