Empirical Bayes Meta-Learning with Synthetic Gradients
semanticscholar(2019)
摘要
We revisit the hierarchical Bayes and empirical Bayes formulations for multi-task learning, which can naturally be applied to meta-learning. The evidence lower bound of the marginal log-likelihood of empirical Bayes decomposes as a sum of local KL divergences between the variational posterior and the true posterior of each task. We derive an amortized variational inference that couples all the variational posteriors into a meta-model, which consists of a synthetic gradient network and an initialization network. Our empirical results on the mini-ImageNet benchmark for episodic few-shot classification significantly outperform previous state-of-the-art methods. 1 Meta-learning with transductive inference The goal of meta-learning is to train a meta-model on a collection of tasks, such that it works well on another disjoint collection of tasks. Suppose that we are given a collection of N tasks for training. The associated data is denoted by D := {dt = (xt, yt)}t=1. In the case of few-shot learning, we are given in addition a support set dt for each task. In this section, we revisit the classical empirical Bayes model for meta-learning. Then, we propose to use a transductive scheme in the variational inference by constructing the variational posterior as a function of xt. 1.1 Empirical Bayes model Due to the hierarchical structure among data, it is natural to consider a hierarchical Bayes model for the marginal likelihood
更多查看译文
AI 理解论文
溯源树
样例
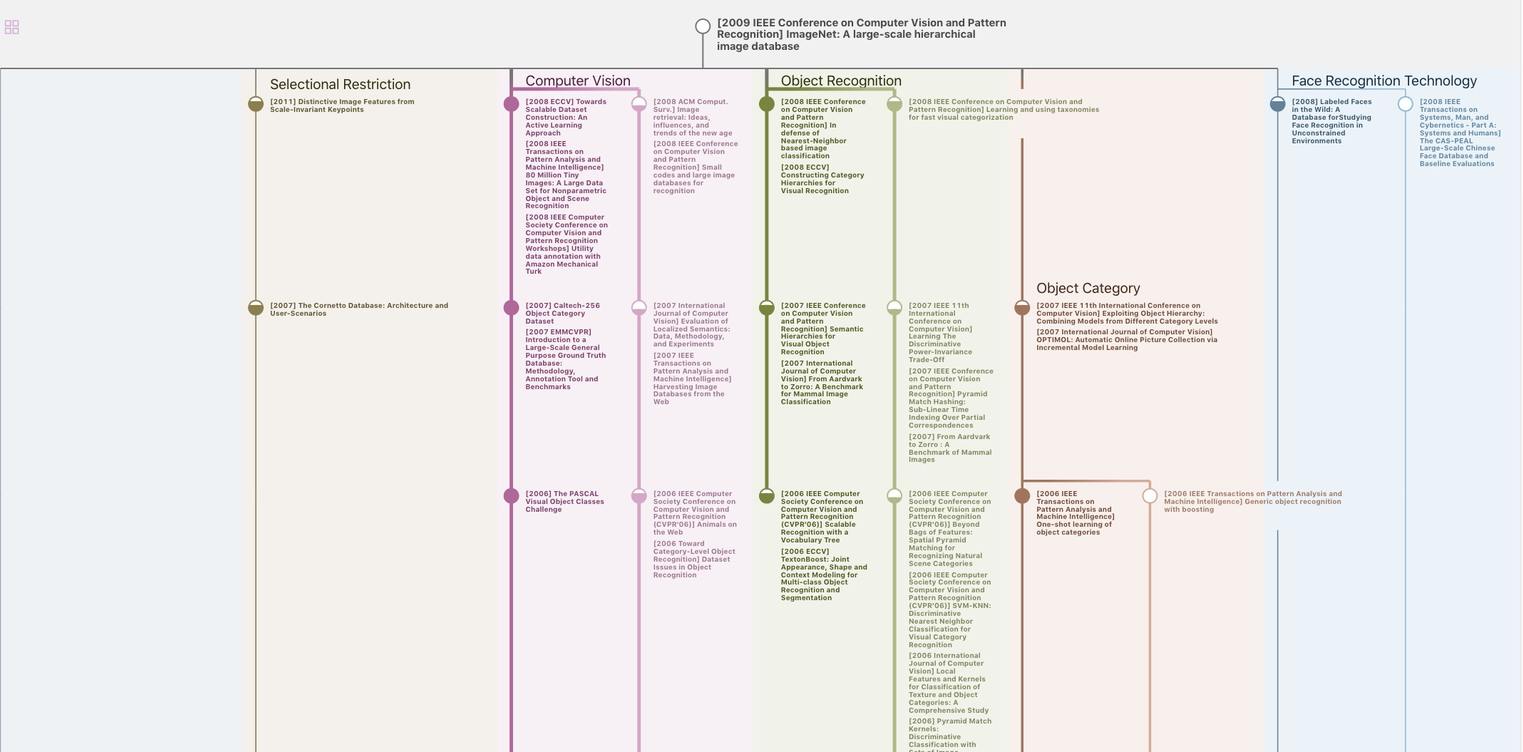
生成溯源树,研究论文发展脉络
Chat Paper
正在生成论文摘要