Search Using Recovered Semantics
semanticscholar(2010)
摘要
We consider the problem of searching for tables in a large table corpus. The Web offers a corpus of 100 million tables, and smaller but sizable corpora are found within enterprises or individual repositories (e.g., data.gov). Table search is challenging because the semantics of the data are typically not explicit in the table itself, and signals that work well for search over document corpora do not apply as well to table corpora. We describe the TableFinder system that partially recovers the semantics of the tables in the corpus, by mapping tables into a database of class labels that is automatically extracted from the Web itself. The database of classes has very wide coverage, but is also noisy. TableFinder identifies a column in each table corresponding to the table’s subject and identifies the classes describing the values in that column. Query answering proceeds by considering tables whose class labels and properties are relevant to the query. We describe experiments that illustrate that TableFinder provides much higher accuracy compared to approaches that extend document search, and we characterize what fraction of tables on the Web can be annotated using our approach.
更多查看译文
AI 理解论文
溯源树
样例
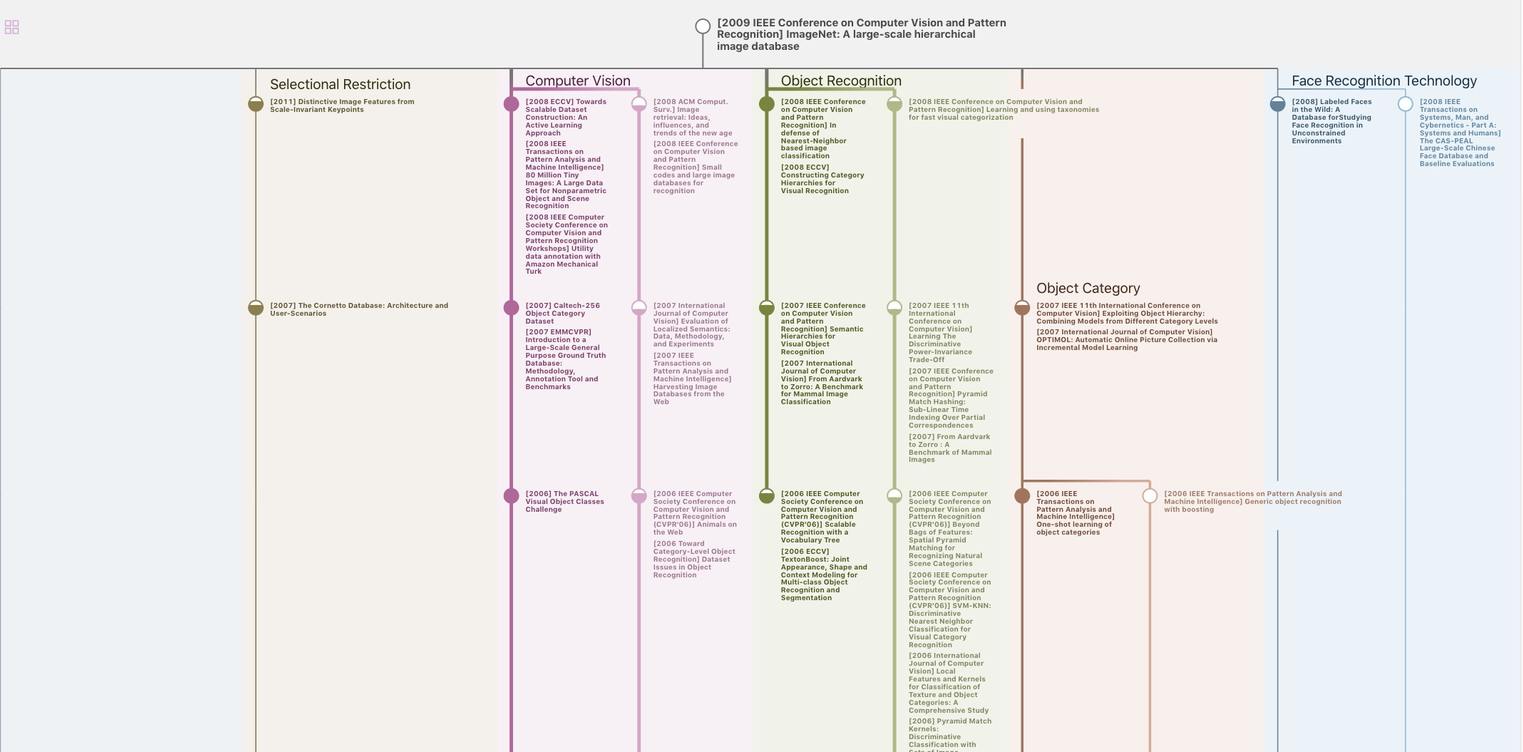
生成溯源树,研究论文发展脉络
Chat Paper
正在生成论文摘要