Learning hierarchical motif embeddings for protein engineering by Thrasyvoulos Karydis
semanticscholar(2017)
摘要
This thesis lays the foundation for an integrated machine learning framework for the evolutionary analysis, search and design of proteins, based on a hierarchical decomposition of proteins into a set of functional motif embeddings. We introduce, CoMET Convolutional Motif Embeddings Tool, a machine learning framework that allows the automated extraction of nonlinear motif representations from large sets of protein sequences. At the core of CoMET, lies a Deep Convolutional Neural Network, trained to learn a basis set of motif embeddings by minimizing any desired objective function. CoMET is successfully trained to extract all known motifs across Transcription Factors and CRISPR Associated proteins, without requiring any prior knowledge about the nature of the motifs or their distribution. We demonstrate that motif embeddings can model efficiently interand intrafamily relationships. Furthermore, we provide novel protein meta-family clusters, formed by taking into account a hierarchical conserved motif phylogeny for each protein instead of a single ultra-conserved region. Lastly, we investigate the generative ability of CoMET and develop computational methods that allow the directed evolution of proteins towards altered or novel functions. We trained a highly accurate predictive model on the DNA recognition code of the Type II restriction enzymes. Based on the promising prediction results, we used the trained models to generate de novo restriction enzymes and paved the way towards the computational design of a restriction enzyme that will cut a given arbitrary DNA sequence with high precision. Thesis Supervisor: Joseph M. Jacobson Title: Associate Professor of Media Arts and Sciences
更多查看译文
AI 理解论文
溯源树
样例
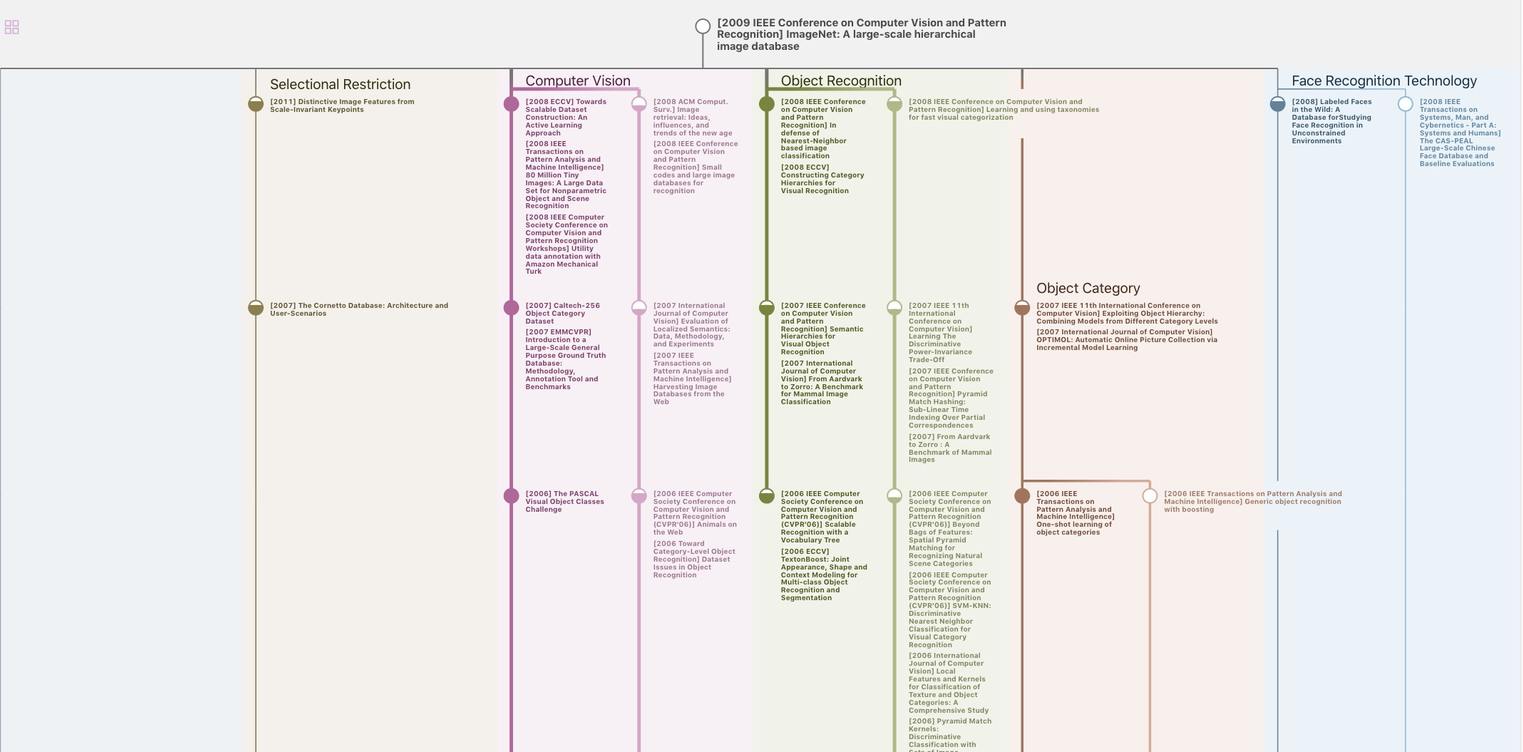
生成溯源树,研究论文发展脉络
Chat Paper
正在生成论文摘要