QoS-Aware Web Service Composition Using Kernel-Based Online Quantile Estimation
semanticscholar(2010)
摘要
Often many available web services provide similar functionality, but have different Quality of Service (QoS). In this case, a choice needs to be made to determine which services are selected to participate in a given service composition. This paper proposes a technique for QoS-aware service composition with the purpose to pro-actively minimize the rate of violations of a service level agreement (SLA) between a customer and the service (composition) provider. In contrast to most existing work on QoS based service composition, we assume time-varying QoS attributes of participating services and use time series prediction to determine an optimal composition. Due to the nature of the predication problem, we propose the use of a kernel-based online quantile estimator. Kernels enable the modeling of non-linearities in QoS attributes with respect to time. Online learning lowers the computational complexity compared to batch learning and allows the model to be easily updated with each new training example. Quantile estimation permits to quantify the violation rate of SLAs. We demonstrate the feasibility of this technique in an existing framework for on-demand service composition. This framework enables a service provider to offer to a customer on-the-fly creation of a customized BPEL process based on an SLA requested by the customer. Our results show that the proposed algorithms drastically reduce the violation rate of the SLA while minimizing the rejection rate of customer SLA requests.
更多查看译文
AI 理解论文
溯源树
样例
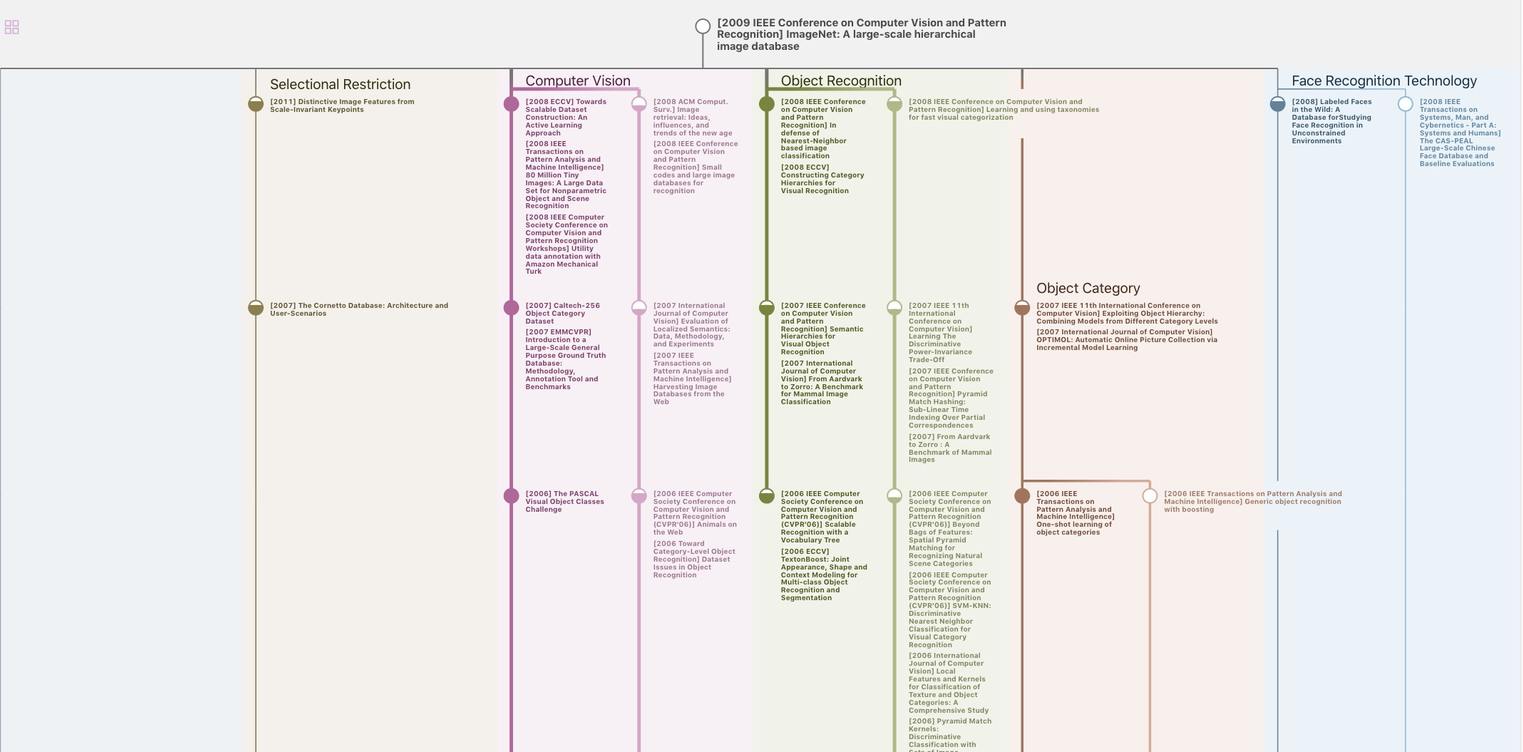
生成溯源树,研究论文发展脉络
Chat Paper
正在生成论文摘要