Logdet divergence based metric learning using triplet labels
Proceedings of the Workshop on Divergences and Divergence Learning (ICML’13)(2013)
摘要
Metric learning is fundamental to lots of learning algorithms and it plays significant roles in many applications. In this paper, we present a LogDet divergence based metric learning approach to a learn Mahalanobis distance over input space of the instances. In the proposed model, the most natural constraint triplets are used as the labels of the training samples. Meanwhile, in order to avoid overfitting problem, the model uses the LogDet divergence to regularize the obtained Mahalanobis matrix as close as possible to a given matrix. Besides, a cyclic iterative algorithm is presented to solve the objective function and accelerate the metric learning process. Furthermore, this paper constructs a novel dynamic triplets building strategy to guarantee that the most useful triplets are used in every training cycle. Experiments on benchmark data sets demonstrates the proposed model achieve an improved performance when compared with the state-of-theart methods.
更多查看译文
AI 理解论文
溯源树
样例
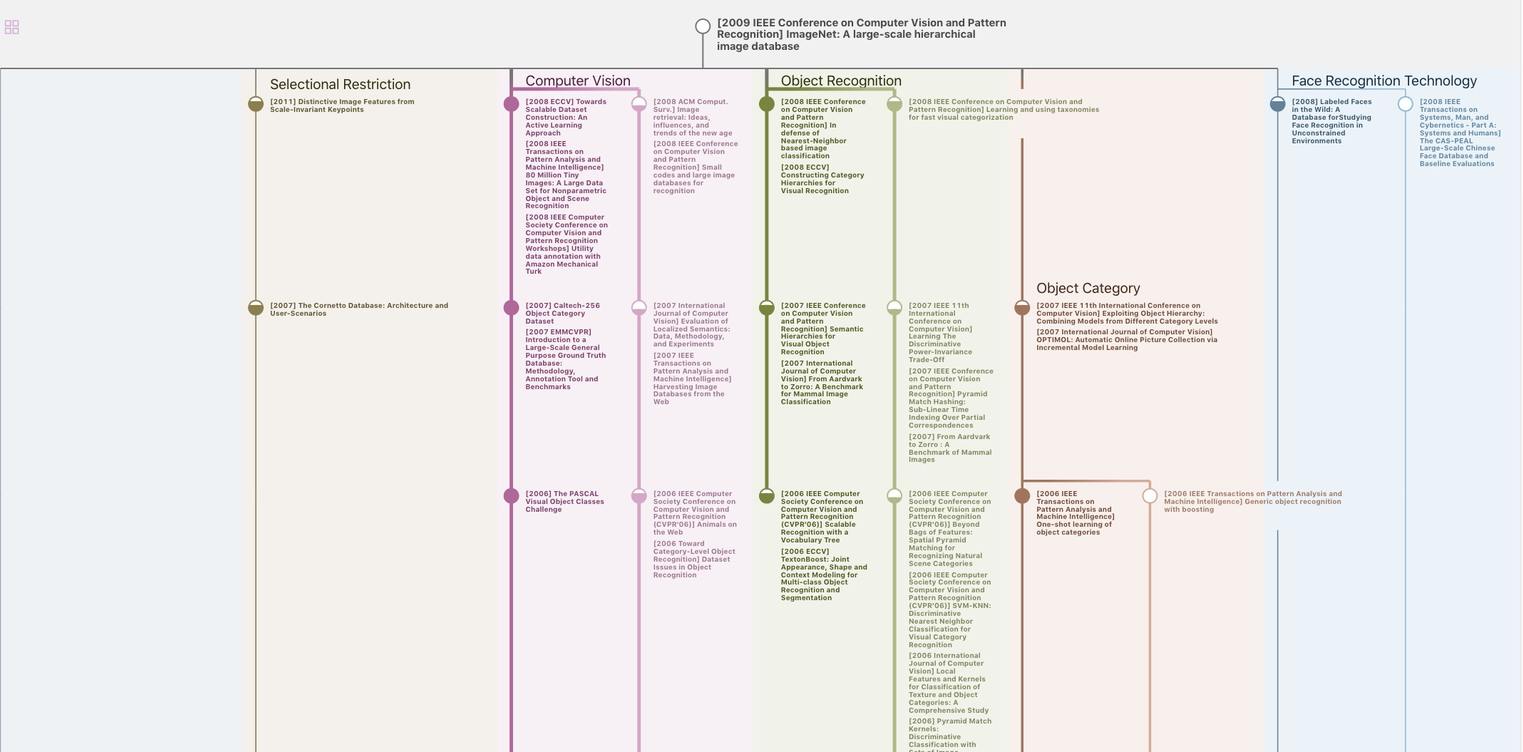
生成溯源树,研究论文发展脉络
Chat Paper
正在生成论文摘要