Optimal Communication-Distortion Tradeoff in Voting
EC '20: The 21st ACM Conference on Economics and Computation Virtual Event Hungary July, 2020(2020)
摘要
In recent work, Mandal et al. [2019] study a novel framework for the winner selection problem in voting, in which a voting rule is seen as a combination of an elicitation rule and an aggregation rule. The elicitation rule asks voters to respond to a query based on their preferences over a set of alternatives, and the aggregation rule aggregates voter responses to return a winning alternative. They study the tradeoff between the communication complexity of a voting rule, which measures the number of bits of information each voter must send in response to its query, and its distortion, which measures the quality of the winning alternative in terms of utilitarian social welfare. They prove upper and lower bounds on the communication complexity required to achieve a desired level of distortion, but their bounds are not tight. Importantly, they also leave open the question whether the best randomized rule can significantly outperform the best deterministic rule.
We settle this question in the affirmative. For a winner selection rule to achieve distortion d with m alternatives, we show that the communication complexity required is ~Θ (m/d) when using deterministic elicitation, and ~Θ (m/d3) when using randomized elicitation; both bounds are tight up to logarithmic factors. Our upper bound leverages recent advances in streaming algorithms. To establish our lower bound, we derive a new lower bound on a multi-party communication complexity problem.
We then study the k-selection problem in voting, where the goal is to select a set of k alternatives. For a k-selection rule that achieves distortion d with m alternatives, we show that the best communication complexity is ~Θ (m/(kd)) when the rule uses deterministic elicitation and ~Θ (m/(kd3)) when the rule uses randomized elicitation. Our optimal bounds yield the non-trivial implication that the k-selection problem becomes strictly easier as k increases.
更多查看译文
AI 理解论文
溯源树
样例
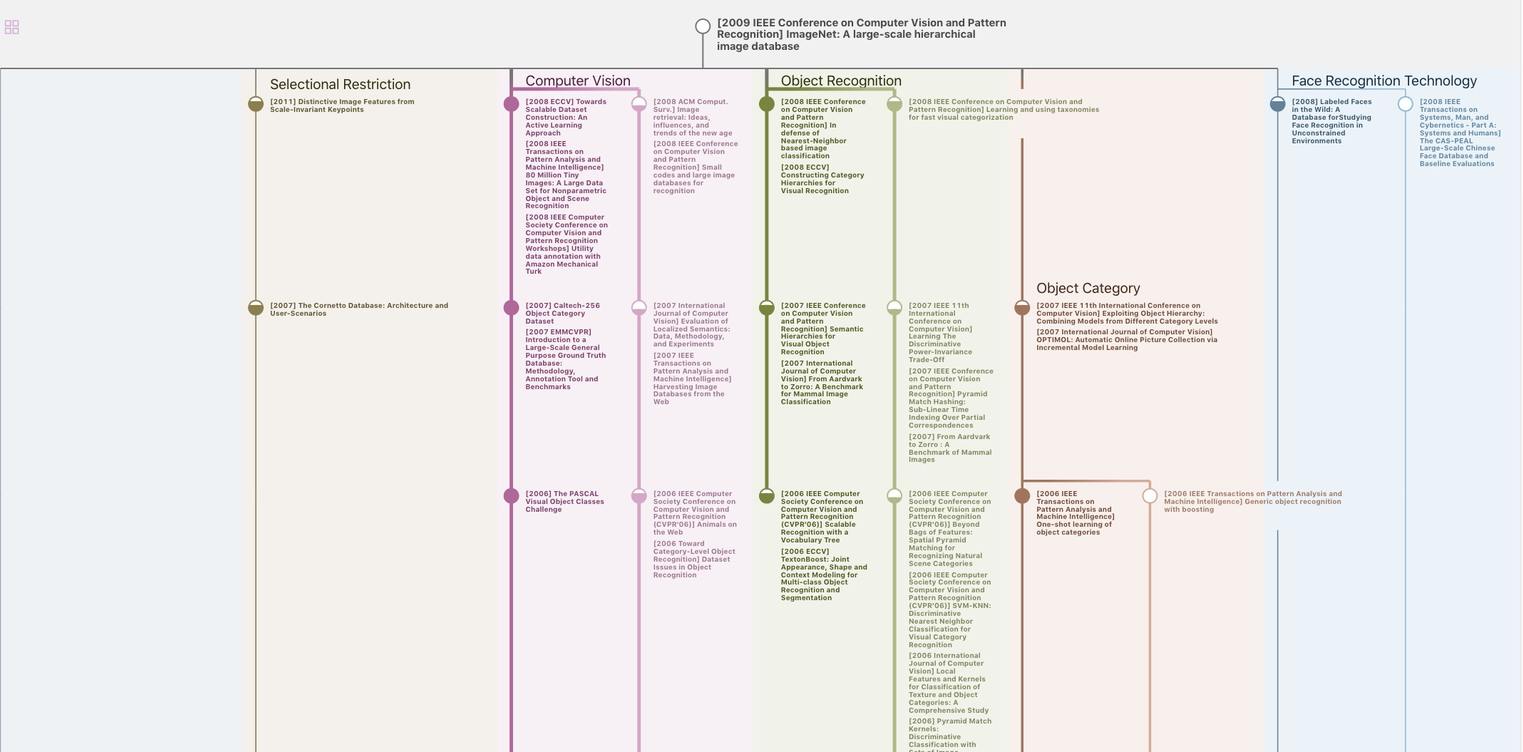
生成溯源树,研究论文发展脉络
Chat Paper
正在生成论文摘要