Adversarial Oracular Seq2seq Learning for Sequential Recommendation
IJCAI 2020(2020)
摘要
Recently, sequential recommendation has become a significant demand for many real-world applications, where the recommended items would be displayed to users one after another and the order of the displays influences the satisfaction of users. An extensive number of models have been developed for sequential recommendation by recommending the next items with the highest scores based on the user histories while few efforts have been made on identifying the transition dependency and behavior continuity in the recommended sequences. In this paper, we introduce the Adversarial Oracular Seq2seq learning for sequential Recommendation (AOS4Rec), which formulates the sequential recommendation as a seq2seq learning problem to portray time-varying interactions in the recommendation, and exploits the oracular learning and adversarial learning to enhance the recommendation quality. We examine the performance of AOS4Rec over RNN-based and Transformer-based recommender systems on two large datasets from real-world applications and make comparisons with state-of-the-art methods. Results indicate the accuracy and efficiency of AOS4Rec, and further analysis verifies that AOS4Rec has both robustness and practicability for real-world scenarios.
更多查看译文
AI 理解论文
溯源树
样例
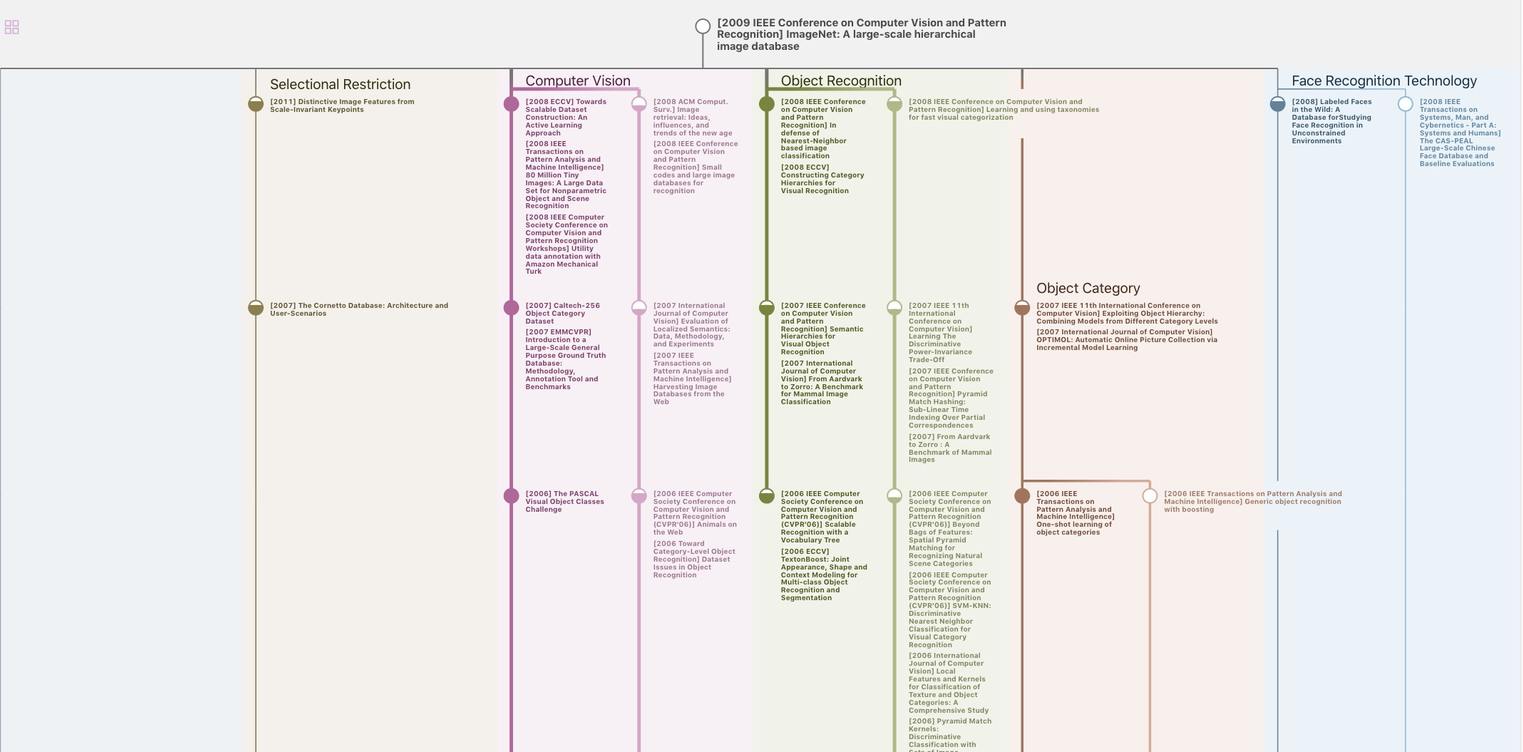
生成溯源树,研究论文发展脉络
Chat Paper
正在生成论文摘要