Multi-step Deep Autoregressive Forecasting with Latent States
semanticscholar(2019)
摘要
In many forecasting applications, it is important to predict an overall trajectory within a period of time, i.e. a forecasting horizon. Various deep neural network models have been introduced to tackle the so-called multi-step forecasting problem. However, most existing approaches either recursively apply a powerful but complex singlestep model or explicitly presume that a relatively simple yet restrictive latent process determines the temporal structure. In this paper, we propose a new framework that combines the advantages of both categories and avoids their weaknesses. We maintain a complex latent process in which each state is implicitly connected through a deep temporal convolutional network with gated recurrent structures between layers in substitution of vanilla recurrent networks. Further, multi-step predictions are made simultaneously by taking advantage of known features such as datetime. We evaluate our model on a number of public benchmark datasets with forecasting horizons of varying length to demonstrate not only its outstanding performance in terms of accuracy but also faster evaluation with its fully-parallelizable architecture.
更多查看译文
AI 理解论文
溯源树
样例
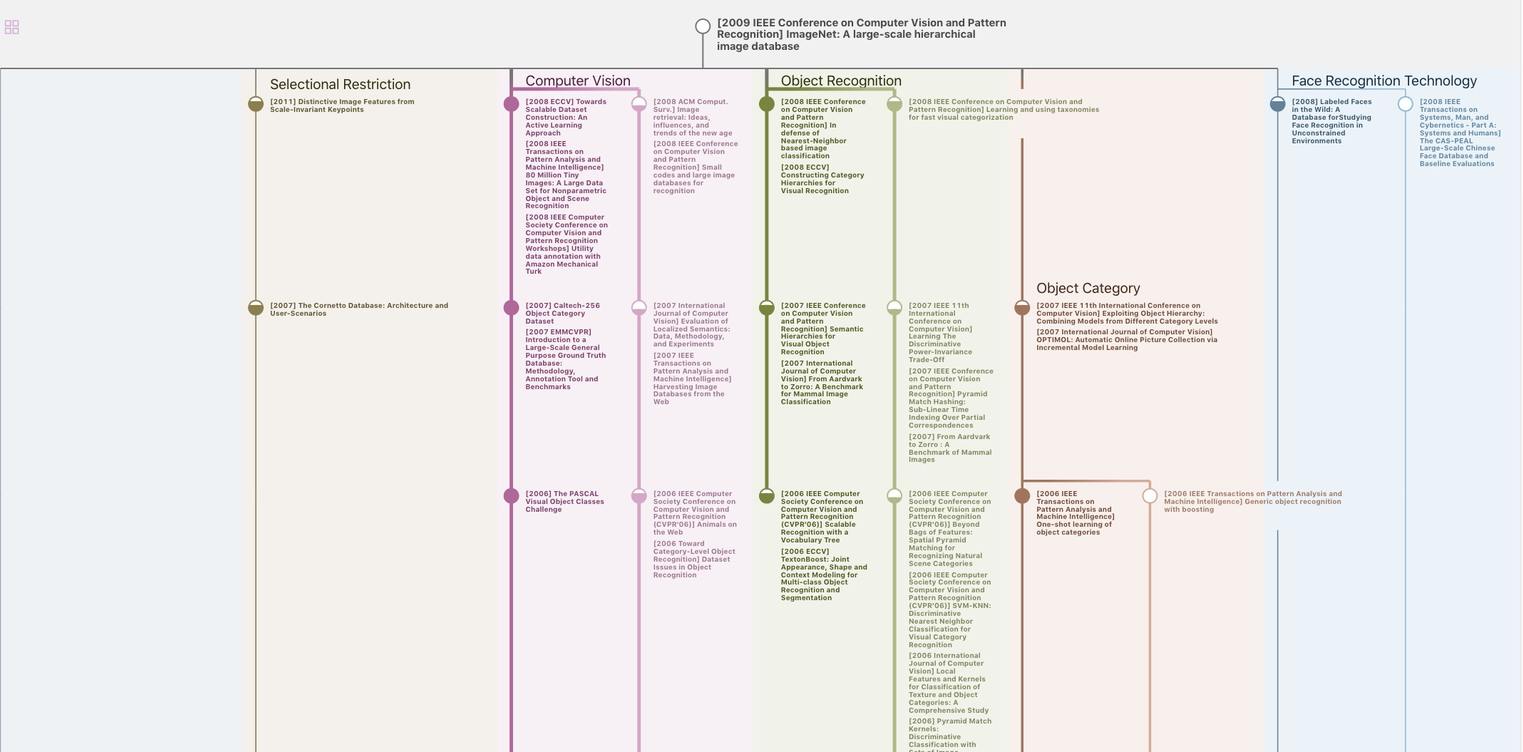
生成溯源树,研究论文发展脉络
Chat Paper
正在生成论文摘要