CoFi-points: Collaborative Filtering via Pointwise Preference Learning on User/Item-Set
ACM Transactions on Intelligent Systems and Technology(2020)
摘要
AbstractWith the explosive growth of web resources, an increasingly important task in recommender systems is to provide high-quality personalized services by learning users’ preferences from historically observed information. As an effective preference learning technology, collaborative filtering has been widely extended to model the one-class or implicit feedback data, which is known as one-class collaborative filtering (OCCF). For a long time, pairwise ranking-oriented learning scheme has been viewed as a superior solution than the pointwise scheme for OCCF due to its higher accuracy in most cases. However, we argue that with appropriate model design, pointwise preference learning can achieve comparable or even better performance than the counterpart, i.e., pairwise preference learning. In particular, we propose a new preference assumption, i.e., pointwise preference on user/item-set. Based on this new assumption, we develop a novel, simple, and flexible solution called collaborative filtering via pointwise preference learning on user/item-set (CoFi-points). Furthermore, we derive two specific algorithms of CoFi-points with respect to the involved user-set and item-set, i.e., CoFi-points(u) and CoFi-points(i), referring to preference assumptions defined on user-set and item-set, respectively. Finally, we conduct extensive empirical studies on four real-world datasets with the state-of-the-art methods, and find that our solution can achieve very promising performance with respect to several ranking-oriented evaluation metrics.
更多查看译文
关键词
One-class collaborative filtering, implicit feedback, pointwise preference learning, user-set, item-set
AI 理解论文
溯源树
样例
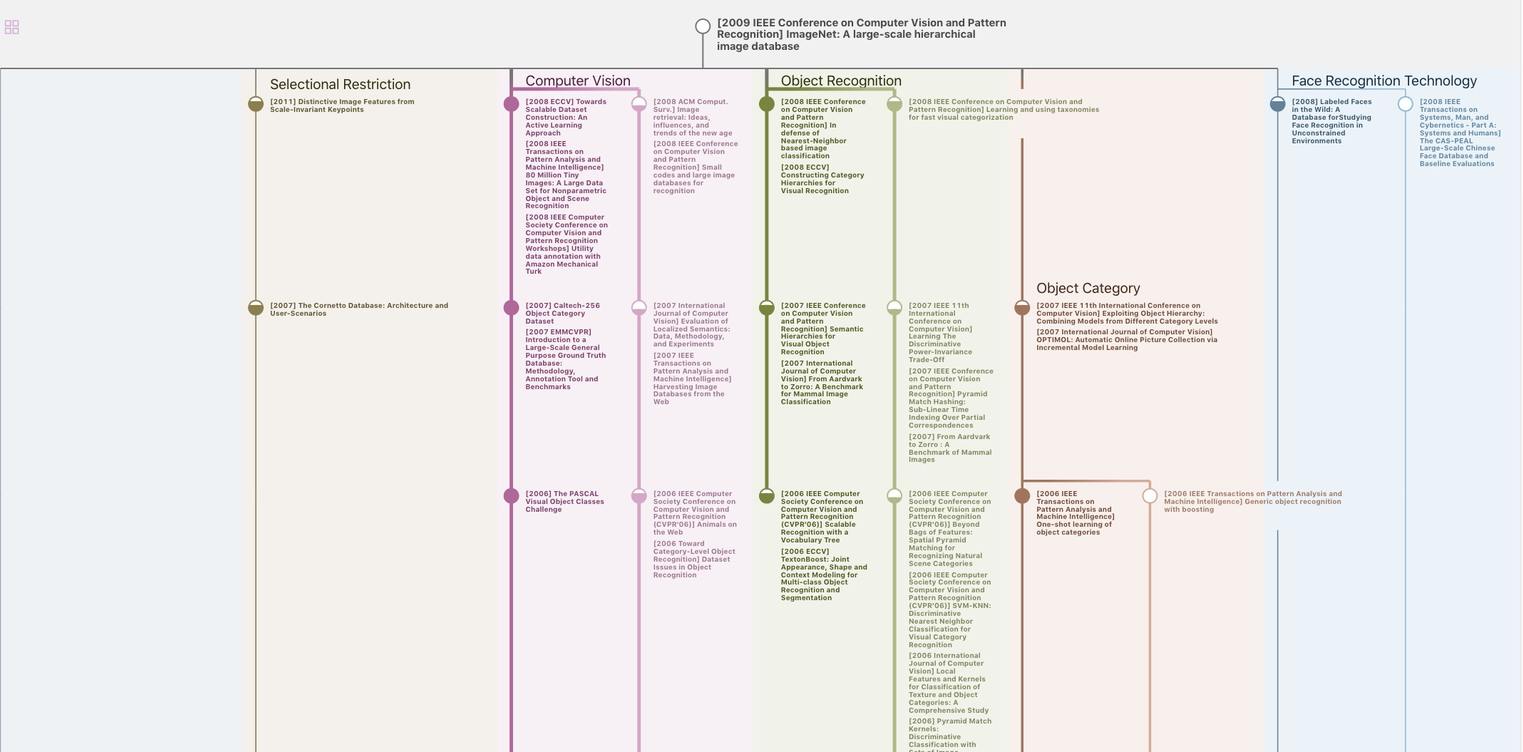
生成溯源树,研究论文发展脉络
Chat Paper
正在生成论文摘要