Emulating a sensor using soft material dynamics: A reservoir computing approach to pneumatic artificial muscle
2020 3rd IEEE International Conference on Soft Robotics (RoboSoft)(2020)
摘要
The McKibben pneumatic artificial muscle (PAM) is a promising soft actuator featuring flexibility, light weight, and high power. However, to realize high-precision control with such soft actuators and achieve human-like, autonomous, and natural behavioral control, both actuation techniques and sensing technologies require development to make the most of their features. In this regard, McKibben PAMs contain a drawback rooted in the nonlinear mechanical properties of their soft materials, as frictional anisotropy triggered upon PAM actuation between its fibers and the tubes causes hysteresis. Therefore, because the length of PAM is not uniquely determined by the applied pressure, it is generally necessary to install a sensor device (such as a laser displacement meter) for measuring the length in addition to the platform. In this study, we propose a scheme that can estimate a PAM's length using applied pressure and its internal dynamics in real time, making use of the machine learning technique called reservoir computing. We show that estimation can be performed with significantly high accuracy. We subsequently demonstrate that the proposed approach is robust against drastic and immediate changes in platform conditions, and can be adapted dynamically to real-time change. We expect this approach can replace the functionality of the laser displacement meter, freeing the platform from mechanical constraints and leading to the realization of a simpler soft actuator capable of sensing using soft material dynamics.
更多查看译文
关键词
soft material dynamics,McKibben pneumatic artificial muscle,flexibility,high-precision control,soft actuators,McKibben PAMs,nonlinear mechanical properties,internal dynamics,machine learning,frictional anisotropy,reservoir computing,recurrent neural networks
AI 理解论文
溯源树
样例
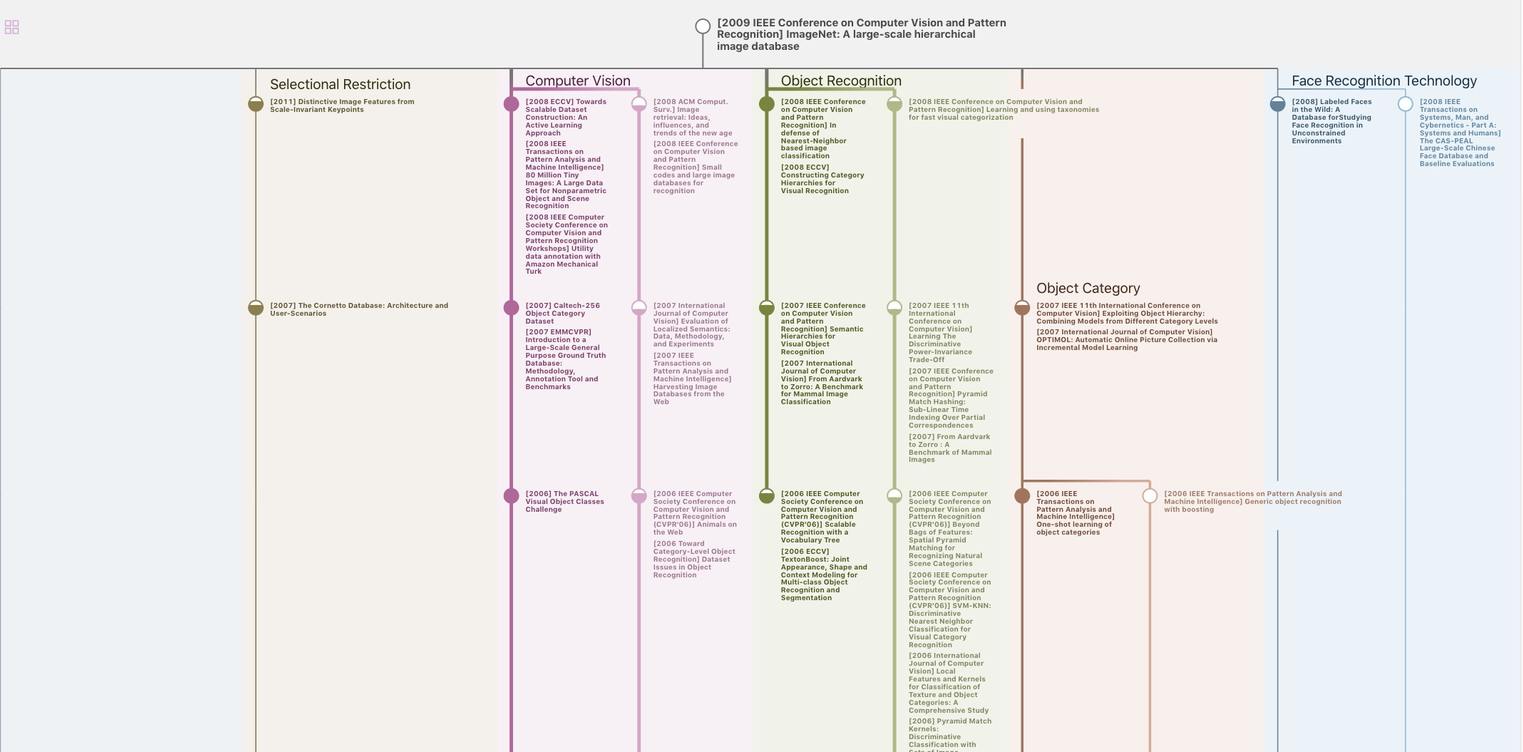
生成溯源树,研究论文发展脉络
Chat Paper
正在生成论文摘要