Neuromorphic Computing with Phase Change, Device Reliability, and Variability Challenges
2020 IEEE International Reliability Physics Symposium (IRPS)(2020)
摘要
Neuromorphic computing with analog memory can accelerate deep neural networks (DNNs) by enabling multiply-accumulate (MAC) operations to occur within memory. Analog memory, however, presents a number of device-level challenges having macro-implications on the achievable accuracy and reliability of these artificial neural networks. This paper focuses on the adverse effects of conductance drift in phase-change memory (PCM) on network reliability. It is shown that conductance drift can be effectively compensated in a variety of networks by applying a ‘slope correction’ technique to the squashing functions to maintain accuracy/reliability for a period of ~1 year. In addition to conductance drift, PCM poses considerable variability challenges, which impact the accuracy of the initial t
0
weights. This paper summarizes recent advances in optimizing t
0
weight programming, and provides evidence suggesting that the combination of ‘slope correction’ and programming optimization techniques may allow DNN acceleration using analog memory while maintaining software-equivalent accuracy with reasonable reliability.
更多查看译文
关键词
analog DNN accelerator,crossbar array,phase-change memory,reliability,variability
AI 理解论文
溯源树
样例
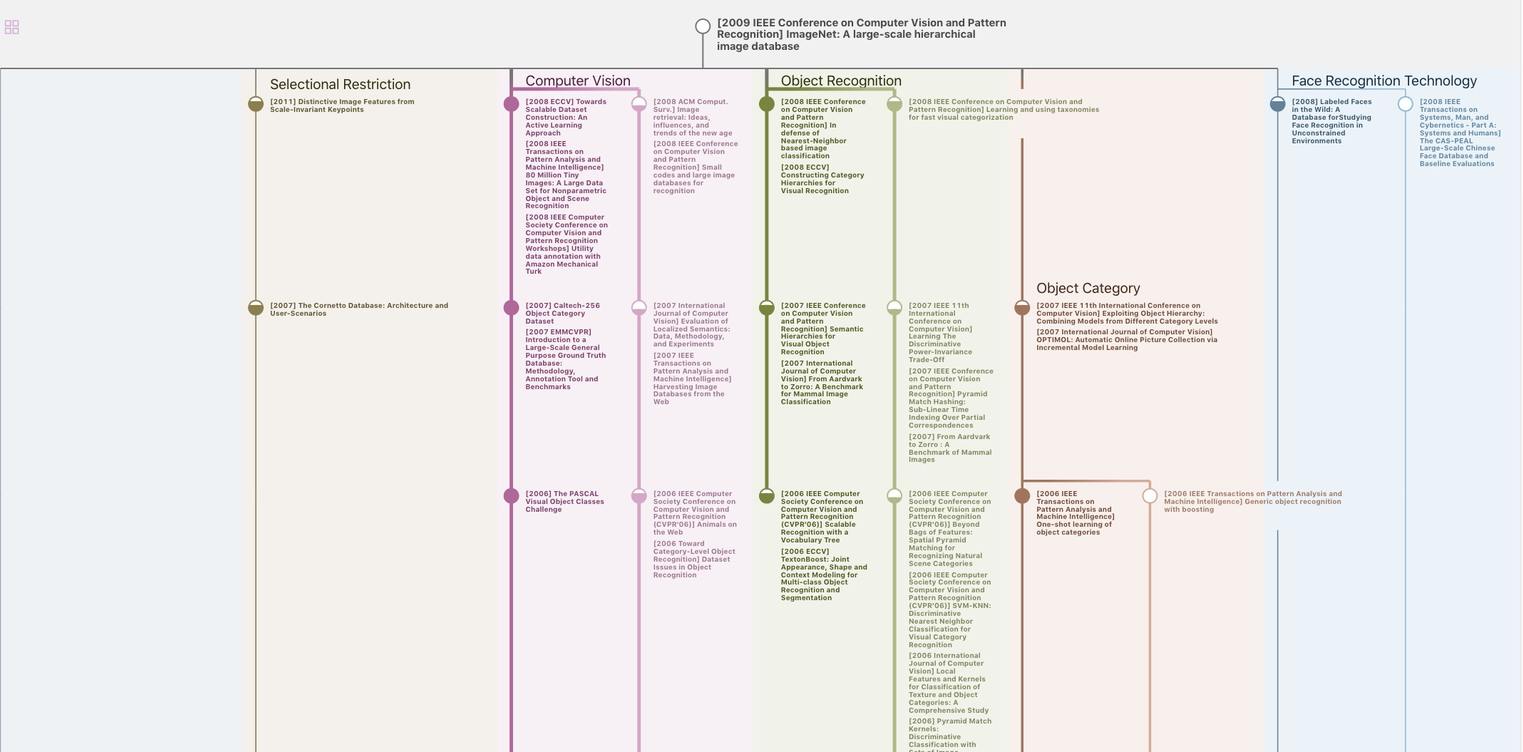
生成溯源树,研究论文发展脉络
Chat Paper
正在生成论文摘要