PaletteStarViz: a visualization method for multi-criteria decision making from high-dimensional pareto-optimal front
GECCO '20: Genetic and Evolutionary Computation Conference Cancún Mexico July, 2020(2020)
摘要
Visual representation of a many-objective Pareto-optimal front in four or more dimensional objective space requires a large number of data points. Moreover, choosing a single point from a large set even with certain preference information is problematic, as it causes a large cognitive burden on the part of the decision-makers. Therefore, many-objective optimization and decision-making practitioners have been interested in effective visualization methods to enable them to filter down a large set to a few critical points for further analysis. Most existing visualization methods are borrowed from other data analytic domains and they are too generic to be effective for many-criteria decision making. In this paper, we propose an alternative visualization method, following an earlier concept, using star-coordinate plots for effectively visualizing many-objective trade-off solutions. The proposed PaletteStarViz respects some basic topological, geometric, and functional decision-making properties of high-dimensional trade-off points mapped to a "two-and-a-half" dimensional space. We demonstrate the use of PaletteStarViz to a number high-dimensional Pareto-optimal fronts.
更多查看译文
AI 理解论文
溯源树
样例
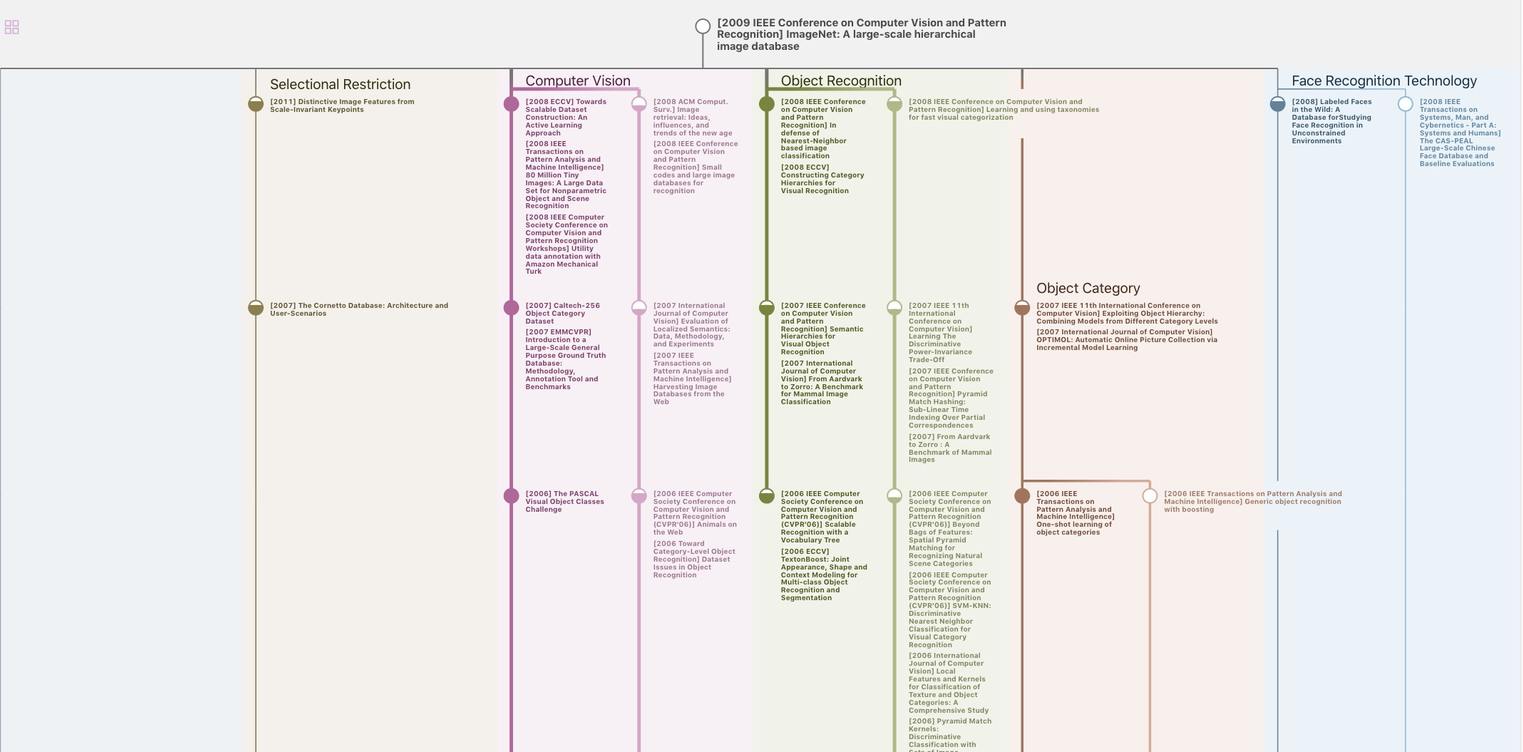
生成溯源树,研究论文发展脉络
Chat Paper
正在生成论文摘要