Searching Towards Class-Aware Generators for Conditional Generative Adversarial Networks
arXiv: Computer Vision and Pattern Recognition(2022)
摘要
Conditional generative adversarial networks (cGANs) are designed to generate images based on the provided conditions, e.g., class-level distributions, semantic label maps, etc. Existing methods have used the same generator architecture for all classes. This paper presents an idea that adopts neural architecture search (NAS) to find a class-aware architecture for each class. The search space contains regular and class-modulated convolutions, where the latter is designed to introduce class-specific information while avoiding the reduction of training data for each class generator. The search algorithm follows a weight-sharing pipeline with mixed-architecture optimization so that the search cost does not grow with the number of classes. To learn the sampling policy, a Markov decision process is embedded into the search algorithm, and a moving average is applied for better stability. Class-aware generators show advantages over class-agnostic architectures experimentally. Moreover, we discover two intriguing phenomena that are inspirational to craft cGANs by hand.
更多查看译文
关键词
Generators,Computer architecture,Training,Microprocessors,Training data,Optimization,Convolution,Conditional generative adversarial networks,neural architecture search,class-aware
AI 理解论文
溯源树
样例
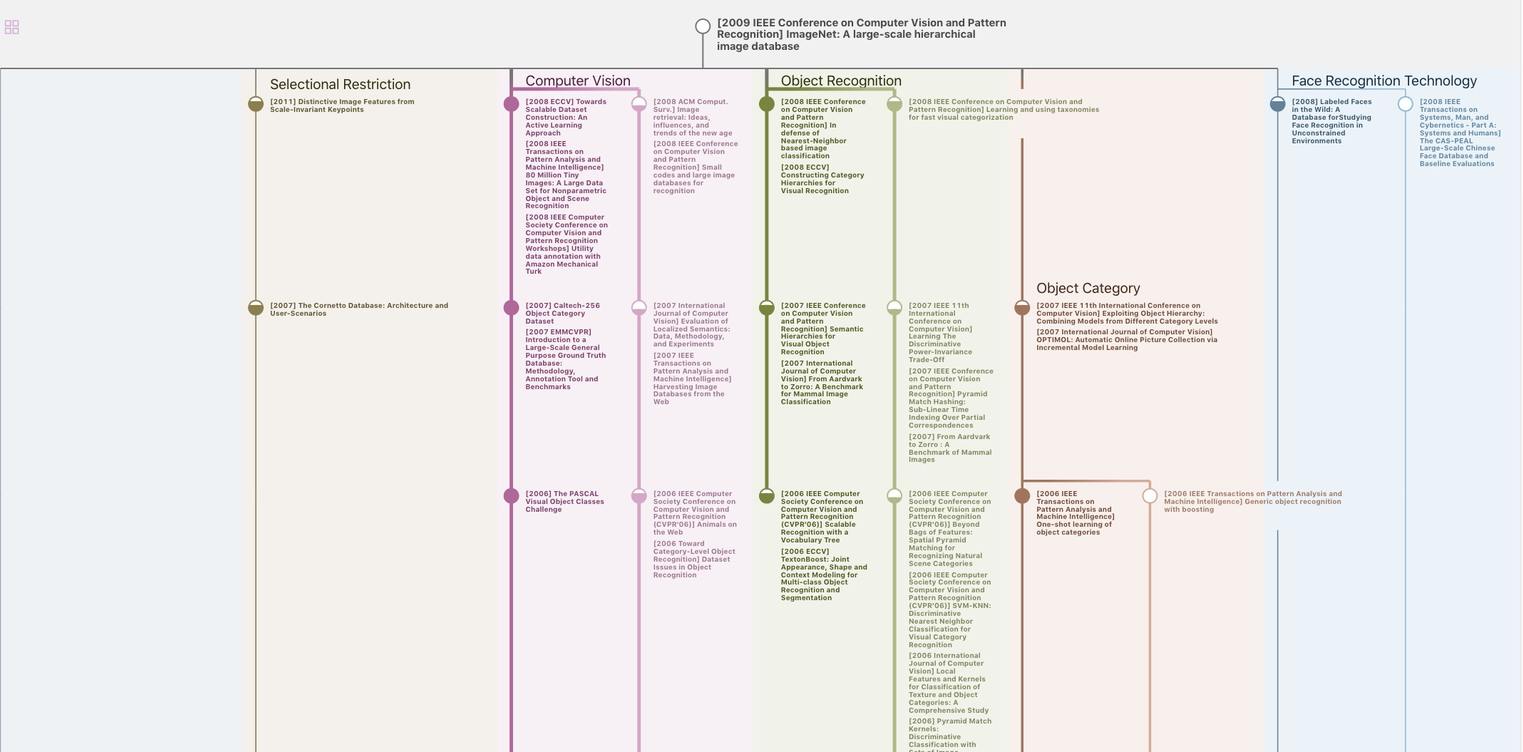
生成溯源树,研究论文发展脉络
Chat Paper
正在生成论文摘要