Representing Temporal Attributes for Schema Matching
KDD '20: The 26th ACM SIGKDD Conference on Knowledge Discovery and Data Mining Virtual Event CA USA July, 2020(2020)
摘要
Temporal data are prevalent, where one or several time attributes present. It is challenging to identify the temporal attributes from heterogeneous sources. The reason is that the same attribute could contain distinct values in different time spans, whereas different attributes may have highly similar timestamps and alike values. Existing studies on schema matching seldom explore the temporal information for matching attributes. In this paper, we argue to order the values in an attribute A by some time attribute T as a time series. To learn deep temporal features in the attribute pair (T, A), we devise an auto-encoder to embed the transitions of values in the time series into a vector. The temporal attribute matching (TAM) is thus to evaluate matching distance of two temporal attribute pairs by comparing their transition vectors. We show that computing the optimal matching distance is NP-hard, and present an approximation algorithm. Experiments on real datasets demonstrate the superiority of our proposal in matching temporal attributes compared to the generic schema matching approaches.
更多查看译文
关键词
Data Integration, Schema Matching, Temporal Data
AI 理解论文
溯源树
样例
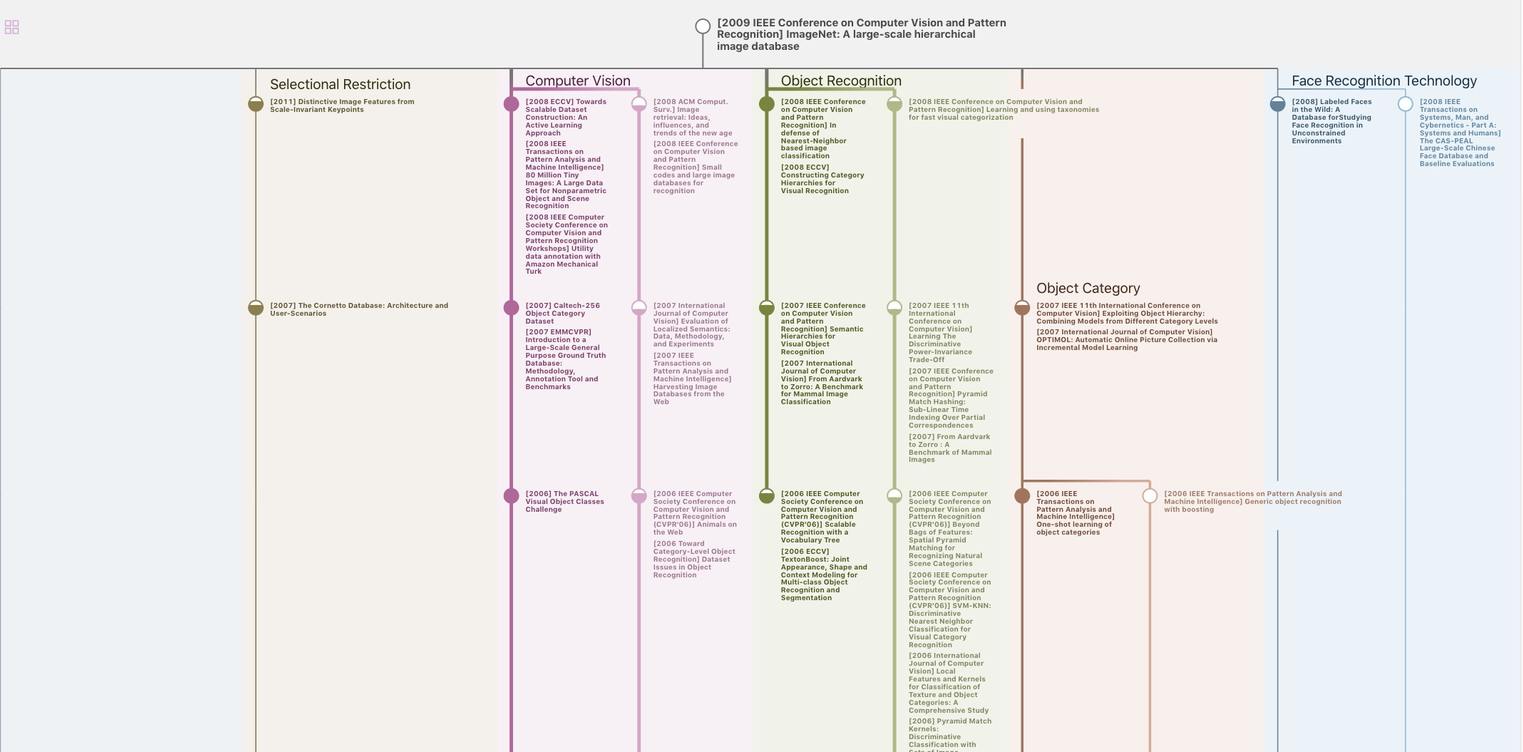
生成溯源树,研究论文发展脉络
Chat Paper
正在生成论文摘要