Robust testing of low-dimensional functions.
ArXiv(2021)
摘要
A natural problem in high-dimensional inference is to decide if a classifier f : R-n -> {-1, 1} depends on a small number of linear directions of its input data. Call a function g : R-n -> {-1, 1}, a linear k-junta if it is completely determined by some k-dimensional subspace of the input space. A recent work of the authors showed that linear k-juntas are testable. Thus there exists an algorithm to distinguish between: (1) f : R-n -> {-1, 1} which is a linear k-junta with surface area s. (2) f is epsilon-far from any linear k-junta with surface area (1 + epsilon)s. The query complexity of the algorithm is independent of the ambient dimension n. Following the surge of interest in noise-tolerant property testing, in this paper we prove a noise-tolerant (or robust) version of this result. Namely, we give an algorithm which given any c > 0, epsilon > 0, distinguishes between: (1) f : R-n -> {-1, 1} has correlation at least c with some linear k-junta with surface area s. (2) f has correlation at most c - epsilon with any linear k-junta with surface area at most s. The query complexity of our tester is k(poly(s/epsilon)). Using our techniques, we also obtain a fully noise tolerant tester with the same query complexity for any class C of linear k-juntas with surface area bounded by s. As a consequence, we obtain a fully noise tolerant tester with query complexity k(O(poly(log k/epsilon))) for the class of intersection of k-halfspaces (for constant k) over the Gaussian space. Our query complexity is independent of the ambient dimension n. Previously, no non-trivial noise tolerant testers were known even for a single halfspace.
更多查看译文
关键词
Property testing,Junta testing,Gaussian analysis
AI 理解论文
溯源树
样例
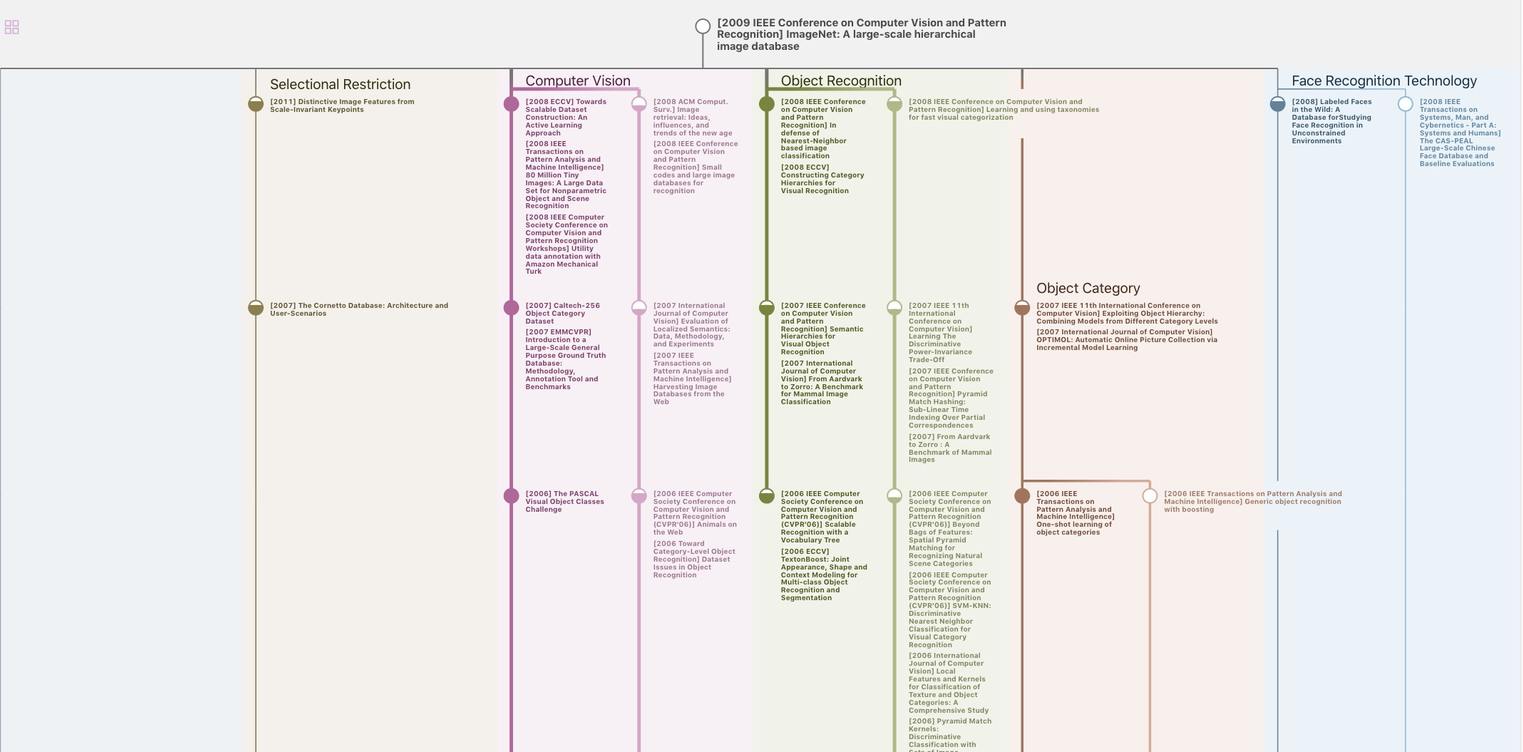
生成溯源树,研究论文发展脉络
Chat Paper
正在生成论文摘要