Feature Ranking under Industrial Constraints in Continuous Monitoring Applications based on Machine Learning Techniques
2020 IEEE International Instrumentation and Measurement Technology Conference (I2MTC)(2020)
摘要
The design work-flow of machine learning techniques for continuous monitoring or predictive maintenance in an industrial context is usually a two step procedure: the selection of features to be computed from the observed signals and training of a suitable algorithm with real-life meaningful data, that will be next deployed in the second step. Feature selection is a relevant task since it provides a powerful optimisation of the deployed algorithm performance, for the given training data-set. The paper provides a method for feature ranking and selection that embeds constraints coming from real-life applications, including sensing device specifications, environmental noise, available processing resources, being all these latter aspects not considered in the currently available literature methods for feature selection. A practical case-study in the field on anomaly detection of machines is reported and discussed, in order to show the good properties of the provided method.
更多查看译文
关键词
Condition Monitoring,Predictive Maintenance,Machine Learning,Feature engineering,Feature selection,Industrial constraint,Constrained optimisation,Data Fusion,IoT,IIoT,Industry 4.0
AI 理解论文
溯源树
样例
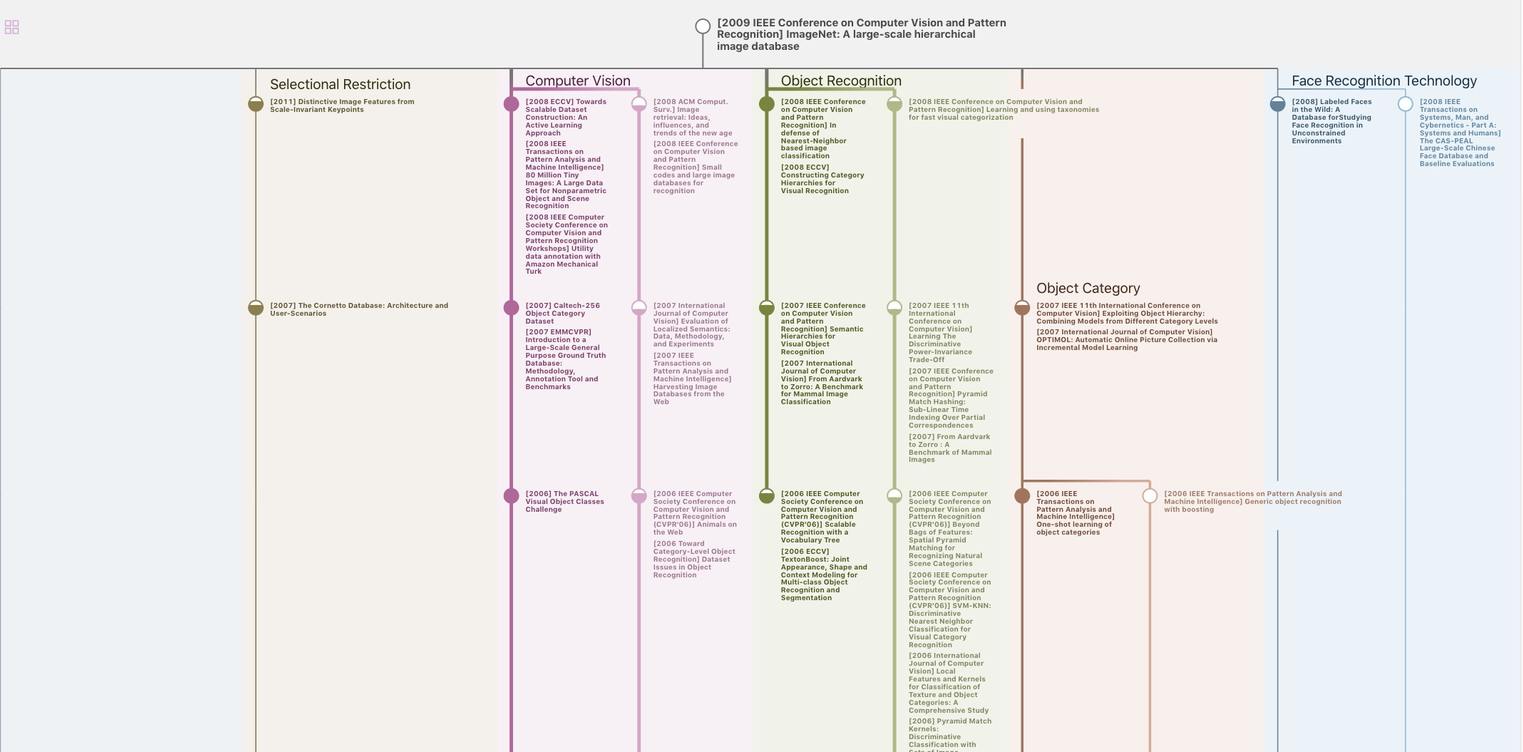
生成溯源树,研究论文发展脉络
Chat Paper
正在生成论文摘要