Input-Convex Neural Networks and Posynomial Optimization
semanticscholar(2016)
摘要
Fitting a model to data is a general problem that arises in many fields. Recently, deep neural networks have been successfully applied to modeling natural images, speech, and language. Posynomial models are another class of models that have been widely applied to problems in engineering design due to their tractability as an optimization problem. Both of these approaches are parametric models for function fitting, though they make different assumptions about the structure of the function being modeled. Neural networks are well-known to be universal function approximators, but this expressiveness comes at the cost of being highly non-convex in their parameters, making them difficult to optimize. By contrast, optimizing posynomial functions can be re-formulated as a convex optimization problem, but these models impose many restrictions on the structure of the learned function. In this paper, we explore connections between deep neural networks and posynomials. First, we explore a network model that trades off expressiveness for more principled optimization. We investigate a novel application of such a network and then we formulate such a model as a posynomial. Finally, we consider the problem of fitting a posynomial function with a neural network and demonstrate fast and accurate posynomial fitting using deep learning machinery.
更多查看译文
AI 理解论文
溯源树
样例
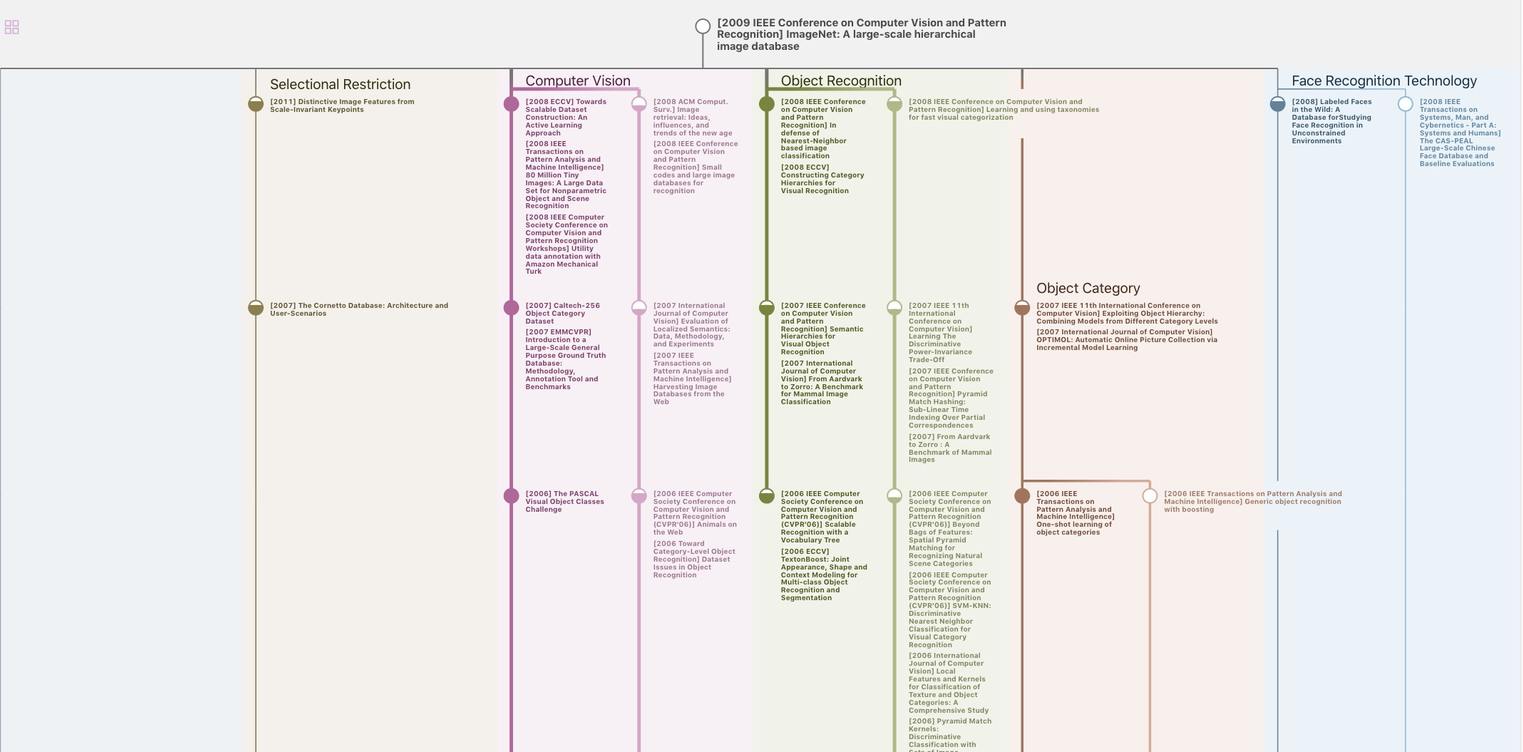
生成溯源树,研究论文发展脉络
Chat Paper
正在生成论文摘要