Contrast Pattern Mining in Paired Multivariate Time Series of a Controlled Driving Behavior Experiment
ACM Transactions on Spatial Algorithms and Systems(2020)
摘要
AbstractThe controlled experiment is an important scientific method for researchers seeking to determine the influence of the intervention, by interpreting the contrast patterns between the temporal observations from control and experimental groups (i.e., paired multivariate time series (PMTS)). Due to recent technological advances and the growing popularity of sensing technology such as in-vehicle sensors and activity trackers, time series data is experiencing explosive growth in both size and complexity. This is threatening to overwhelm the interpretation of control experiments, which conventionally rely on human analysts. Thus, it is imperative to develop automated methods that are expected to simultaneously characterize and detect the interpretable contrast patterns in PMTS generated by controlled experiments. However, a few challenges prohibit existing methods from directly addressing this problem: (1) handling the coupling of contrast identification and pattern characterization, (2) dynamically characterizing the patterns in PMTS, and (3) mining the contrast patterns in multiple PMTS with ubiquitous individual differences. Therefore, we propose a novel framework to mine interpretable contrast patterns based on the dynamic feature dependencies for PMTS through optimization. The proposed framework simultaneously characterizes the dynamic feature dependency networks for PMTS and detects the contrast patterns. Specifically, we characterize the generative process of PMTS as a probabilistic model defined by pairwise Markov random fields whose likelihoods are maximized using our group graphical lasso. The model is then generalized to handle multiple PMTS and solved by proposing a customized algorithm based on the expectation-maximization framework. Extensive experiments demonstrate the effectiveness, scalability, and interpretability of our approach.
更多查看译文
关键词
Contrast pattern, dynamic feature dependency, controlled experiment, driving behavior, multivariate time series
AI 理解论文
溯源树
样例
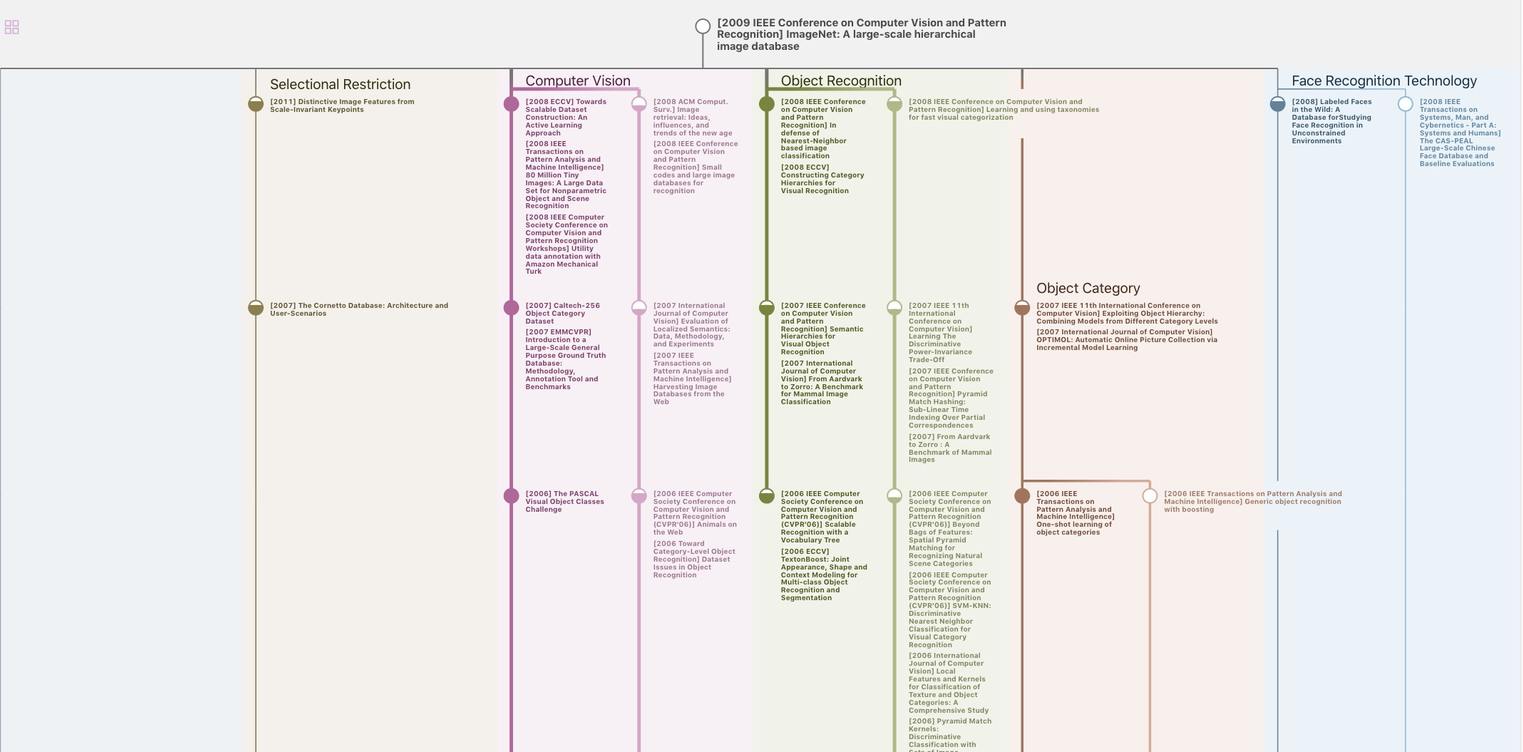
生成溯源树,研究论文发展脉络
Chat Paper
正在生成论文摘要